Next-generation AI algorithms using self-supervised learning
Artificial intelligence (AI) is the biggest tool humanity has in the 21st century which is set to transform our lives by expanding our understanding of the smallest particles and the infinite possibilities of the universe, guarding us from harm by predicting dangerous situation, personalising products and services to improve quality of life, delivering precise healthcare, better understanding the 3D structure of human DNA, improving healthcare by drug discovery and testing, generating new possibilities for creation and entertainment, creating smart and safe cities. The research will build the next generation of AI algorithms which will be applicable across a range of applications, hence, will have a very high impact. If you have what it takes to transform lives, lead the next revolution in AI this opportunity is for you.
Start date
1 October 2022Duration
3 yearsApplication deadline
Funding source
Surrey Institute for People-Centred AI (SI-PAI) and School of CSEEFunding information
- Stipend of £16,062 for 2022-23, which will increase each year in line with the UK Research and Innovation (UKRI) rate
- Home rate fee allowance of £4,500 (with automatic increase to UKRI rate each year).
For exceptional international candidates, there is the possibility of obtaining a scholarship to cover overseas fees.
About
Much of the success of the AI is attributed to supervised-pretraining (SP) of deep neural networks and then adopting them for downstream applications specific tasks. However, there are several challenges and issues with labelled data, e.g, labelling cost, noisy-labels, incomplete and inadequate labels (focusing on only dominant concept/concepts in images), inherent human labelling bias. An alternative to supervised-learning is self/unsupervised-learning which learns without labels. Two of the key principles of self-supervised-learning in (computer) vision-based AI systems are a) get augmented views of the same input and enforce some notion of consistency between the views, b) mask part of the input and recover that part from the rest of the unmasked input.
Although SSL is predicted to be the future of AI research, as put by AI pioneers, “The revolution will not be supervised". However, until very recently self-supervised-pretraining fell behind supervised-pretraining for computer vision applications hindering the realisation of SSL dream. This was changed by Group Masked Model Learning (GMML) which was proposed in our seminal work SiT: Self-supervised vIsion Transformers in 2021. The GMML marked a milestone in AI development as it is the first method to outperform supervised-pretraining and learned semantic concepts without using any labels. The core idea of GMML is adopted by tech giants like Microsoft, Facebook and several others for application areas including, computer vision, audio, medical-image analysis, anomaly detection, multimodal analysis and many more.
The research will build upon the world's most advanced self-supervised-learning algorithms created at CVSSP and create the future generation of AI algorithms which will rely on very little labelled data to learn and will be applicable across a range of applications, hence, will have a very high impact. If you have what it takes to transform lives, lead the next revolution in AI this opportunity is for you.
Related links
Centre for Vision, Speech and Signal Processing (CVSSP) Surrey Institute for People-Centred Artificial IntelligenceReferences
- Sara Atito, Muhammad Awais, Ammarah Farooq, Zhenhua Feng, Josef Kittler, “MC-SSL0.0: Towards Multi-Concept Self-Supervised Learning”, 2021 (https://arxiv.org/abs/2111.15340).
- Sara Atito, Muhammad Awais, Josef Kittler, “SiT: Self-supervised vIsion Transformer” (https://arxiv.org/abs/2104.03602).
- Aston Zhang, Zack C. Lipton, Mu Li, Alex J. Smola, “Dive into Deep Learning” (https://d2l.ai).
- Ian Goodfellow and Yoshua Bengio and Aaron Courville, Deep Learning, MIT Press, 2016 (https://www.deeplearningbook.org).
- Michael M. Bronstein, Joan Bruna, Taco Cohen, Petar Veličković, Geometric Deep Learning Grids, Groups, Graphs, Geodesics, and Gauges, 2021 (https://arxiv.org/abs/2104.13478).
Eligibility criteria
All applicants should have (or expect to obtain) a first-class degree in a numerate discipline (mathematics, science or engineering) or MSc with Distinction (or 70% average) and a strong interest in pursuing research in this field. Additional experience which is relevant to the area of research is also advantageous.
English language requirements
IELTS Academic 6.5 or above (or equivalent) with 6.0 in each individual category.
How to apply
Applications should be submitted via the Centre for Vision, Speech and Signal Processing programme page. Please clearly state the studentship title and supervisor on your application.
Studentship FAQs
Read our studentship FAQs to find out more about applying and funding.
Application deadline
Contact details
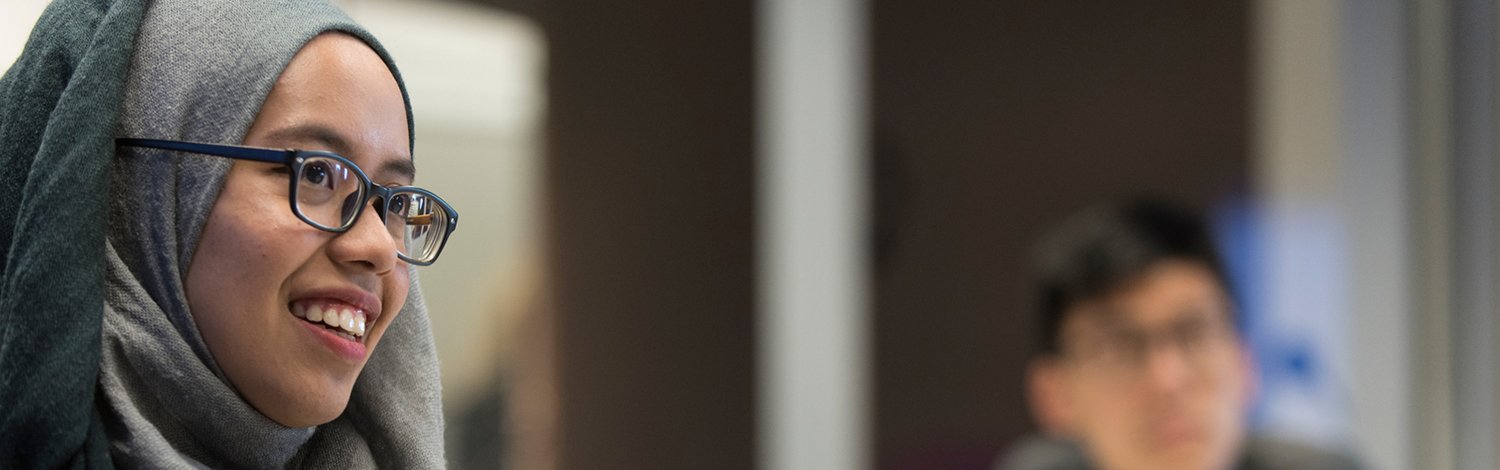
Studentships at Surrey
We have a wide range of studentship opportunities available.