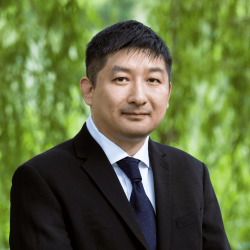
Professor Jin Xuan
Academic and research departments
Faculty of Engineering and Physical Sciences, School of Chemistry and Chemical Engineering.About
Biography
Professor Jin Xuan joined the University of Surrey as the Associate Dean of Research and Innovation for the Faculty of Engineering and Physical Sciences in September 2022. He holds a Chair in Sustainable Processes and a prestigious EPSRC Open Fellowship at the School of Chemistry and Chemical Engineering. He is also a Turing Fellow at The Alan Turing Institute. Before moving to Surrey, he was the Head of the Department of Chemical Engineering at Loughborough University.
Professor Xuan has won a number of prizes and awards in recognition of his research excellence. He is the recipient of the Philip Leverhulme Prize of Engineering in 2022 for his pioneering research on Energy and AI, and the Beilby Medal and Prize, jointly from the Society of Chemical Industry (SCI), the Royal Society of Chemistry (RSC), and the Institute of Materials, Minerals and Mining (IOM3) in 2020 for his work that ‘has exceptional practical significance in chemical engineering, applied materials science, energy efficiency or a related field’. He also led the UKRI CircularChem Centre winning the IChemE Global Award for Sustainability in 2023 and the RSC Environment, Sustainability and Energy Horizon Prize: John Jeyes Prize in 2025.
Professor Xuan actively takes leadership in the wider research community. He is an EPSRC Strategic Advisory Team (SAT) member for Energy and Decarbonisation, and a member of the Research, Innovation & Knowledge Transfer Committee of the Engineering Professors’ Council.
Prof Xuan contributed to the establishment of the emerging Energy and AI research community globally. He is the Founding Editor-in-Chief of Digital Chemical Engineering (IChemE), the Founding Editor of Energy and AI (Elsevier), and the co-chair of the International Conference of Energy and AI series (Tianjin 2020, London 2021, Belfort 2022, Xining 2023, Ningbo 2024, Reykjavik 2025). In his Editorial of Energy and AI, he provided a strategic overview in this interdisciplinary research area including wider societal issues such as ethics, morality, policy and law.
Professor Xuan is an advocate for Responsible Assessment. He was interviewed by Nature as part of a news item China bans cash rewards for publishing papers, where he provided his expert view on policy development for proper use of journal metrics in research evaluation. He also actively promotes EDI in the wider research community. He serves as a member of the EPSRC's EDI Strategic Advisory Group and the EDI Champion in the EPSRC Energy SAT. He is the leading author of The equality, diversity and inclusion in energy and AI: Call for actions.
University roles and responsibilities
- Associate Dean (Research and Innovation) for the Faculty of Engineering and Physical Sciences
- Professor of Sustainable Processes
- Member of Council
- Member of Senate
- Member of the Faculty EDI Committee
News
ResearchResearch interests
Professor Xuan’s research focuses on net zero energy, circular economy and sustainable development via digital and engineering innovations. He is leading the development of bespoke AI and digital solutions to enable next generation energy and chemical materials, devices, processes and systems. His research has significantly influenced the early development of Energy and AI as a young, interdisciplinary field internationally.
The future sustainable development of novel energy generation and materials manufacturing relies on radical innovations in chemical processes with highly embedded functionality, integration and multi-physics interactions. Prof Xuan has led the development of novel multiscale predictive models beyond the state of art for complex chemical and energy systems, and has delivered affordable, inclusive low-carbon solutions to tackle global climate change challenges. His work on advanced modelling has influenced the fundamental understanding of complex flow and chemical systems, by having discovered a number of important reaction/fluid phenomena at microscale, and has impacted on the development of a series of energy devices, such as fuel cells, electrolysers and solar fuel reactors.
His current research is focusing on the digital transformation of complex chemical and energy processes via integrated smart sensing, advanced modelling and data-centric deep learning, with a vision to deliver a paradigm-shift in how future materials, processes and systems can be designed, optimised and self-evolved throughout their entire lifecycle, enabling connected products and services, and making them super-efficient, zero-loss, whilst maximising their value creation.
Circular Chemical Economy
Prof Xuan is the Director of the £4.5 million UKRI Interdisciplinary Centre for Circular Chemical Economy. It brings together stakeholders from academia, industry, government, NGOs and general public to transform the UK’s chemical industry into a fossil-independent, climate-positive and environmentally-friendly circular economy. The Centre is playing a key role in helping the UK to reduce waste and the environmental impacts of production and consumption and creating opportunities for new UK industries, which has been recognised as the winner of the IChemE Global Award for Sustainability 2023 and the RSC Environment, Sustainability and Energy Horizon Prize: John Jeyes Prize 2025.
EPSRC Open Fellowship
Prof Xuan holds a prestigious EPSRC Open Fellowship to develop the next generation of clean energy devices using advanced artificial intelligence (AI). The five-year £2 million fellowship will enable Prof Xuan to develop novel explainable AI (XAI) tools and models which lead to an automated loop of materials design, manufacturing and testing of electrochemical materials and devices. Prof Xuan will work with industrial partners from Siemens PSE, Intelligent Energy and Johnson Matthey.
Flue2Chem:
Building a UK value chain in converting industrial waste gases into sustainable materials for consumer products
Prof Xuan is leading the Surrey team as part of the Flue2Chem project, which is a £5.4m, two-year, Innovate UK programme spearheaded by Unilever. In the project, industry giants in the UK are joining forces in the first-ever cross-sector collaboration aimed at reducing greenhouse gas emissions, with the aims to convert industrial waste gases into chemicals that can be used to manufacture superior and more sustainable consumer products. The Flue2Chem Project is featured as a case study in the UK government's Advanced Manufacturing Plan, released in December 2023.
Research interests
Professor Xuan’s research focuses on net zero energy, circular economy and sustainable development via digital and engineering innovations. He is leading the development of bespoke AI and digital solutions to enable next generation energy and chemical materials, devices, processes and systems. His research has significantly influenced the early development of Energy and AI as a young, interdisciplinary field internationally.
The future sustainable development of novel energy generation and materials manufacturing relies on radical innovations in chemical processes with highly embedded functionality, integration and multi-physics interactions. Prof Xuan has led the development of novel multiscale predictive models beyond the state of art for complex chemical and energy systems, and has delivered affordable, inclusive low-carbon solutions to tackle global climate change challenges. His work on advanced modelling has influenced the fundamental understanding of complex flow and chemical systems, by having discovered a number of important reaction/fluid phenomena at microscale, and has impacted on the development of a series of energy devices, such as fuel cells, electrolysers and solar fuel reactors.
His current research is focusing on the digital transformation of complex chemical and energy processes via integrated smart sensing, advanced modelling and data-centric deep learning, with a vision to deliver a paradigm-shift in how future materials, processes and systems can be designed, optimised and self-evolved throughout their entire lifecycle, enabling connected products and services, and making them super-efficient, zero-loss, whilst maximising their value creation.
Circular Chemical Economy
Prof Xuan is the Director of the £4.5 million UKRI Interdisciplinary Centre for Circular Chemical Economy. It brings together stakeholders from academia, industry, government, NGOs and general public to transform the UK’s chemical industry into a fossil-independent, climate-positive and environmentally-friendly circular economy. The Centre is playing a key role in helping the UK to reduce waste and the environmental impacts of production and consumption and creating opportunities for new UK industries, which has been recognised as the winner of the IChemE Global Award for Sustainability 2023 and the RSC Environment, Sustainability and Energy Horizon Prize: John Jeyes Prize 2025.
EPSRC Open Fellowship
Prof Xuan holds a prestigious EPSRC Open Fellowship to develop the next generation of clean energy devices using advanced artificial intelligence (AI). The five-year £2 million fellowship will enable Prof Xuan to develop novel explainable AI (XAI) tools and models which lead to an automated loop of materials design, manufacturing and testing of electrochemical materials and devices. Prof Xuan will work with industrial partners from Siemens PSE, Intelligent Energy and Johnson Matthey.
Flue2Chem:
Building a UK value chain in converting industrial waste gases into sustainable materials for consumer products
Prof Xuan is leading the Surrey team as part of the Flue2Chem project, which is a £5.4m, two-year, Innovate UK programme spearheaded by Unilever. In the project, industry giants in the UK are joining forces in the first-ever cross-sector collaboration aimed at reducing greenhouse gas emissions, with the aims to convert industrial waste gases into chemicals that can be used to manufacture superior and more sustainable consumer products. The Flue2Chem Project is featured as a case study in the UK government's Advanced Manufacturing Plan, released in December 2023.
Publications
Highlights
Recent representative publications
- Jiao K, Xuan J, Du Q, Bao Z, Xie B, Wang B, Zhao Y, Fan L, Wang H, Hou Z, Huo S, Brandon NP, Yin Y, Guiver MD, Designing the next-generation of proton exchange membrane fuel cells, Nature, 2021, 595, 361-369
- Niu Z, Pinfield VJ, Wu B, Wang H, Jiao K, Leung DYC, Xuan J*, Towards the digitalisation of porous energy materials: Evolution of digital approaches for microstructural design, Energy & Environmental Science, 2021, 14, 2549-2576. (Highlighted as journal front cover)
- Niu Z, Zhao W, Wu B, Wang H, Lin W, Pinfield VJ, Xuan J*, π Learning: A Performance-informed framework for microstructural electrode design, Advanced Energy Materials, 2023, 13, 2300244. (Highlighted as journal front cover)
- Leong KW, Pan W, Yi X, Luo S, Zhao X, Zhang Y, Wang Y, Mao J, Chen Y, Xuan J, Wang H, Leung DYC, Next-generation magnesium-ion batteries: The quasi-solid-state approach to multivalent metal ion storage, Science Advances, 2023, 9, eadh1181.
- Pan W, Zhao Y, Mao J, Wang Y, Zhao X, Leung KW, Luo S, Liu X, Wang H, Xuan J, Yang S, Chen Y, Leung DYC, High-energy single-walled carbon nanotube cathode for aqueous Al-ion battery boosted by multi-ion intercalation chemistry, Advanced Energy Materials, 2021, 11, 2101514. (Highlighted as journal back cover)
- Wang B, Zhang G, Wang H, Xuan J*, Jiao K, Multi-physics-resolved digital twining of proton exchange membrane fuel cells with a data-driven surrogate model, Energy and AI, 2020, 1, 100004.
- Lu X, Zhu C, Wu Z, Xuan J, Francisco JS, Wang H, In-situ observation of the pH gradient near the gas diffusion electrode of CO2 reduction in alkaline electrolyte, Journal of the American Chemical Society, 2020, 142, 15438–15444. (Highlighted as journal front cover)
- Xu H, Ma J, Tan P, Chen B, Wu Z, Zhang Y, Xuan J*, Ni M, Towards online optimisation of solid oxide fuel cell performance: combining deep learning with multi-physics simulation, Energy and AI, 2020, 1, 100003.
- Zhakeyev A, Wang P, Zhang L, Shu W, Wang H, Xuan J*, Additive manufacturing: Unlocking the evolution of energy materials, Advanced Science, 2017, 4, 1700187. (Most Accessed Article in November 2017)
- Wang B, Prinsen P, Wang H, Bai Z, Wang H, Luque R, Xuan J*, Macroporous materials: microfluidic production, functionalization and application, Chemical Society Reviews, 2017, 46, 855-914.
The electrochemical conversion of biomass-derived compounds into value-added chemicals using renewable electricity has attracted attention as a promising pathway for sustainable chemical production, with the electrooxidation of cyclohexanol being a typical example. However, optimizing and upscaling these processes have been hindered due to a limited understanding of the underlying mechanisms and limiting factors. To address this, there is a critical need for experimental tools that enable more efficient and reproducible measurements of these complex processes. In this work, we develop an automated microfluidic platform and use it to conduct controlled and efficient measurements of cyclohexanol electrooxidation on nickel electrodes under various electrolyte compositions and flow rates. The platform features microchannel networks integrated with multiple analytical instruments such as pumps, an electrochemical workstation, and a digital microscope to perform laboratory functions including electrolyte preparation, reaction control, microscopy, and electrochemical characterization, all streamlined through automation. Cyclohexanol electrooxidation on nickel is found to follow Fleischmann's mechanism, with an irreversible heterogeneous reaction as the rate-determining step. The effects of ionic and nonionic surfactant additives are screened, both demonstrating the ability to enhance current densities through different mechanisms. The developed platform is readily transferable for measuring other power-to-chemical processes and is believed to be a powerful tool for accelerating the understanding and development of sustainable electrosynthesis.
Generative Artificial Intelligence is a rapidly developing area being used to create powerful tools which have the potential to change a wide range of professional practices in chemical engineering. As this area develops, new principles on responsible use of Generative AI in chemical engineering are required to ensure that traditional engineering ethics are able to accommodate the new landscape. In this perspective, we assess the current state of engineering ethics, responsible AI principles and suggest how they can combine to ensure that Generative AI can be used responsibly within the chemical engineering sector. Whilst there are many aspect to engineering ethics and responsible AI use, the core principles which include transparency, integrity, and accountability are omnipresent and provide a shared foundation of good practice on which new regulations may be built as the need arises. Future breakthrough will require development on the AI technology itself, the people-centre approach and regulation changes.
