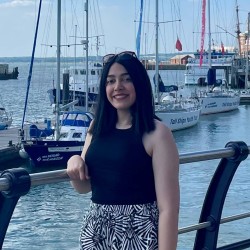
Yasaman Mohammadi
Academic and research departments
Surrey Institute for People-Centred Artificial Intelligence (PAI), Global Centre for Clean Air Research, Centre for Vision, Speech and Signal Processing (CVSSP).About
My research project
AI in Air PollutionIntegrating AI and Machine Learning for State-of-the-Art Air Pollution Modeling; With a Focus on a Physically-Informed Approach to Air Quality Assessment
Supervisors
Integrating AI and Machine Learning for State-of-the-Art Air Pollution Modeling; With a Focus on a Physically-Informed Approach to Air Quality Assessment
Publications
The aim of this paper is the spatiotemporal characterization of daily PM10 (1 km spatial resolution) using machine learning (ML) models (Random Forest (RF) and Gaussian Process Regression (GPR)) over Tehran as the most polluted city in Iran for policy-makers. The performances of the ML models (which are calibrated via large-scale and different spatially interpolated in situe information) were compared against a benchmark station-based interpolator named Inverse Distance Weighting (IDW) using various statistical metrics. Using hold-out training approach and 70% of the available data, the Kling-Gupta Efficiency (KGE) values of the validation (training) sites were achived 0.60(0.56), 0.61(0.72), and 0.68(0.6) for GPR, RF, and IDW, respectively. Based on the seasonal assessmentof the validation gauges, all models performed similarly well in spring and summer; however, the IDW and ML models had better accuracy in winter and autumn, respectively. Furthermore, the results of Correlated Triple Collocation (CTC) implied that ML-based techniques provided a more accurate spatial distribution over the computational grids. Based on the evaluation of the representated daily mean maps, the IDW produced “Bull's eyes” around the monitoring stations. Although IDW yielded reasonable site-based performance, the IDW method may not deliver a realistic estimation of the pollutant over the study region.