This novel study presents an effective comprehensive life cycle assessment (LCA) of a novel sustainable carbon dioxide capture and utilization (CCU) system to co-produce alcohol ethoxylate (AE7), a valuable surfactant (a high-value chemical component of liquid detergents), and low-medium distillate range liquid fuel. Conventionally, AE7 is produced by reacting fatty alcohols with ethylene oxide from mostly fossil and marginally bio-based resources. This research develops novel AE7 production using carbon sources from flue gas of paper and steel industries, addressing a critical gap in the literature. The core process is Fischer-Tropsch (FT) synthesis using syngas formed by the reverse-water-gas-shift reaction, where recycled CO2 reacts with H2. FT produces C11-C13 alkanes and a light-to-medium fuel co-product. The alkanes are converted into C12-C14 fatty alcohols through dehydrogenation, hydroformylation, and hydrogenation. Fatty alcohols react with ethylene oxide to form AE7. The yields (w/w) of AE7 and the fuel co-products are 3.7 % and 3.4 % for paper industry flue gas, and 8.0 % and 9.5 % for steel industry flue gas, respectively. Renewable (wind) electricity meets the hydrogen demand and electricity needs for the reactions, a total of 13.4 and 33.3 kWh/kg flue gas, respectively. The life cycle impact assessment includes global warming potential (GWP) and other impacts using ReCiPe, Impact+ , and Product Environmental Footprint methods. Baseline scenarios show GWP ranging from 2.2 to 3.6 kg CO2e/kg surfactant for conventional cradle-to-gate AE production systems. The new systems have GWP ranging 0.4–1.3 kg CO2e/kg flue gas (cradle-to-gate) using mass allocation. Meanwhile, the paper industry’s flue gas system has biogenic CO2, while the steel industry’s CO2 is fossil-based. Considering the GWP reductions due to biogenic CO2 contents, their overall GWP is 2.56 kg CO2e and 10.33 kg CO2e per kg of product (AE7 +fuel) (cradle-to-grave) using economic allocation. Thus, biogenic CCU is critical for the sustainable co-production of high-value surfactants and fuel.
In order to utilize different wavelengths of rays in the solar spectrum and improve the power generation efficiency of solar systems, a novel linear Fresnel solar CPV/T hybrid system with advanced film beam splitter is proposed in this study. The system mainly consists of a Linear Fresnel Reflector (LFR) concentrator, the PV utilization sub-system, the thermal utilization sub-system, and the film beam splitter, where the thermal utilization sub-system includes a thermal receiver tube and a matching secondary reflector. The film beam splitter is designed by using the Needle method, and the average reflectance and transmittance of the film beam splitter are 26.43% and 73.57% under the full solar spectrum, respectively. The optical performance and thermodynamic analysis of the system are conducted through Monte Carlo Ray Tracing (MCRT) and Computational Fluid Dynamics (CFD) simulation methods. The optical performance results show that the concentrator can provide relatively high concentrating uniformity and the optimal installation height of the thermal receiver tube is about 1446.0 mm. The overall optical efficiency of the novel linear Fresnel solar CPV/T hybrid system can be maintained above 90% when the incident deviation angle is controlled to be within 0.1°. The thermodynamic analysis results indicate that utilizing water at 10 bar as the internal heat transfer medium (HTM) within the thermal receiver tube can effectively enhance the system’s thermal performance through the strategic reduction of the operating temperature of the solar cells and the inlet HTM temperature of the thermal receiver tube, coupled with an increase in the inlet HTM velocity. At the operating temperature of solar cells of 303.0 K, the inlet HTM temperature of 293.0 K and the velocity of the thermal receiver tube of 0.005 m s-1, respectively, the system attains a total output power of 7146.3 W and an exergy efficiency of 25.98%. [Display omitted] •A novel linear Fresnel solar CPV/T hybrid system based on film beam splitter is proposed.•Various designs of the film beam splitter on silicon CPV cell performance are studied.•Reflectance and transmittance of the film beam splitter are used as performance indicators.•The CPV/T hybrid system achieves improved concentrating uniformity.•The total output power and exergy efficiency attain 7146.3 W and 25.98%, respectively.
•Novel TEA of AE7 surfactant production using CO2 from steel industry flue gas•CO2 conversion rates around 3% in different processing capacities•Green hydrogen costs are the biggest factor influencing minimum selling price (MSP)•Lowest MSP of $8.77/kg exceeds the forecasted $3.75/kg for fossil-based AE7•Monte Carlo simulation shows a 21% chance of positive NPV vs. bio-based surfactants Successfully transitioning to a net-zero and circular carbon economy requires adopting innovative technologies and business models to capture CO2 and convert it into valuable chemicals and materials. Given the high economic costs and limited funding available for this transition, robust economic modelling of potential circular carbon pathways is essential to identify economically viable routes. This study introduces a novel techno-economic analysis (TEA) of producing alcohol ethoxylate (AE7), a valuable surfactant, from industrial flue gas. Traditionally, AE7 is produced by reacting fatty alcohols with ethylene oxide derived from fossil or bio-based sources. This research explores a method using CO2 captured from steel industry flue gas to produce AE7, addressing a notable gap in the literature. It evaluates a thermo-catalytic pathway involving Fischer-Tropsch (FT) synthesis with syngas generated by the reverse-water gas-shift reaction, where CO2 reacts with H2. CO2 conversion rates range around 3% across processing capacities of 25 kt/a, 100 kt/a, and 1000 kt/a. The study finds that the CO2 mass fraction concentration in the process emission is 2.47 × 10–5, compared to 0.13 in the incoming flue gas, highlighting the system's positive environmental impact. A radial basis function neural network was built to forecast the long-term average price of fossil-based and bio-based surfactants to benchmark the results against. Economic analysis reveals that the cost of green hydrogen significantly impacts the minimum selling price (MSP), making cost parity with existing fossil-based surfactants challenging. The lowest MSP of $8.77/kg remains above the long-term forecasted price of $3.75/kg for fossil-based C12–14 AE7. However, Monte Carlo simulations show a 21% probability of achieving a positive net present value (NPV) compared to leading bio-based surfactant alternatives. Sensitivity analyses identify capital costs, the price of low-carbon hydrogen (LCOH), and diesel prices as the most influential factors affecting the MSP. Continued advancements in Fischer-Tropsch catalyst technologies, reductions in green hydrogen costs and growing consumer demand for environmentally friendly products could significantly enhance the economic feasibility of this sustainable approach, paving the way for broader adoption and contributing to a circular carbon economy. [Display omitted]
Designing high‐performance porous electrodes is the key to next‐generation electrochemical energy devices. Current machine‐learning‐based electrode design strategies are mainly orientated toward physical properties; however, the electrochemical performance is the ultimate design objective. Performance‐orientated electrode design is challenging because the current data driven approaches do not accurately extract high‐dimensional features in complex multiphase microstructures. Herein, this work reports a novel performance‐informed deep learning framework, termed π learning, which enables performance‐informed microstructure generation, toward overall performance prediction of candidate electrodes by adding most relevant physical features into the learning process. This is achieved by integrating physics‐informed generative adversarial neural networks (GANs) with convolutional neural networks (CNNs) and with advanced multi‐physics, multi‐scale modeling of 3D porous electrodes. This work demonstrates the advantages of π learning by employing two popular design philosophies: forward and inverse designs, for the design of solid oxide fuel cells electrodes. π learning thus has the potential to unlock performance‐driven learning in the design of next generation porous electrodes for advanced electrochemical energy devices such as fuel cells and batteries. An innovative performance‐informed deep learning framework is demonstrated for microstructural fuel cell electrode design. The framework is applied to two popular electrode design philosophies; forward and inverse design, demonstrating its versatility and robustness. This work paves an efficient way for designing novel electrode microstructures with direct performance evaluation for advanced electrochemical energy devices beyond fuel cells.
The drive for efficiency improvements in CO2 capture technologies continues to grow, with increasing importance given to the need for flexible operation to adapt to the strong fluctuations in the CO2-rich flue gas flow rate and CO2 concentration. Using renewable energy can improve the environmental benefit of CO2 capture technologies; however, renewable energy resources often suffer from the challenge of non-uniform power generation as a result of weather and seasonal variations. In this work, we aimed to dynamically self-optimise the CO2 capture process in a renewable energy system via enhanced weathering of calcite with fresh water in a packed bubble column (PBC) reactor, in which CO2 from flue gas produced by a power plant is converted into bicarbonate and stored in the ocean. Data-driven surrogate dynamic models of the PBC reactor are developed to predict the reactor CO2 capture rate (CR) and power consumption (PC) and are trained using the data generated by physics-based models. Two deep learning models are considered to capture the dynamics of the PBC reactor: a long short-term memory network (LSTM); and a two-stage multilayer perceptron network (MLP). Data-driven models based on LSTM were developed to predict wind energy (R2: 0.908) and inlet flue gas CO2 concentration (R2: 0.981) using publicly available datasets. A multi-objective NSGA-II genetic algorithm is then applied that utilised the inlet flue gas CO2 concentration and wind energy predictions to pre-emptively self-optimise the reactor process conditions (i.e., superficial liquid flow rate and superficial gas flow rate) to maximise the carbon capture rate and minimise non-renewable energy consumption. The results should that by using the dynamic modelling and predictive multi-objective optimisation framework proposed within this study, the PCB reactor CR increased by an average of 16.7% over a one-month operation, whilst simultaneously reducing the proportion of now-renewable energy consumed from an average of 92.9% to an average of 56.6%. Overall, this study demonstrates the effectiveness of a dynamic data-driven modelling and multi-objective optimisation approach to increase the operational flexibility of CO2 capture reactors to adapt to strong fluctuations in flue gas and intermittent renewable energy supply.
•Linear Fresnel solar collector is designed using enhancer.•Heat transfer and flow characteristics with various enhancers are studied.•Lower rotation rate benefits the enhanced performance of Kenics mixer insert.•Overall optimum case achieves PEC value ranging 1.47∼1.73 compared to plain case. Linear Fresnel collector system is a type of solar thermal utilization installation. It is mainly used to focus solar radiation on the receiver tube to generate high temperature thermal energy which can be applied in many aspects. This study investigates five different types of receiver tube models using water as the flowing medium, which leads to improving heat transfer performance by means of being equipped with enhancers. The optical results and thermohydraulic performance of the Linear Fresnel collector system are evaluated through Monte Carlo Ray Tracing and Computational Fluid Dynamics (CFD) simulation methods. The obtained optical results reveal that the distribution of energy flux density concentrating on the receiver tube surpasses that of the parabolic trough collector system. In order to maintain an energy reception rate of 90.0% or higher, the installation height range of the receiver tube should be between 1468–1532 mm. When the sun-tracking error increases from 0.0° to 1.0°, the overall optical efficiency of the Linear Fresnel collector system decreases from 98.9% to 79.7%. The thermohydraulic performance shows that the enhancers can generate a swirling flow as its main feature, which effectively promotes the homogenization of the temperature field and destroys the boundary layer near the receiver tube wall, thus greatly improving the convective heat transfer in the receiver tube. However, the introduction of the enhancers also means that the pressure drop loss of the receiver tube increases. Furthermore, the impact of the Kenics mixer's enhancer on heat transfer and flow characteristics is further amplified as the rotation rate decreases, owing to its alternating and complex structure. Through a comparative analysis of the influence of different receiver tube models on Nusselt number (Nu), Frictional resistance coefficient (f) and comprehensive evaluation criterion (PEC) under four rotation rate conditions (y = 6.3, 8.1, 11.34, 18.9), the conclusions present that the receiver tube with an enhancer at a low rotation rate has a larger Nu than that at a high rotation rate, while the Frictional resistance coefficient changes little at different rotation rates. Therefore, the PEC is higher when the receiver tube is equipped with a low-rotation-rate enhancer. Moreover, the receiver tube equipped with a Kenics mixer at a rotation rate of 6.3 was found to be the overall optimum case, with the PEC value varying from 1.47 to 1.73. [Display omitted]
Green hydrogen from water electrolysis is a key driver for energy and industrial decarbonization. The prediction of the future green hydrogen cost reduction is required for investment and policy-making purposes but is complicated due to a lack of data, incomplete accounting for costs, and difficulty justifying trend predictions. A new AI-assisted data-driven prediction model is developed for an in-depth analysis of the current and future levelized costs of green hydrogen, driven by both progressive and disruptive innovations. The model uses natural language processing to gather data and generate trends for the technological development of key aspects of electrolyzer technology. Through an uncertainty analysis, green hydrogen costs have been shown to likely reach the key target of
Proton exchange membrane (PEM) based electrochemical systems have the capability to operate in fuel cell (PEMFC) and water electrolyser (PEMWE) modes, enabling efficient hydrogen energy utilisation and green hydrogen production. In addition to the essential cell stacks, the system of PEMFC or PEMWE consists of four sub-systems for managing gas supply, power, thermal, and water, respectively. Due to the system's complexity, even a small fluctuation in a certain sub-system can result in an unexpected response, leading to a reduced performance and stability. To improve the system's robustness and responsiveness, considerable efforts have been dedicated to developing advanced control strategies. This paper comprehensively reviews various control strategies proposed in literature, revealing that traditional control methods are widely employed in PEMFC and PEMWE due to their simplicity, yet they suffer from limitations in accuracy. Conversely, advanced control methods offer high accuracy but are hindered by poor dynamic performance. This paper highlights the recent advancements in control strategies incorporating machine learning algorithms. Additionally, the paper provides a perspective on the future development of control strategies, suggesting that hybrid control methods should be used for future research to leverage the strength of both sides. Notably, it emphasises the role of artificial intelligence (AI) in advancing control strategies, demonstrating its significant potential in facilitating the transition from automation to autonomy.
This paper reports the first theoretical modeling study of air-breathing microfluidic fuel cells (MFCs). The model is based on a semi-empirical Graetz–Damköhler (Gz–Da) analysis. The theoretical formulas derived clearly demonstrate the effects of the MFC design and operational parameters on the electrochemical activities of MFCs in quantitative detail. The modeling analysis shows that the electrode kinetics have significant effects on the trade-off relationship between the fuel utilization and current density. Moreover, the analysis reveals that the co-laminar flow of MFCs limits the fuel utilization considerably. ► A theoretical model of air-breathing microfluidic fuel cells (MFC) is reported. ► Pe–Da analysis for MFC is performed. ► Electrode kinetics is important for fuel utilization–current relationship. ► Co-laminar flow of MFC is largely the limiting factor causing low fuel utilization.
Nickel-yttrium stabilized zirconium (Ni-YSZ) nanocomposite were synthesized by to different paths: mechanochemical method and a novel sol-gel method at different temperatures. Preliminary structural/textural results obtained by XRD, BET and SEM analyses showed a typical crystalline cubic structure, with small crystallite size in those nanocomposites synthesized by sol-gel method as well as higher specific surface area and uniform distribution. (C) 2017 The Authors. Published by Elsevier Ltd.
Graphene microspheres are fabricated through a 3D-printed inkjet nozzle based on the gas-liquid microfluidic method. This method realizes rapid and controllable fabrication of uniform graphene microspheres with up to 800 mu L min(-1) (ca. 1 L d(-1)) of yields, which is 2 orders of magnitude higher than those of the conventional microfluidic method. The diameter of the graphene microspheres could be flexibly controlled from 0.5 to 3.5 mm by adjusting the gas pressure. The porous graphene microspheres show great dye decoloration performance. The maximum adsorption capacity of methylene blue is 596 mg/g, which is the highest adsorption capacity among that of the reduced graphene-oxide absorbents. A performance improvement of 21% is obtained by applying sodium alginate into graphene as a curing agent. The adsorption behavior follows a Langmuir isotherm and pseudo-second-order kinetic model. Besides, the graphene microspheres exhibit great selective adsorption and could separate cationic dye methylene blue (MB) and anionic dye methyl orange (MO).
Energy storage capacity has been a major limiting factor in pursuit of increasing functionality and mobility for portable devices. To increase capacity limits, novel battery designs with multi-electron redox couples and increased voltages have been listed as a priority research direction by the US Department of Energy. This study leverages the benefits of microfluidics technology to develop a novel mixed-pH media aluminum-air cell which incorporates the advantages of the trivalence of aluminum and mixed-pH thermodynamics. Experimentally, the new cell exhibited an open circuit potential of 2.2 V and a maximum power density of 176 mW cm(-2), which are respectively 37.5% and 104.6% higher than conventional single alkaline aluminum-air cell under similar conditions. With further optimization of channel thickness, a power density of 216 mW cm(-2) was achieved in the present study. (C) 2015 Elsevier Ltd. All rights reserved.
Energy storage and conversion is a very important link between the steps of energy production and energy consumption. Traditional fossil fuels are natural and unsustainable energy storage medium with limited reserves and notorious pollution problems, therefore demanding for a better choice to store and utilize the green and renewable energies in the future. Unitized regenerative fuel cell (URFC), a compact version of regenerative fuel cell with only one electrochemical cell, is one of the competent technologies for this purpose. A URFC can produce hydrogen fuel through an electrolysis mode to store the excess energy, and output power in a fuel cell mode to meet different consumption requirements. Such a reversible system possesses several distinctive advantages such as high specific energy, pollution-free, and most importantly, the decoupled energy storage capacity with rated power. Based on the different electrolytes utilized, current available URFC technologies include the most common proton exchange membrane (PEM)-based URFC, and other types of URFC such as the alkaline, solid oxide and microfluidic URFCs. This part of the URFC review emphasizes on the PEM-based URFC. Specifically, the research progress on both cell components and systematic issues is introduced. Benefiting from its fairly mature technology stage, the PEM-based URFC has already been applied in aerospace and terrestrial areas. However, for large-scale application, their cost and efficiency are still the obstacles when competing with other energy storage technologies. As for the alkaline, solid oxide and microfluidic types of URFC, their research progress is reported independently in part B of this review.
Fuel cells and solar energy are promising candidates for electricity generation. It is forecast that fuel cells and solar power systems will play an important role in reducing the greenhouse gas footprint and replacing fossil fuels. Therefore, the limitations of fuel cells and solar power systems, such as low efficiency, high cost, and low reliability, must be addressed appropriately to enable their full potentials. Metal foam is a new class of material that has gained immense attention due to its excellent properties suitable for a wide range of applications. Its unique characteristics distinguish it from typical solid metals. The properties of metal foam can be modified during the fabrication stage by manipulating its physical structure. The goal of this paper is to review the application of metal foam in fuel cells and solar power systems. Besides, the performance of metal foam in fuel cells and solar systems is also discussed. Metal foam has been applied to the electrodes, gas diffusion layer and flow field of fuel cells to enhance performance, especially in regard to current density and flow distribution. Furthermore, metal foam is a heat exchanger for the solar energy harvesting system to improve its efficiency. Superior performances in experimental testing allows the possibility of commercialization of metal foam products in the renewable energy field.
Non-precious-metal single atom (NPM-SA) confined nanomaterials with maximum metal atom utilization have recently attracted particular interest, providing opportunities for exploiting novel heterogeneous electrocatalysts with low cost and high efficiency. The applications of non-precious-metal single-atom catalysts (NPM-SACs) have been extensively developed in electrochemical energy technologies such as fuel cells, metal-air batteries, and hydrogen production via water splitting. NPM-SACs can be confined on substrates with various structures and dimensions from 1D to 3D. However, the role of the structural dimensions of substrates has not been critically summarized. In this review, recent advances in NPM-SACs such as facile synthesis, characterization, performance in catalyzing oxygen redox reactions, and related applications are highlighted through a focus on different dimensional substrates (1D, 2D, and 3D). The general fabrication strategies and the catalytic mechanism of NPM-SACs in the oxygen reduction reaction (ORR) and oxygen evolution reaction (OER) are extensively discussed. Moreover, the opportunities and challenges in this emerging field are presented on the basis of its current development.
International water security has become unprecedentedly complicated, therefore, effective and selective removal of hazardous materials, especially toxic heavy metal ions, are significant for effluent purification. In this regard, ion-imprinted polymers with special recognition cavities have received much attention. However, configuration screening and performance optimization of functional materials by trial-and-error design method is undoubtedly time- and money-consuming. In this study, high-performance ion-imprinted chitosan microspheres (ICSMs) were successfully designed via density functional theory (DFT) calculation and synthesized via facile microfluidic technology. As-synthesized ICSMs exhibited highly uniform morphology (Dav = 420.6 µm, CV = 3.6%) and ultra-high adsorption capacity (qmax = 107.12 mg g−1). The adsorption isotherm was best fitted to the Langmuir model while the kinetic data followed the pseudo-second order model, indicating a dominant role of chemisorptions. Also, ICSMs displayed satisfactory stability and reusability (95.34 mg g−1, after 5 cycles). Moreover, the selective adsorption mechanism was quantitative revealed by electronegativity, electrophilicity index, adsorption energy (Ea) and bond length. This study is expected to lay a foundation for high-performance biosorbents design and synthesis for future water remediation. [Display omitted] •High-performance ICSMs were obtained by DFT-guided design and microfluidic synthesis.•Ultra-high adsorption property for targeted metal ions (107.12 mg g−1) was proved.•Target ions selective removal from mono- and multi-component systems was achieved.•Selectivity mechanism was quantitative revealed by adsorption energy and bond length.•Superior and stable re-adsorption efficiency (> 95 mg g−1 after 5 cycles) was verified.
•A formic acid microfluidic fuel cell based on counter-flow design is proposed.•Highest fuel utilization of 91.4% is obtained at 1μLmin−1.•Pump energy consumption is reduced to minimal.•Ohmic resistance in electrolyte is the major performance limiting factor. Microfluidic fuel cell (MFC) is a promising energy source for portable applications, which draws lots of R&D attention. However, MFCs fed with hydrocarbon fuel like formic acid suffers low fuel utilization problem because of sluggish kinetics, complicate reaction condition and dilemma on cell control. In this work, a formic acid MFC based on counter-flow design is proposed. This counter-flow structure is verified a promising design for high Graetz number operation, which is especially beneficial for high fuel utilization manipulation of MFC. A breakthrough in fuel utilization is achieved and the highest fuel utilization of 91.4% is obtained at 1μLmin−1. It is revealed that counter-flow MFC is capable for low flow rate operation, which is significant for reduce the pump energy consumption and improve the energy efficiency of MFC system. Each potential loss involved in counter-flow MFC is categorized and it is found that potential loss caused by internal resistance hinders performance mostly.
► Limiting factors of air-breathing laminar-flow based fuel cell (LFFC) is analyzed. ► A numerical model for LFFC is developed. ► Air breathing process is not a limiting factor at the present stage. ► Oxygen starvation is significant when the cell current density exceeds 200mAcm−2. Laminar flow-based fuel cell (LFFC) is a relatively new type of fuel cell that does not require the use of proton exchange membrane. While the first-generation LFFC uses dissolved oxygen at the cathode, the second-generation LFFC (2G-LFFC) adopts a more advanced air-breathing design for achieving high power density. The architecture and operational mechanisms of a 2G-LFFC are more complex. In order to gain detailed understanding of the 2G-LFFC, an integrated CFD/electrochemical kinetics modeling study has been conducted to analyze the cell limiting factors and sufficiency of the oxidant supply from air. It is found that under most typical operating conditions, the 2G-LFFC free-breathing mode can supply sufficient oxygen to the electrode reactive surface for cathode half-cell reaction, indicating that the air breathing process is not a limiting factor to the cell performance. However, oxygen starvation will become a major performance limiting factor when the anode is enhanced for higher current density. The results presented in this paper provide useful design guidance for future development of LFFC.
In this paper, a novel Multi-Stage Exhaust Energy Recycling strategy was proposed and optimized to maximize the system efficiency and performance of solid oxide fuel cell -combined heating and power (SOFC-CHP). Both process modeling and experiment work based on 1 kW SOFC-CHP systems were carried out to prove the concept and optimize the system. It is found that the system with multi -stage exhaust gas combustion (MS-EGC) will reduce the system operating temperature from 1149 degrees C to 830 degrees C, which significantly increases the safety of system operation and reduces the material requirement. The system combining MS-EGC with anode off gas recovery (MS-AOGR & EGC) leads to highest overall co-generation efficiency up to 92%. A coupled reactor integrating MS-EGC modules was developed and tested for a 1 kW SOFC system to realize the proposed strategy. The results showed that in MS-EGC, recycling of thermal energy at first stage rarely affects the chemical energy utilization in subsequent stages and the overall system performance, further confirming the advantages of the innovative multi-stage energy recycling strategy. (C) 2017 The Authors. Published by Elsevier Ltd. This is an open access article under the CC BY license
Proton-exchange membrane fuel cells (PEMFCs) are considered one of the viable solutions to the decarbonization of the transport sector. However, their performance and durability are yet to be competitive with internal engine vehicles due to the complex interaction of electrochemical and physical phenomena in PEMFCs. The electrochemical and physical phenomena that occurred in PEMFCs, including the polarization curves, profiles of the reactant and product species, velocities of species, as well as temperature distribution, could be described by coupling the reaction kinetics with the transport processes of mass, momentum, energy, and charge. In this chapter, the fundamentals and operating principles of PEMFCs are explained along with the governing equations that describe various electrochemical and multiphysics transport processes in PEMFCs.
For the next generation of proton exchange membrane (PEM) fuel cells, the conventional electrode with uniform distribution of functional components is urged to be replaced by functional graded electrode for the prominent performance, efficiency and avoid exacerbated catalyst cost. Due to the complex and non-linear behaviours of PEM fuel cell system, rapid and effective computational model and optimisation algorithm are required to handle such a complex relationship between electrode design parameters and cell performance. In this work, a multi-physics model with multi-directionally graded electrode is developed, in which a deep machine learning approach is embedded, to create a surrogate model for multi-objective optimisation empowered by non-dominated sort genetic algorithm (NSGA-II). A robust prediction deep neural network model with the mean square error lower than 0.01 is obtained from training and then coupling with NSGA-II to evaluate and optimise the fuel cell performances and cost. Remarkably, the Pareto front is successfully defining the trade-off relationship between the objectives where it aids to identify an optimum point where it satisfies the cost effectiveness while maintaining relatively high cell performances. Our work presents a promising strategy to optimise the fuel cell system with underlying interaction and allow rapid and accurate prediction and optimisation.
[Display omitted] •Factory-on-chip concept is demonstrated with multi-dimensional scale-up strategy.•Design principle is derived with the flow resistance model of a 3D microfluidic flow network.•A module with 80 channels is fabricated and investigated with two fluid systems.•Chitosan/TiO2 composite material is continuously synthesized and characterized. Droplet microfluidics provide an advanced platform for functional material synthesis. However, the process has been largely limited to the scale of laboratory study with the device known as lab-on-chip. Here, a multi-dimensional scale-up strategy based on modularised microfluidic reactors is presented to develop large-scale devices defined as factory-on-chip, achieving throughput enhancement to the industrial production scale. Under the guidance of the derived principle, an up-scaling system is demonstrated with eight microchannels parallelized to form an array, ten arrays stacked as the module, and five modules integrated in a system with 400 channels in total. Experiments showed that the circular array arrangement improved the uniformity of product droplets by 42.4% compared to that achieved with a parallel array. The stacking effect was also investigated with two types of material production systems. Chitosan/TiO2 composite microspheres, as advanced wastewater treatment material, were continuously synthesized in mass production with a narrow size distribution of 3.59%, which could hardly be achieved with conventional methods. The material exhibited a methyl-orange dye removal efficiency of 65.3%, which constitutes an improvement of 10.8% compared to single-component chitosan microspheres.
Titanium dioxide nanoparticles were obtained by using poly-acrylic acid gel in a two-step synthesis method at room temperature using titanium (IV) chloride as titanium source. The formed nanoparticles were studied by different characterization techniques, showing that different proportion of anatase/rutile phase can be obtained by adjusting the amount of acrylic acid in the initial gel. Aluminum storage performance of the synthesized materials was also investigated, obtaining higher capacity density results at higher rutile ratio (75.1 mAh g–1).
A dimensionless steady-state computational model was developed for microfluidic fuel cell (MFC) with flow-through porous electrodes. The dimensionless nature of the model featured with high versatility as a single set of modeling analysis could interpret a wide range of MFCs in terms of fuel cell properties, physical dimensions and scales. Based on the model, statistical Multi-Parametric Sensitivity Analysis (MPSA) was implemented to evaluate the effects of different parameters on the cell output. Eleven major parameters, grouped in terms of cell operation, charge transport, mass transport and porous structure, were investigated under different working conditions. These parameters were consequently classified into strong-, moderate- and weak-influencing factors. The results showed that the charge transfer coefficient and the equilibrium potential were the most influencing factors. The results indicated that the relationship between charge transfer coefficient and porous electrode properties needed to be further specified and the commonly used uniform equilibrium potential assumption of the porous electrode was questionable. Based on the results, guidance for the future modeling and experimental research on MFC was provided. (C) 2015 Elsevier Ltd. All rights reserved.
Sustainable chemistry for renewable energy generation and green synthesis is a timely research topic with the vision to provide present needs without compromising future generations. In the era of Industry 4.0, sustainable chemistry and process are undergoing a drastic transformation from continuous flow system toward the next level of operations, such as cooperating and coordinating machine, self-decision-making system, autonomous and automatic problem solver by integrating artificial intelligence, data and hardware in the cyber-physical systems. Due to the lack of convergence between the physical and cyber spaces, the open-loop systems are facing challenges such as data isolation, slow cycle time, and insufficient resources management. Emerging researches have been devoted to accelerating these cycles, reducing the time between multistep processes and real-time characterization via additive manufacturing, in-/on-line monitoring, and artificial intelligence. The final goal is to concurrently propose process recipes, flow synthesis, and molecules characterization in sustainable chemical processes, with each step transmitting and receiving data simultaneously. This process is known as ‘closing the loop’, which will potentially create a future lab with highly integrated systems, and generate a service-orientated platform for end-to-end synchronization and self-evolving, inverse molecular design, and automatic science discovery. This perspective provides a methodical approach for understanding cyber and physical systems individually, enabled by artificial intelligence and additive manufacturing, respectively, in combination with in-/on-line monitoring. Moreover, the future perspective and key challenges for the development of the closed-loop system in sustainable chemistry and process are discussed.
•Sophisticated physics-based reactor-scale models for EW-based CO2 capture were developed.•Two data-driven surrogate models, RSM and E-AHF, were developed.•Relationship between five design variables and three objective functions were investigated.•E-AHF surrogate model shows higher accuracy than the RSM approach.•Reactor performance was significantly improved after GA-based multi-variable optimisation. Enhanced weathering (EW) of minerals could potentially absorb atmospheric CO2 at gigaton scale per year and store it as bicarbonate and carbonate in the ocean. However, this process must be accelerated by engineered reactors, in which optimal reaction conditions maximise the CO2 capture rate and minimise the energy and water consumption. In this work, trickle beds (TBs) and packed bubble columns (PBCs), operated with fresh water and CO2 -rich flue gas, are chosen as typical chemical reactors to perform the EW-based CO2 capture. We firstly develop experimentally validated physics-based mechanistic models then generate data to train data-driven surrogate models to achieve rapid prediction of performance and multi-variable optimization. Two surrogate models, namely, response surface methodology (RSM) and extended adaptive hybrid functions (E-AHF), are developed and compared, in which the effect of five design variables on three objective functions are investigated. Results show that the R2 for the prediction of CO2 capture rate (CR) and water consumption (WC) through RSM and E-AHF is higher than 0.84. For TB reactors, in particular, the calculated R2 is higher than 0.96. The prediction accuracy of energy consumption (EC) through the RSM approach is, however, relatively poor (R2 ∼ 0.79), but is improved by using the E-AHF surrogate model, increasing to R2 ∼ 0.89. The developed data-driven surrogate model can rapidly predict the performance indicators of TB and PBC reactors without solving complex mechanistic models consisting of many partial differential equations. After optimization using the surrogate models, improvements were achieved in the objectives for TB and PBC reactors as follows: CR increased by 37.8 % and 13.1 %, EC reduced by 37.4 % and 23.8 %, and WC reduced by 12.5 % and 40.7 %, respectively.
Next-generation ultrahigh power density proton exchange membrane fuel cells rely not only on high-performance membrane electrode assembly (MEA) but also on an optimal cell structure. To this end, this work comprehensively investigates the cell performance under various structures, and it is revealed that there is unexploited performance improvement in structure design because its positive effect enhancing gas supply is often inhibited by worse proton/electron conduction. Utilizing fine channel/rib or the porous flow field is feasible to eliminate the gas diffusion layer (GDL) and hence increase the power density significantly due to the decrease of cell thickness and gas/electron transfer resistances. The cell structure combining fine channel/rib, GDL elimination and double-cell structure is believed to increase the power density from 4.4 to 6.52 kW L-1 with the existing MEA, showing nearly equal importance with the new MEA development in achieving the target of 9.0 kW L-1.
The dead-ended anode (DEA) and anode recirculation operations are commonly used to improve the hydrogen utilization of automotive proton exchange membrane (PEM) fuel cells. The cell performance will decline over time due to the nitrogen crossover and liquid water accumulation in the anode. Highly efficient prediction of the short-term degradation behaviors of the PEM fuel cell has great significance. In this paper, we propose a data-driven degradation prediction method based on multivariate polynomial regression (MPR) and artificial neural network (ANN). This method first predicts the initial value of cell performance, and then the cell performance variations over time are predicted to describe the degradation behaviors of the PEM fuel cell. Two cases of degradation data, the PEM fuel cell in the DEA and anode recirculation modes, are employed to train the model and demonstrate the validation of the proposed method. The results show that the mean relative errors predicted by the proposed method are much smaller than those by only using the ANN or MPR. The predictive performance of the two-hidden-layer ANN is significantly better than that of the one-hidden-layer ANN. The performance curves predicted by using the sigmoid activation function are smoother and more realistic than that by using rectified linear unit (ReLU) activation function.
Carbon is one of the most attractive electrode materials for electrochemical energy storage. An ideal electrode structure requires a pore distribution ranging from nanoscale to milliscale to simultaneously enable efficient mass transfer, enlarge the specific surface area, and minimize the electrical resistance. Here, a novel hybrid method to fabricate carbon electrodes with a designable hierarchical pore structure is presented. The proposed manufacturing combines stereolithography, pyrolysis, and chemical activation, which contribute to producing pores in millimeter, micrometer, and nanometer, respectively. The prepared hierarchical microarchitectural material outperforms the commercial carbon paper by five times in current density. Further enhancement in the electrochemical performance can be achieved through optimizing the distribution of hierarchical pores, which is proved feasible in the applications of vanadium redox flow battery and supercapacitor applications.
Microfluidic fuel cell (MFC) is a considered as a promising energy source for powering portable electronic devices due to low cost and easy control. However, the energy density of MFC is much lower than conventional batteries like lithium battery. Due to the fuel crossover, the optimized operation concentration remains 1-2M. In this paper, a counter-flow MFC is presented to achieve concentrated fuel. Density mismatch between fuel and electrolyte is the key cause for fuel crossover. Experimental and modeling study confirms that through adjusting different channel design according to fuel concentration, fuel crossover can be prevented.
The pH-differential membraneless architecture could enhance the thermodynamic property and raise the electrochemical performance of a dual electrolyte microfluidic reactor (DEMR) for electrochemical conversion of CO2. Freed from hindrances of membrane structure and thermodynamic limitation, DEMR demonstrates the possibility of altering anolyte and catholyte pHs to achieve higher reactivity rates and efficiencies. Different operation condition parameters of a microfluidic network would affect the reactor performance to a certain extents, constraining further improvement. Therefore, we conducted experimental analysis to study the mechanisms and intrinsic correlations of catalyst to Nafion ratio, microchannel thickness, electrolyte flow rate and CO2 supply for an optimized outcome. A comprehensive investigation on the cell durability was also carried out in the way of repetitiveness and long period operation, regarding both reactivity and efficiency. It was found that the catalyst to Nafion ratio affects the performance in a parabolic relation and there exists optimal values of electrolyte flow rate and microfluidic channel thickness for maximized cell performance. The influence of the reactant CO2 supply rate is not significant above a certain level where kinetics limitation is not dominant. The parametric study provides an operational point of view on the dual electrolyte microfluidic reactor and serves as a tool for DEMR optimization design. (C) 2016 Elsevier Ltd. All rights reserved.
High-performance monodispersed chitosan microparticles for methyl orange (MO) adsorption were synthesized on a microfluidic platform coupled with a cross-linking approach. Batch adsorption experiments were carried out to evaluate the capacity and kinetics of the as-prepared microparticles on the adsorption of MO. Due to the advantage of microfluidics that all the manipulations and operations are related to independent droplets, the prepared microparticles are controlled to within a narrow size distribution (CV = 1.86%) while exhibiting uniform high performance (adsorption capacity = 182 mg g(-1)). Adsorption experiments were carried out under various design and operation conditions. It is found that the adsorption isotherm was well described by the Langmuir model and the adsorption kinetics followed a pseudo-second-order kinetic model. The high performance together with biodegradable feature and low-cost raw material give the microfluidic-synthesized chitosan microparticles a promising potential for future dye effluent treatment.
A comprehensive multiphysics 3D model of an anode-supported planar reversible solid oxide cell (rSOC) with a half-channel-unit-cell geometry is created and validated. The physical phenomena that are modeled include reversible electrochemistry/charge transport, coupled with momentum/mass/heat transport. Several electrode microstructures comprising the homogeneous and functionally graded porosity distributions are applied to the validated model, to evaluate and compare the current-voltage (j-V) performance in both fuel cell mode and electrolysis mode. The results indicate that increasing the porosity in a homogeneous porous electrode does not always promote the cell's j-V performance. An optimal porosity emerges where the effect of porosity on the mass transport is maximized, which ranges between 0.5 and 0.7 in the working conditions of the present study. Compared with homogeneous porous electrodes, the heterogeneous porous electrode design with a functionally graded porosity distribution is found to be a potential option to better the overall j-V performance of the rSOC. Furthermore, it is discovered that theoretically grading the porosity in the width direction (i.e., increasing porosity from the center of each gas channel to the center of each adjacent rib) brings an outsize benefit on the cell's performance, compared to the traditional way of improving the porosity along the cell thickness direction.
Photocatalytic oxidation has been widely investigated and applied to perform degradation of organic pollutants in water and air. In recent technological advancement, photocatalysis (PC) is integrated into fuel cell (FC) to form photocatalytic fuel cell (PFC) for simultaneous wastewater treatment and production of electricity. In the PFC mechanisms, the organic pollutant, acting as a fuel in the fuel cell component, is decomposed upon light activation at the photoanode and the flow of photoexcited electrons is driven by the potential difference between the two electrodes. Thus, unwanted electron-hole recombination is effectively inhibited, resulting in enhanced PC activity. In other words, the chemical energy stored in the organic pollutant is recovered and converted into useful electricity during the wastewater treatment process. The photoelectrochemical technology can also be implemented for hydrogen generation and carbon dioxide reduction. Various strategies have been investigated for improving the PFC mechanisms through better visible-light photoelectrodes, innovative cell designs, dualphotoelectrode setup, as well as optimal control. In this review, the fundamentals and technological development of PFC will be discussed with special attention to novel cell configurations. With better knowledge and understanding of the PFC, we can identify promising research directions to further develop the PFC technologies.
Aluminum-air cell, with trivalence redox couples, attracts a lot of interest to break the capacity limitation of conventional batteries in pursuit of higher mobility for portable devices. In order to overcome the self-discharge issue of aluminum, a microfluidic aluminum-air cell working with methanol-based anolyte was developed in this work. The operation feasibility of this membraneless cell was first proved. Then, the influences of electrolyte concentration and water content in the methanol-based anolyte on cell performances were investigated. Experimentally, a high discharge capacity density of 2507 mAh/g was achieved at a low current of 0.015mA.
Air-cathode microfluidic fuel cells are promising micro-scale power sources that unfortunately undergo substantial performance loss at the cathode. This study therefore develops a mathematical model to gain a better understanding of the fundamental processes and polarization characteristics associated with the MFC air cathode operation so as to find strategies to minimize the cathode polarization. The model is solved for the four regions of an MFC cathode compartment (i.e. gas channel, gas diffusion layer, catalyst layer and electrolyte microchannel), and considers microfluidic flow, species transport, charge transport and multi-step oxygen reduction reactions. Relying on the model, transport and chemical patterns inside the MFC cathode compartment are examined. Corresponding electrode polarization behaviors are analyzed over a wide operating potential range including different forms of resistance. Through a series of model-based parametric studies, it is found that the internal transfer resistance slightly decreases with increasing catalyst layer porosity but can be effectively reduced through a proper control of electrolyte hydrodynamic conditions, indicating microfluidic technology is a powerful tool for enhancing electrochemical cells. Copyright (C) 2011, Hydrogen Energy Publications, LLC. Published by Elsevier Ltd. All rights reserved.
[Display omitted] •Amphoteric carboxymethyl chitosan/gelatin microspheres (CCGMs) with hierarchical porous structure were synthesized.•Ultra-high adsorption properties (383.142 mg g−1 for MO and 584.795 mg g−1 for MB) were demonstrated.•pH induced selective removal of cationic and anionic dyes from the binary mixed solution was achieved.•Selectivity mechanism was illustrated as the synergy of electrostatic interaction and π-π stacking.•Superior and stable re-adsorption efficiency, higher than 80% after 5 cycles, was realized. The substandard discharge of highly toxic dyestuff wastewater will pose a serious threat to the environment and human beings, even causing unimaginable irreversible damages. In the current study, novel amphoteric carboxymethyl chitosan/gelatin microspheres (CCGMs), synthesized by a facile inverse suspension route with two-step successive crosslinking process, were evaluated as a potential adsorbent for high efficient pH induced selective removal of azo-dyes. The as-prepared CCGMs were characterized using scanning electron microscopy (SEM), Fourier transform infrared spectroscopy (FT-IR), thermogravimetry analysis (TGA) and X-ray photoelectron spectroscopy (XPS). Various influential factors such as solution pH, temperature, and contact time were employed to ascertain the optimal condition for representative azo-dyes adsorption, including methyl orange (MO) and methylene blue (MB). The maximal adsorption of MO and MB on the CCGMs at pH values of 3 and 11 were 383.142 mg g−1 and 584.795 mg g−1, respectively. The adsorption mechanism were demonstrated as the synergistic effect of electrostatic interaction and π-π stacking between dyes and CCGMs. Besides, the outstanding and stable regeneration of as-prepared CCGMs were also verified with five consecutive recycling. Thus, the newly developed CCGMs could be a highly promising candidate for dyestuff wastewater treatment.
We investigate different operating conditions effects, including cathode relative humidity, anode stoichiometry and inlet pressure, on PEMFC with anode recirculation by conducting dynamic simulations. The performance improvement caused by the self-humidification effect is about 6.5% with dry cathode inlet, and it is very slight with fully humidified cathode inlet. Nitrogen fraction in the anode is low in the first 20 min under a low cathode relative humidity. A 0.3–0.6 cathode relative humidity might be suitable for the simulated cases. Generally, the fuel cell benefits from increasing anode stoichiometry by enhancing the self-humidification effect, decreasing the performance decline rate and ameliorating hydrogen distribution along the channel. Increasing anode inlet pressure and cathode inlet pressure play the mitigated and exacerbated role on voltage decline, respectively. However, increasing cathode inlet pressure can significantly improve output performance especially under a low cathode relative humidity. We suggest an appropriate low cathode relative humidity, increasing anode stoichiometry, and increasing anode and cathode inlet pressure by similar amount for PEMFC with anode recirculation. •Modeling of operating condition effect on anode recirculation PEMFC is presented.•An appropriate low cathode humidity is proposed for low nitrogen crossover rate.•High anode stoichiometry can improve fuel distribution and mitigate voltage decline.•Increasing anode and cathode inlet pressure by similar amount is recommended.
Microfluidic fuel cells and flow batteries are free from the static physical barrier that separated the anodic and cathodic compartments, introducing the advantages of low cost and feasible miniaturized application. Recently, the concept of dual electrolyte stream proves itself an effective strategy to enhance the reactor performance by pairing catholyte and anolyte with thermodynamically favored pHs. Being able to be implemented in both fuel cell and electrolyzer modes, the dual electrolyte strategy demonstrates superior peak power density, low overpotentials, high reactivity, and high efficiency. However, keeping the characteristics of laminar flow requires continuous electrolyte flowing in the microchannel. Besides, neutralization reaction would occur within the mixing layer between the catholyte and the anolyte, requiring higher flow rate to control the layer thickness. These lead to considerable electrolyte wastage that will significantly weaken the economical aspect and electrolyte utilization efficiency. To tackle this issue, this study investigated the electrolyte degradation process and proposed an operation scheme for electrolyte recycling. Key parameters of electrolytes were tracked and monitored by mimicking different reactor situations. Results indicated that with appropriately adjusted operating conditions, electrolyte recycling would be feasible in a microfluidic pH-differential network. Accordingly, an pH indicator for electrolyte recycling was proposed for potential practical application. •Electrolyte recycling feasible on dual electrolyte microfluidic networks.•Key operation conditions optimized for better recycling operation.•pH degradation predictable for practical applications.•Reactor performance can be controlled within a stable range.
Microfluidics has been proved a promising way for highly efficient lanthanide extraction and separation benefiting from the astounding mass transfer characteristics. Efforts have been devoted by us previously to provide a clear understanding of the separation intensification mechanism of lanthanide pairs with distinguished extraction kinetics in microfluidics, such as Eu 3+ /La 3+ . However, for those lanthanide pairs with closely similar extraction kinetics, the working principles of separation intensification are different, which could not be explained by our former proposed theory of nonequilibrium separation intensification. In this study, a numerical model considering nonlinear extraction kinetics was presented to comprehend the separation characteristics of Eu 3+ /Sm 3+ , a lanthanide pair with very similar extraction kinetics, in microfluidics. The hydrodynamic and kinetic approaches, i.e. flow rate, initial concentration of metal ions and extractant, pH and temperature, were studied to manipulate separation performance. Analyses on dimensionless number (Damköhler number) were conducted to unveil the working mechanism of such approaches. The numerical study provides a mechanistic understanding of lanthanide separation in microfluidics and offers manipulating principles for further optimization.
Solid oxide electrolysis cell (SOEC) is a novel approach to utilize excess renewable power to produce fuels and chemicals. However, the intermittence and fluctuation of renewable energy requires more advanced optimization strategy to make sure its performance in safety and cost-effectiveness. Here, we propose a hybrid model for the precise and quick optimization of the co-electrolysis process in the SOEC for syngas production, based on the multi-physics simulation (MPS) and deep learning algorithm. The hybrid model fully considers electrochemical/chemical reactions, mass/momentum transport and heat transfer, and presents a small relative error (96%). Various targets including the single-objective, dual-objective and multi-objective optimizations are evaluated with particular attentions on the reactant conversion rate and energy efficiency at different temperatures. The electrolysis efficiency is negatively correlated with the power supply in all strategies and thermal neutral condition (TNC) can be achieved at different temperatures, where 1023 K, 1053 K, 1083 K and 1113 K are corresponded to the TNC power range of 10-16 W, 14-23 W, 18-29 W and 22-37 W, respectively. This theory can be flexibly applied in the sustainable manufacturing and circular economy sectors and energy according to the optimization targets.
[Display omitted] •A facile microfluidic-based route to fabrication of PEI-CS biosorbents is reported.•The PEI-CS biosorbents exhibit outstanding selectivity towards target metal ions in complicated condition.•The PEI-CS biosorbents show ultra-high adsorption capacity towards Cu ions up to 146mgg−1.•The mechanical property of as-prepared PEI-CS biosorbents is over 0.34MPa.•The readsorption efficiency is still higher than 71.2% after 5 adsorption-desorption cycles. Water pollution is the most challenging issue facing mankind nowadays as a result of ever-increasing population and steadily improving life standards. More and more wastewater without effective treatments is arbitrarily discharged into the aquatic environment, which causes irreversible damage and incalculable loss. Especially, the industrial wastewater containing heavy metal ions, such as copper (Cu), cobalt (Co), and manganese (Mn), are toxic to human and living organisms even at low concentrations. A highly efficient and selective removal method has been long sought for heavy metal ions wastewater treatment. In this study, a highly monodispersed polyethylenimine-chitosan (PEI-CS) biosorbent, synthesized by an integrated process with facile microfluidic emulsion, chemical crosslinking, solvent extraction and chemical modification, was used as an efficient adsorbent to remove heavy metal ion from contaminated water. The as-prepared biosorbents demonstrated ultra-high adsorption capacity of 146mgg−1 towards Cu ions, almost triple the performance reported in literature. Besides, PEI-CS biosorbents were also endowed with good mechanical strength, excellent adsorption selectivity towards targeted ion in the presence of other metal ions with different valence states, and high reusability. Therefore, the newly developed PEI-CS biosorbents is a highly promising candidate for wastewater treatment with heavy metal ions.
The aqueous Al‐ion battery has achieved great progress in recent years. It now shows comparable performance to that of even non‐aqueous Al‐ion batteries. However, it also shows relatively low energy output and there is limited general understanding of the mechanism behind this restriction to its practical application. Thus, the development of a high‐performance cathode material is in great demand. Herein, a high‐capacity single‐walled carbon nanotube (SWCNT) is developed as a cathode for the water‐in‐salt electrolyte‐based aqueous Al‐ion battery, which provides an ultra‐high specific capacity of 790 mAh g–1 (based on the mass of SWCNT) at a high current density of 5 A g–1 even after 1000 cycles. Moreover, the SWCNT/Al battery shows a complicated multi‐ion intercalation mechanism, where AlCl4–, Cl–, Al3+, and H+ can function at the same time, improving the battery output. Beyond recently revealed H+ and metal ion co‐intercalation, the Cl‐assisted intercalation of Al3+ ions mechanism is also studied by experimental characterization and modeling for the first time, which significantly boosts the Al3+ storage capacity. This multi‐ion intercalation mechanism combines the high‐voltage anion deintercalation and the high‐capacity cation intercalation, and thus, benefits the development and application of high‐energy Al‐ion batteries in the future. A high‐capacity single‐walled carbon nanotube is utilized as the cathode with a “water‐in‐salt” electrolyte for an aqueous Al‐ion battery. It is found that the carbon nanotube cathode exhibits superior energy density, which can be attributed to the multi‐ion intercalation mechanism, that is, the high‐voltage deintercalation of AlCl4– and the high‐capacity intercalation of Al3+ cations.
The practical application of rechargeable aqueous zinc batteries is impeded by dendrite growth, especially at high areal capacities and high current densities. Here, this challenge is addressed by proposing zinc perfluoro(2‐ethoxyethane)sulfonic (Zn(PES)2) as a zinc battery electrolyte. This new amphipathic zinc salt, with a hydrophobic perfluorinated tail, can form an anode protecting layer, in situ, with a biomimetic lipid‐bilayer structure. The layer limits the anode contact with free H2O and offers fast Zn2+ transport pathways, thereby effectively suppressing dendrite growth while maintaining high rate capability. A stable, Zn2+‐conductive fluorinated solid electrolyte interphase (SEI) is also formed, further enhancing zinc reversibility. The electrolyte enables unprecedented cycling stability with dendrite‐free zinc plating/stripping over 1600 h at 1 mA cm−2 at 2 mAh cm−2, and over 380 h under an even harsher condition of 2.5 mA cm−2 and 5 mAh cm−2. Full cell tests with a high‐loading VS2 cathode demonstrate good capacity retention of 78% after 1000 cycles at 1.5 mA cm−2. The idea of in situ formation of a biomimetic lipid‐bilayer anode protecting layer and fluorinated SEI opens a new route for engineering the electrode–electrolyte interface toward next‐generation aqueous zinc batteries with long lifetime and high areal capacities. New aqueous electrolytes based on an amphipathic zinc salt (Zn(PES)2) are developed to enable dendrite‐free zinc‐metal batteries with high areal capacities and high current densities through in situ formation of a biomimetic lipid‐bilayer protecting layer and fluorinated solid electrolyte interphase on zinc electrode surfaces. This study opens a new route for developing high‐performance zinc battery electrolytes.
A 2,1,3-benzothiadiazole-based photosensitiser has been successfully incorporated into a commercially available 3D printing resin and utilised to fabricate inherently photocatalytic flow reactor components. The freedom of design provided by additive manufacturing enabled the production of photoactive monolith structures with intricate architectures, imparting functionality for heterogeneous photocatalysis and interesting manipulation of fluid dynamics within a fixed bed reactor column. The resultant monoliths were applied and validated in the photosensitisation of singlet oxygen in aqueous media, under continuous flow conditions and visible light irradiation (420 nm). The photo-generated singlet oxygen cleanly converted furoic acid to the γ-lactone, 5-hydroxy-2(5H)-furanone, with a peak space-time yield of 2.34 mmol m−2 h−1 achieved using the Voronoi monolith. [Display omitted] •Direct stereolithographic fabrication of monolithic heterogeneous photocatalysts.•Compatible with commercial resins and stereolithographic apparatus printers.•Rapid access to non-commercially available structures which improved gas-liquid mixing in flow.•Successful application as heterogeneous photosensitisers demonstrated via generation of 1O2 under visible light irradiation.•1O2 mediated oxidation of aqueous furoic acid to 5-hydroxy-2(5H)-furanone achieved in flow.
Microfluidic fuel cell (MFC) is a new type of fuel cell which utilizes two laminar flows as electrolyte for both reactant delivery and ionic conduction purposes. According to the flow configuration, two MFC designs, that is, the co-flow cell and the counter-flow cell, have been proposed in the literature, but the specific merits and demerits between them have not been well understood yet. Therefore, in this work both experimental and numerical comparative studies are conducted on this issue. It is found that the counter-flow MFC encounters an inherent drawback on its power output because the outer part of its electrodes contributes much less than the inner part due to the longer ionic transport path. In conclusion, short and wide electrodes are more appropriate for counter-flow MFCs rather than the conventional long and narrow ones. Nevertheless, the counter-flow MFC does exhibit great advantage on low flow rate tolerance, leading to much improved fuel utilization and energy density at the same time. Considering this huge superiority for real applications, structural optimization of the counter-flow MFC is further conducted by reducing the electrode distance and discarding the outer part of the electrodes. The optimized counter-flow cell performance is successfully improved to the same level with its co-flow counterpart. (C) 2017 Elsevier Ltd. All rights reserved.
•An improved agglomerate sub-model of catalyst layer is developed.•Oxygen local transport resistance is clarified with three individual parts.•Effects of Pt loading and Pt particle dispersion are considered.•The CL agglomerate sub-model is incorporated into 3D PEM fuel cell model.•Fine channel geometry helps improve gas distribution and cell performance. An improved agglomerate sub-model of catalyst layer (CL) involving actual agglomerate size and oxygen local transport characteristics is developed and incorporated into a three-dimensional (3D) multi-phase model of proton exchange membrane (PEM) fuel cell. This makes it capable to consider the effect of platinum (Pt) loading on oxygen transport and fuel cell performance more accurately. Oxygen local transport resistance near the catalyst surface is divided into three parts caused by liquid water blockage, ionomer coverage and Pt/carbon agglomeration, respectively. The resistances caused by ionomer coverage and Pt/carbon agglomeration are two major sources of oxygen local transport resistance. They have opposite variation trends as Pt loading changes. However, the ionomer resistance increases dramatically when Pt loading is lower than 0.1 mg cm−2 because of the much harder transport process through a relatively heavier ionomer coating. The simulation results agree with the experimental data reasonably under different cathode Pt loadings (from 0.3 to 0.025 mg cm−2), for both polarization curves and local transport resistance. In addition, a transport dominance parameter is defined to judge whether the concentration loss predominates the electrochemical reaction. A value greater than 10% can be seen as a symbol of local oxygen starvation. Using this model, fine channel geometry with extremely small channel and rib widths is investigated, and the highest net output power in this study is corresponding to 0.2 and 0.6 mm for channel (rib) width and height.
Aluminum storage in rutile-based TiO2 nanoparticles was for the first time investigated. Electrochemical characteristics of ruffle based TiO2 nanoparticles as an electrode for aluminum-ion batteries were studied using cyclic voltammetry, chronopotentiometry and electrochemical impedance spectroscopy. The first discharge capacity of 29.4 mAh.g(-1) was achieved, and the value remains 22.6 mAh.g(-1) after 50 cycles. The highest coulombic efficiency achieved at 89.8%. (C) 2019 The Authors. Published by Elsevier Ltd.
Proton exchange membrane fuel cell (PEMFC) provides a promising future low carbon automotive powertrain solution. The catalyst layer (CL) is its core component which directly influences the output performance. PEMFC performance can be greatly improved by the effective optimization of CL composition. This work demonstrates a deep optimization of CL composition for improving the PEMFC performance, including the platinum (Pt) loading, Pt percentage of carbon-supported Pt and ionomer to carbon ratio of the anode and the cathode,. The simulation results by a PEMFC three-dimensional (3D) computation fluid dynamics (CFD) model coupled with the CL agglomerate model is used to train the artificial neural network (ANN) which can efficiently predict the current density under different CL composition. Squared correlation coefficient (R-square) and mean percentage error in the training set and validation set are 0.9867, 0.2635% and 0.9543, 1.1275%, respectively. It illustrates that the well-trained ANN has a comparable accuracy with the physical model. Then, the ANN is utilized as the fitness function in the genetic algorithm (GA) to search the optimal CL composition for maximizing the current density. For verification, the optimal solution of CL composition is returned to the physical model and the comparison between the ANN predicted current density and the physical model simulated current density is provided. The percentage error is only 2.418% which can illustrate the validity of this work.
Summary Concentrated photovoltaic cell (CPV) is a solar energy harvesting device that converts solar energy into electrical energy. However, the performance and efficiency of the CPV are heavily dependent on the temperature. Besides, nonuniformity of temperature distribution on the CPV will lead to thermal aging and affects the cycle life. Hence, an effective cooling system is required to remove excess heat generated to ensure that the CPV operates at optimum operating temperature with minimum variation of temperature. Metal foam is a new class of material that possesses huge potential for thermal management. In this study, a functionally graded metal foam is proposed for the CPV thermal management system. Computational thermal fluid dynamic analysis is conducted to investigate the effect of porosity and pore density on the flow field and thermal performance of the aluminum foam heat sink. The investigation results revealed that 10 PPI functionally graded aluminum foam heat sink with two stages of porosity gradient 0.794 and 0.682 produced the lowest pressure drop and highest thermal performance. Temperature difference of 3.9°C was achieved for a solar cell with total heat generation of 900 W under water mass flow rate of 20 gs−1. Novelty Functionally graded metal foam heat sink has been designed for CPV. A CFD model has been developed to investigate the performance of the functionally grade metal foam. Optimized pore density and porosity of the functionally graded metal foam have been identified.
Hybrid renewable energy systems that combine heat and electricity generation is an achievable option for remote areas where grid is uneconomical to extend. In this study, a renewable based system was designed to satisfy the electrical and thermal demands of a remote household in an off-grid Greek island. A hybrid DC system consisted of a combination of photovoltaic modules, wind turbine, electrolyzer-hydrogen tank, fuel cell and batteries were analysed using HOMER Pro software. Based on the optimal obtained system, it is found that such a system can satisfy both electrical and thermal load demand throughout the year in a reliable manner. (C) 2019 The Authors. Published by Elsevier Ltd.
Effective use of natural materials to fabricate porous carbonaceous structures for anodes of microbial fuel cells (MFCs) has a high potential for substantial cost reduction in MFC. In this study, three kinds of plant materials, i.e. king mushroom, wild mushroom and corn stem, were investigated for fabrication of conductive electrode materials by simple carbonization procedures. Structure–reactivity relationships of these electrodes were systematically studied with electrochemical redox probe ([Fe(CN)6]3−/4−) and biofilm electroactivity. The electrochemical and bioelectrochemical accessibilities of the carbonized electrodes were evaluated by impedance, cyclic voltammetry and chronoamperometry techniques in order to study the electron transfer rate (Kapp), charge transfer resistances, oxidative current density and bioelectroactive moieties. The results showed that the electron transfer resistance (Rct) was 94Ω for carbonized corn stem electrode with an electron transfer rate (Kapp) of 3.44×10−2cms−1 for Fe2+/Fe3+ redox probe. Higher bioelectroactivity (9.29×10−8molcm−2) was found from biofilm on carbonized corn stem (Rbiofilm, 45Ω) with an electron transfer rate (bacteria-anode) of 63×10−5cms−1. The maximum bioelectrocatalytic current (imax) of 3.12mAcm−2 was obtained on carbon electrode derived from corn stem. That is 8 times higher than plain graphite electrode. The porous architecture, high electron transfer rate and high electroactive biofilm growth are attributes that qualify natural-material carbon anodes as low-cost alternative for MFC.
Microfluidic membraneless fuel cell (MFC) is a promising fuel cell type due to its simple structure without the need of proton conducting membrane. However, the common disadvantage is the low fuel utilization. Previous works have shown that adopting a conventional method to increase the fuel utilization would cause a low power density. This study shows that the use of hydrodynamic focusing technology can overcome the trade-off problem between the fuel utilization and the current density. A numerical model has been developed to simulate the MFC operation with the fuel stream being hydrodynamically focused by a buffer stream. The results indicate that both fuel utilization and current density can be increased by properly adjusting the buffer flow rate to enhance the flow focusing. The optimal performance is achieved when the buffer-to-fuel flow rate ratio is around 25. Moreover, high fuel flow rate and shallow channel shape have proven beneficial to the cell performance with the use of hydrodynamic focusing technology. It is predicted that a MFC with a current density above 100 mA cm(-2) is capable of achieving fuel utilization up to 50%, which is considerably higher than the previously reported value of 5-8%. Copyright (C) 2011, Hydrogen Energy Publications, LLC. Published by Elsevier Ltd. All rights reserved.
The vision of producing sustainable and renewable bioenergy is to contribute reducing the current global warming impact. It is well known that microorganisms can produce fuels, such as ethanol, butanol, methane, and hydrogen. Alternatively, microbes can produce electricity by means of microbial fuel cell (MFC). The recent development in energy biology has demonstrated the potential of MFC for commercial applications in converting renewable biomass and organic waste into electricity. The biomass sources are highly desirable because they are “carbon‐neutral.” Electrical energy can be extracted from organic waste matter and renewable biomass by microbial degradation. The major challenge of using microbial fuel cell is to increase power density for most of the envisaged applications, such as power monitoring electronic devices, light source of battery charger in off‐grid areas. A wide scope lies ahead in the scaling up of MFC for large‐scale conversion of organic waste and biomass into electricity for powering vehicles, mobile electronic devices or buildings. The use of waste organic from biomass is environment‐friendly and regarded as a renewable energy source. This article gives an overview of microbial fuel cell that covers the bioelectrochemical mechanisms, system and components, characterization, performance, and applications.
[Display omitted] •Low loading of BTZ-Py polymer loaded GA (CMPGA) was successfully prepared.•The synthesis method was green and binder-free under mild conditions.•The CMPGA showed synergistic effect between BTZ-Py polymer and graphene sheets.•CMPGA2 (5 wt% BTZ-Py polymer) had the best methyl orange degradation activity.•Overall stability of CMPGA2 was good after 3 cycles of methyl orange degradation. This paper reports a new class of efficient, green and metal-free visible-light photocatalyst made from graphene aerogel (GA) doped with a conjugated porous polymer (CMPs). Hence, we report the synthesis of a benzothiadiazole (BTZ)-based CMP loaded into GA via a one-step hydrothermal reaction between 2D graphene oxide (GO) and the CMP, performed through a green process and under mild conditions. The as-prepared GA showed a bathochromic shift in the UV–vis diffraction reflectance spectroscopy (DRS) absorption edge to 628.5 nm, demonstrating its ability to absorb light in the visible region. SEM, TEM, XPS, EDX mapping results further showed the successful loading of the BTZ-based CMP in the GA array. The synthesized GA was used as a 3D structured photocatalyst for the visible-light-driven photodecomposition of methyl orange (MO) with an efficiency of 89.2% (5 wt% CMP). When compared to that of the pure CMP (86.9%), a comparable yet small increase in the efficiency was observed. This is due to a synergistic effect between GO and loaded polymer in GA array upon the formation of CMPGA hybrid structure via chemical interaction between BTZ-Py and GO throughout the mild hydrothermal reaction, and the enhanced photocatalytic activity exhibited from 1 mg equivalent polymer in the CMPGA2 hybrid when compared to the 20 mg pure polymer. Upon repeated use, the depreciation in photocatalytic activity was low with a
The microfluidic technology for function microsphere synthesis has high control precision. However, the throughput is too low for industrial scale-up applications. Current scale-up design focuses on a multi-channel in 2D, in which the distribution uniformity parameter δ increases linearly, resulting in the deterioration of the flow distribution performance. The 3D modular scale-up strategy could greatly alleviate this problem, but no design principles have been developed yet. For the first time, this paper establishes the microfluidic 3D scale-up design criteria. Based on the modular design concept, the design method of 2D and 3D throughput scale-up parameters N and M, distribution uniformity parameters δ and β, and microchannel design parameter KR were proposed. The equivalent resistance coefficient was defined, and the influence of different parameters on a 2D array and 3D stack was analyzed. Furthermore, the error correction method was studied. It was found that the two-stage scale-up process contradicted each other. A good scale-up performance of one stage led to the limitation of another stage. Increasing the resistance of each channel Ru could both increase the two-stage scale-up performance, which was an important factor. A single-module scale-up system with 8 channels in a single array and 10 arrays in a vertical stack, which had 80 channels in total, was designed and fabricated based on the proposed design criteria for generating Chitosan/TiO2 composite microspheres. The average particle size was 539.65 µm and CV value was about 3.59%. The throughput was 480 ml h−1, which effectively increased the throughput scale and the product quality.
A multiscale-designed optofluidic reactor is demonstrated in this work, featuring an overall reaction rate constant of 1.32 s(-1) for photocatalytic decolourization of methylene blue, which is an order of magnitude higher as compared to literature records. A novel performance-enhancement mechanism of microscale in situ photo-galvanic acceleration was found to be the main reason for the superior optofluidic performance in the photocatalytic degradation of dyes as a model reaction.
Because of increasing numbers of oil spill accidents, considerable attention has been paid to the development of effective and inexpensive oil sorbents. Carbonized cotton fibers (CCFs) with a hollow tubular structure were successfully prepared by treating natural cotton in a N-2 atmosphere and used as high-capacity oil sorbents. The material properties of the as-prepared CCFs were investigated by scanning electron microscopy, X-ray diffraction, contact-angle measurements, and N-2 adsorption-desorption. Maximum oil sorption tests indicated that CCFs-400 showed the highest oil adsorption capacity and could absorb up to 32-77 times its own weight in pure oils and organic solvents, suggesting an increase of 27-126% compared with the capacity of cotton fibers. Also, repeatability, selectivity, and floating-ability tests suggested that CCFs-400 showed much better performance than cotton fibers in pure oil medium or water oil mixtures. Owing to their multiscale porous structures, superhydrophobicity, and superoleophilicity, the CCFs demonstrated great potential as low-cost and effective sorbents in oil adsorption.
•Open source solid oxide fuel cell models were developed at cell and pore scales.•Highly resolved real digital microstructures of anode were employed.•Two models were validated against both experiments and various numerical models.•Representative volume element with respect to current density was determined.•Synthetic and real digital electrode microstructure were compared. Solid oxide fuel cells are high-efficiency renewable energy devices and considered one of the most promising net-zero carbon energy technologies. Numerical modelling is a powerful tool for the virtual design and optimisation of the next-generation solid oxide fuel cells but needs to tackle issues for incorporating the multi-scale character of the cell and further improving the accuracy and computational efficiency. While most of solid oxide fuel cell models were developed based on closed source platforms which limit the freedom of customisation in numerical discretization schemes and community participation. Here, an open source multi-physics and multiscale platform for advanced SOFC simulations consisting of both cell- and pore-scale performance models was developed using OpenFOAM. The modelling aspects are elucidated in detail, involving the coupling of various physical equations and the implementation of the pore-scale electrode in the performance model. The entire platform was carefully validated against experimental data and the other numerical models which were implemented in commercial software ANSYS Fluent and based on the lattice Boltzmann method. The cell-scale model is subsequently employed to study the effects of different fuels, i.e., pure hydrogen and different ratios of pre-reformed methane gas under various operating temperatures. It is found that the cell-scale model reasonably predicts the effects of these parameters on the cell performance, aligning well with the Fluent model. This study further identified the size of representative element volume with respect to the current density for the anode via the pore-scale model where the realistic microstructures reconstructed by a Xe plasma focused ion beam–scanning electron microscopy are employed as computational domains. It is found that a volume element size of 1243 voxels is sufficient to yield the representative current density of the whole. All these numerical investigations show that OpenFOAM is a potential multi-physics and multi-scale computational platform that is capable of accurately predicting both cell-scale and pore-scale performance and spatial information of solid oxide fuel cells. The developed models are also made public in GitHub to inspire community to further develop around it.
•A comprehensive PEMFC system model integrating all subsystems is developed.•All sub-models are rigorously validated to guarantee the system model accuracy.•Membrane dehydration is observed when the stack is operated at 70 °C and 80 °C.•Co-current flow pattern contributes to higher water utilization of whole system.•Increasing humidifier area results in much slower humidification responses. A comprehensive proton exchange membrane fuel cell (PEMFC) system model is developed, including a pseudo two-dimensional transient multiphase stack model, a one-dimensional transient multiphase membrane humidifier model, a one-dimensional electrochemical hydrogen pump model, an air compressor model with proportion-integral-derivative control and a ribbon-tubular fin radiator model. All sub-models have been rigorously validated against experimental data to guarantee the system model accuracy. The effects of stack operating temperature, gas flow pattern and humidifier structural design are investigated to cast insights into the interaction among stack and auxiliary subsystems. The results indicate that the stack is successfully maintained at required operating temperatures (60 °C, 70 °C, 80 °C) with help of the radiator when the whole system starts from ambient temperature (25 °C). However, the stack is likely to suffer from membrane dehydration when operated at 70 °C, and the problem becomes more severe at 80 °C, causing significant performance deterioration. The water and temperature distribution inside the system are further demonstrated. The co-current flow pattern contributes to better water utilization of the whole system which may lead to higher output performances. But the counter-current flow pattern has positive effects on parameter distribution uniformity inside fuel cell, which is beneficial for the stack durability. As regards the membrane dehydration, it is found that optimizing membrane humidifier area does not fundamentally solve the problem. Increasing humidifier area contributes to higher water vapor transfer rate, however, it results in much slower humidification responses.
CO2 can be converted to useful fuels by electrochemical processes. As an effective strategy to address greenhouse effect and energy storage shortage, electrochemical reduction of CO2 still needs major improvements on its efficiency and reactivity. Microfluidics provides the possibility to enhance the electrochemical performance, but few studies have focused on the virtual interface. This work demonstrates a dual electrolyte microfluidic reactor (DEMR) that improves the thermodynamic property and raises the electrochemical performance based on a laminar flow membrane-less architecture. Freed from hindrances of a membrane structure and thermodynamic limitations, DEMR could bring in 6 times higher reactivity and draws electrode potentials closer to the equilibrium status (corresponded to less electrode overpotentials). The cathode potential was reduced from -2.1 V to -0.82 V and the anode potential dropped from 1.7 V to 1 V. During the conversion of CO2, the peak Faradaic and energetic efficiencies were recorded as high as 95.6% at 143 mA/cm(2) and 48.5% at 62 mA/cm(2), respectively, and hence, facilitating future potential for larger-scale applications. (C) 2016 Elsevier Ltd. All rights reserved.
•The performance limiting factors of droplet microfluidic extraction are identified.•The mechanisms of microfluidic separation intensification are unveiled.•Separation performance can be controlled in droplet microfluidic extraction. Droplet-based microfluidic extraction is a promising way for effective lanthanides extraction due to its outstanding mass transfer performance. The separation process can be greatly enhanced with the droplet-based microfluidic extraction technique. However, the interactions between mass transfer, microfluidic dynamics and extraction kinetics are still unclear, which has hindered further manipulation on microfluidic extraction to boost extraction performance. In this study, the mechanisms of microfluidic droplet-based extraction and separation intensification of lanthanides are for the first time unveiled by using a numerical simulation model. The limiting factors for the performance of droplet-based microfluidic extraction are identified through a model-based parametric analysis. The numerical analyses provide a comprehensive understanding of droplet-based microfluidic extraction systems and offer operation and optimization guidelines for future research in this area.
A promising strategy to mitigate both energy shortage and global warming is the conversion of CO2 into chemicals that can be used as fuels (chemical fuels) by utilizing renewable energy sources. Up to date, solar-driven CO2 reduction has been achieved with photochemical (PC) and photoelectrochemical (PEC) systems or electrochemical cells combined with a photovoltaic system (PV-EC). This study is intended to compare and highlight the state-of-the-art PEC systems for CO2 reduction and show the limitation factors that still hinder their widespread utilization. The review starts with a description of semiconducting photocatalyst properties and fundamental understanding of PEC CO2 reduction process. Then, the most significant performance metrics used for evaluation of PEC systems are explained in details. In addition, recent progress in PEC CO2 reduction systems is summarized and classified in different categories according to the chemical product. Different strategies such as doping, combination of two or more semiconductors, synthesis of nanostructured materials, passivation layers and co-catalysts that enhance light absorption, chemical stability, charge transfer and reduce ohmic losses and overpotentials of photoactive materials are reviewed. Besides the improvement of photocatalysts, research progress on the front of PEC reactor design, combined with the development of advanced modelling tools and characterization techniques are expected to bring PEC CO2 reduction a step closer to commercialization.
Microfluidic fuel cell is a promising power source in many applications, such as portable electronic devices. In this work, a novel and unique scale-up approach is developed to increase the capability of the microfluidic aluminum-air fuel cell, while maintaining the co-laminar flow characteristic at the same time. In the scaled-up cell, the crossover of different electrolyte streams was well controlled and a higher cell output was achieved. With a four-fold increase in the electrode area, the maximum current density could be maintained over 75%. Impedance spectroscopy study on full aluminum-air cells was conducted for the first time, and employed to analyze the cell properties during their scaling up process. (C) 2016 The Electrochemical Society. All rights reserved.
This paper reports a mathematical model for the calculation of various losses in a dual electrolyte membraneless electrolytic cell (DEME) for CO2 to formic acid conversion. The microfluidic characteristics of the cell were explored. Based on the electrochemical equilibrium states, major limiting factors, including mass transfer constraints, kinetic losses, and overpotentials, were considered. In particular, the acid-base interface and the neutralization losses therein were identified. We also quantified the electrical resistance losses on electrodes and within the micro-channels. Computational results were validated against previous experimental data. To our best knowledge, this is the first model studying the dual electrolyte arrangement and the associated losses, which can be used to develop future parametric optimization strategies.
To better understand the mass transfer and provide possible performance improvement methods for the microfluidic devices relying on the two-layer laminar flow, a computational fluid dynamics simulation is performed to investigate the phenomenon of a two-layer laminar stream passing through a curved microchannel. The results show that due to the density difference between the two fluids, asymmetric pair of Dean vortices are formed as the fluids pass through the curve. Due to the inter-stream convection, the denser fluid intrudes into the less-dense one resulting in a Gamma sharp layer-interface. Furthermore, the parametric analysis helps identify ways to minimize the inter-stream mass transfer, such as increasing the density ratio, as well as reducing the Re number and aspect ratio. The study concludes that the microchannel curve can be properly designed to obtain a clear interface between two streams to fulfill the basic requirement of microfluidic devices. (C) 2011 Elsevier B.V. All rights reserved.
Desired electrochemical reaction and mass transport rates vary in the operation of PEM fuel cells due to the inhomogeneous spatial distribution of reactants and products. A segmented fuel cell unit was manufactured and a comprehensive model was developed to study the effect of the graded distributions of platinum loading and operating temperature, to simultaneously save the usage of platinum, improve the cell performance and maintain the homogeneity of current density. The increase of temperature towards the cathode outlet improved the reaction kinetics and reduced the liquid water content along the gas flow direction, which decreased the required platinum loading. A large temperature gradient may lead to membrane/ionomer dehydration and oxygen starvation near the cathode outlet due to the increase in the saturation pressure of vapor and the dilution of the increased vapor content. A systematical design of the gradients of platinum loading and temperature achieved an improved cell performance and saved the usage of Pt-based catalysts without worsening the homogeneity of current density.
In this study, a facile hydrothermal method was successfully developed to synthesize boron self-doped TiO2 nanosheets directly from TiB2 powders. XRD and TEM results suggested that the as-prepared TiO2 were pure anatase phase and showed a sheet morphology with reactive (001) facets. XPS analysis revealed the presence of boron as dopants by substituting oxygen atom in TiO2 nanosheets. The effect of HBF4 on TiO2 synthesis was also investigated. Photocatalytic disinfection experimental study implied that boron self-doped TiO2 exhibited better performance than P25 under visible light irradiation. The enhanced photocatalytic effect was ascribed to the nanosheet morphology with reactive (001) facets and self-doping of boron in TiO2. •Boron self-doped TiO2 nanosheets derived from TiB2 were successfully achieved.•TiB2 was the source of boron dopants and TiO2 precursor.•Boron self-doped TiO2 nanosheets showed better photocatalytic activity than P25.•The enhanced photocatalytic activity was due to the reactive facets and self-doping of boron.
Liquid fuel cells operated at room-temperature are promising candidates for the next-generation of power sources for microelectronic devices. Enabling high-concentrated or even neat fuel operation of fuel cells, which is currently limited by adverse fuel crossover through membrane, could significantly improve the cell current density, reversible potential and energy density. Herein, microfluidic tools are proposed to solve the crossover problem. A three-dimensional numerical model for air-breathing microfluidic fuel cell (MFC) fed with concentrated formic acid is developed. The model couples CFD with electrochemical kinetics to account for the complex interactions inside the cell. Maxwell-Stefan equations are used to describe the nonlinear behaviors in the electrolyte. Based on the model analysis, the feasibility of concentrated fuel operation has been proved. Nonlinear diffusion characteristics, mass transport and performance of MFC cell are analyzed. (C) 2013 Elsevier Ltd. All rights reserved.
The promise and challenge of electrochemical mitigation of CO2 calls for innovations on both catalyst and reactor levels. In this work, enabled by our high-performance and earth abundant CO2 electroreduction catalyst materials, we developed alkaline microflow electrolytic cells for energy-efficient, selective, fast, and durable CO2 conversion to CO and HCOO-. With a cobalt phthalocyanine-based cathode catalyst, the CO-selective cell starts to operate at a 0.26 V overpotential and reaches a Faradaic efficiency of 94% and a partial current density of 31 mA/cm(2) at a 0.56 V overpotential. With a SnO2-based cathode catalyst, the HCOO--selective cell starts to operate at a 0.76 V overpotential and reaches a Faradaic efficiency of 82% and a partial current density of 113 mA/cm(2) at a 1.36 V overpotential. In contrast to previous studies, we found that the overpotential reduction from using the alkaline electrolyte is mostly contributed by a pH gradient near the cathode surface.
Emerging emission-to-liquid (eTL) technologies that produce liquid fuels from CO2 are a possible solution for both the global issues of greenhouse gas emissions and fossil fuel depletion. Among those technologies, CO2 hydrogenation and high-temperature CO2 electrolysis are two promising options suitable for large-scale applications. In this study, two CO2-to-methanol conversion processes, i.e., production of methanol by CO2 hydrogenation and production of methanol based on high-temperature CO2 electrolysis, are simulated using Aspen HYSYS. With Aspen Energy Analyzer, heat exchanger networks are optimized and minimal energy requirements are determined for the two different processes. The two processes are compared in terms of energy requirement and climate impact. It is found that the methanol production based on CO2 electrolysis has an energy efficiency of 41%, almost double that of the CO2 hydrogenation process provided that the required hydrogen is sourced from water electrolysis. The hydrogenation process produces more CO2 when fossil fuel energy sources are used, but can result in more negative CO2 emissions with renewable energies. The study reveals that both of the eTL processes can outperform the conventional fossil-fuel-based methanol production process in climate impacts as long as the renewable energy sources are implemented. (C) 2015 Elsevier Ltd. All rights reserved.
•A hybrid model is developed for SOFCs combining MPS and deep learning algorithm.•A detailed study is conducted considering the fuel complexity and thermal effects.•The deep learning algorithm is well trained for prediction with an accuracy > 99%.•Online optimisation of SOFC performance is achieved using the genetic algorithm.•An efficiency > 65% is ensured under safe operation in real-time optimisation. The use of solid oxide fuel cells (SOFCs) is a promising approach towards achieving sustainable electricity production from fuel. The utilisation of the hydrocarbons and biomass in SOFCs is particularly attractive owing to their wide distribution, high energy density, and low price. The long-term operation of SOFCs using such fuels remains difficult owing to a lack of an effective diagnosis and optimisation system, which requires not only a precise analysis but also a fast response. In this study, we developed a hybrid model for an on-line analysis of SOFCs at the cell level. The model combines a multi-physics simulation (MPS) and deep learning, overcoming the complexity of MPS for a model-based control system, and reducing the cost of building a database (compared with the experiments) for the training of a deep neural network. The maximum temperature gradient and heat generation are two target parameters for an efficient operation of SOFCs. The results show that a precise prediction can be achieved from a trained AI algorithm, in which the relative error between the MPS and AI models is less than 1%. Moreover, an online optimisation is realised using a genetic algorithm, achieving the maximum power density within the limitations of the temperature gradient and operating conditions. This method can also be applied to the prediction and optimisation of other non-liner, dynamic systems. The schematic of an SOFC with complex fuel composition and the workflow for the development of combined multi-physics model and AI simulation. [Display omitted]
► We model CO2 reduction in microfluidic cells. ► Transport phenomena on cathode side are studied. ► Limiting factors to the cell performance are identified. ► Parametric study is performed towards an optimal cell operation. A numerical model for a microfluidic electrolytic cell (MEC) for CO2 utilization and fuel production is developed and validated against experimental data in literature. Mass transfer and electrochemical characteristics at the cathode of the MEC are studied. Limiting factors to the cell performance are found to be (i) low diffusivity of CO2 in the porous electrode, (ii) competing hydrogen evolution reaction (HER) at the cathode and (iii) dilution effect of hydrogen. Parametric effects are also studied to find strategies to optimize the cell performance.
Using biogas for hydrogen production via autothermal reforming (ATR) can potentially increase the energy conversion efficiency and correspondingly reduce environmental impact. The present study aimed to investigate the performance and characteristics of biogas ATR. A two-dimensional numerical model was developed based on the integration of computational fluid dynamics (CFD) and chemical kinetics. The mass transport, chemical reactions and heat transfer can be analyzed simultaneously in the porous domain. The results show that the presence of CO2 in the feedstock will reduce the performance of the biogas ATR. The effects of operating and feeding conditions were examined and the optimal conditions were identified. operating the reformer with the steam-to-CH4 ratio (S/CH4) and air-to-CH4 ratio (A/CH4) equal to 0.5 and 2, respectively, can achieve high H-2 concentration, while operation with S/CH4 and A/CH4 equal to 4.5 and 2, respectively, can achieve high energy efficiency. The results also show that using either H-2 or O-2 membrane in the reformer can enhance the biogas autothermal reforming performance by producing high concentration of H-2 (40-65%) and solving the harmful hot spot problems. (C) 2009 Professor T. Nejat Veziroglu. Published by Elsevier Ltd. All rights reserved.
This article provides an up-to-date highly comprehensive overview (594 references) on the state of the art of the synthesis and design of macroporous materials using microfluidics and their applications in different fields.
Air-breathing reversible microfluidic fuel cell (RMFC) provides flexibility to choose either acid or alkaline medium for the bifunctional oxygen electrode. A numerical model has been developed and validated to predict the performance of an air-breathing RMFC. Half-cell J-V characteristics of the RMFC using different pH media for the oxygen electrode are compared. The model results suggest that when the RMFC is operated in fuel cell (FC) mode, alkaline medium is preferred for the oxygen electrode, and when operated in electrolysis-cell (EC) mode, acid medium is preferred. By further analyzing the round-trip energy efficiency and major potential loss of the half-cell, it is found that adopting acid medium for oxygen electrode can maximize the overall charging/discharging cycle efficiency and performance of RMFC, due to much lower activation overpotential in the EC mode. Heat and mass transport characteristics of the half-cell are also investigated. It is found that the flowing electrolyte can efficiently remove the heat generated by various sources in the RMFC, leading to the mass convection in the oxygen electrode and surrounding environment solely driven by concentration gradient. Due to the presence of water vapor as the reaction product, FC mode operation in acid medium yields the most intensive breathing process of the oxygen electrode. The results provide implications to further optimizations of RMFC. Copyright (c) 2011, Hydrogen Energy Publications, LLC. Published by Elsevier Ltd. All rights reserved.
Sludge conditioning by the Fenton process can effectively reduce sludge water content. The Fenton process has ideal dewaterability at different initial pH levels. However, the difference in the initial pH levels can lead to variations in conditioner dosage, resulting in different environmental impacts and economic pressures. This study provides insights into the role of the life cycle linked response surface methodology (LC-RSM) framework in revealing optimal solutions with both environmental and economic performance in different combinations of conditioners. Response surface methodology (RSM) was used to construct a model of sludge dewatering by the Fenton process, and life cycle assessment and life cycle cost were used to quantify the environmental and economic performance of all conditioning solutions in the model. The LC-RSM optimization solution was 6%–68% and 15%–70% lower than the RSM recommended solution in terms of environmental impacts and economic costs, respectively. For specific impact categories, such as global warming potential, the LC-RSM optimization solution was 19%–56% lower than the RSM recommended solution. In addition, the total environmental impact of the different conditioner combinations ranged between 4.1 and 7.8 Pt and 3.2–6.7 Pt at 53% and 58% of the sludge target water content, respectively. Surprisingly, the environmental impact of the solution with superior dewatering performance (53%) was lower than that of the solution with poor dewatering performance (58%) in many cases. The above results show that the LC-RSM optimization solution has better environmental and economic performance compared to RSM. In addition, the environmental impacts and economic costs of different solutions with the same water content target vary widely, which may lead to comparing sludge conditioning procedures with different targets in an unfair environment. Therefore, a unified platform for comparison is needed, and LC-RSM can provide support. This study can assist decision-makers in identifying the best solution for both environmental and economic performance in a multivariate system and stimulate further discussion on how to compare different processes on a more uniform platform.
An innovative coating-by-parts (CBP) sampling method is proposed to improve the experimental study of water gas shift (WGS) systems used in systems in packed-bed and monolithic reactors. The CBP method enables detailed measurements of chemical species concentrations and operating conditions inside a reactor. Chemical kinetics-coupled CFD model has been set up for simulation to evaluate the performance of CBP. The model was first validated by the experimental data and subsequently run for multiple cases to simulate the use of CBP. The results show that the CBP method has an acceptable accuracy in packed-bed reactor and monolithic reactors. Meanwhile, another commonly used experimental method, namely, the central line sampling method, is found to achieve a high accuracy in the monolithic reactor, but a lower accuracy in the packed-bed reactor with low inlet velocity and packed by larger pellets. Copyright (C) 2010 John Wiley & Sons, Ltd.
•Thermodynamics of aluminum intercalation in TiO2(B) is predicted.•The specific capacity with volume change is 755.05 mA h g−1.•It doubles the theoretical value of lithium storage in TiO2(B).•TiO2(B) can offer new opportunities for aluminum-ion batteries. Aluminum-ion batteries have emerged as a potential alternative to lithium-ion batteries by offering advantages such as abundant aluminum resources, low costs and good safety. Exploring suitable electrode materials lies at the heart of the development of aluminum-ion batteries. Relying on first-principles density functional theory, this study predicts the thermodynamics of aluminum intercalation in TiO2(B) as a promising aluminum storage material. Three sites are identified to be the preferential locations for aluminum within the TiO2(B) structure, and the stable intercalation site is found to prefer 5-fold coordinated to oxygen atoms with a slightly off-center position. The supercell volume change associated with aluminum intercalation is < 10%, and the thermodynamically maximum achievable Al/Ti ratios are respectively 0.6562 and 0.75 without and with considering this volume change. The corresponding specific capacities without and with volume change are calculated to be 660.62 mA h g−1 and 755.05 mA h g−1, doubling the theoretical value of lithium storage in TiO2(B). As expected, aluminum has a very poor mobility in bulk TiO2(B) due to its exceptionally high surface charge density, which would be addressable through the use of nanosized and defective materials. Our calculations suggest that TiO2(B) can offer new opportunities for developing electrode materials for aluminum-ion batteries.
[Display omitted] •Monodispersed chitosan microspheres were prepared by facile microfluidics technique.•Multi-stage interactions between heavy metal ions and adsorbents were revealed.•High selective adsorption in the present of other competitive ions was demonstrated.•Selectivity mechanism was well illustrated by ionic scale effect and DFT analysis.•The re-adsorption efficiency is still higher than 74% after 5 regeneration cycles. Many industrial wastewater streams contain heavy metals, posing serious and irreversible damage to humans and living organisms, even at low concentrations due to their high toxicity and persistence in the environment. In this study, high-performance monodispersed chitosan (CS) microspheres were prepared using a simple microfluidic method and evaluated for metal removal from contaminated water. Batch experiments were carried out to evaluate the adsorption characteristics for the removal of copper ions, one representative heavy metal, from aqueous solutions. The inherent advantages of microfluidics enabled a precise control of particle size (CV = 2.3%), while exhibiting outstanding selectivity towards target ions (adsorption capacity 75.52 mg g−1) and fair regeneration (re-adsorption efficiency 74% after 5 cycles). An integrated adsorption mechanism analytic system was developed based on different adsorption kinetics and isotherms models, providing an excellent adsorption prediction model with pseudo-second order kinetics (R2 = 0.999), while the isotherm was fitted best to the Langmuir model (R2 = 0.998). The multi-step adsorption process was revealed via quantitative measurements and schematically described. Selective adsorption performance of CS microspheres in the present of other competitive metal ions with different valence states has been demonstrated and studied by both experimental and density functional theory (DFT) analysis.
Silicon/graphite blended electrodes are promising candidates to replace graphite in lithium ion batteries, benefiting from the high capacity of silicon and the good structural stability of carbon. Models have proven essential to understand and optimise batteries with new materials. However, most previous models treat silicon/graphite blends as a single "lumped" material, offering limited understanding of the behaviors of the individual materials and thus limited design capability. Here, we present a multi-material model for silicon/graphite electrodes with detailed descriptions of the contributions of the individual active materials. The model shows that silicon introduces voltage hysteresis to silicon/graphite electrodes and consequently a "plateau shift" during delithiation of the electrodes. There will also be competition between the silicon and graphite lithiation reactions depending on silicon/graphite ratio. A dimensionless competing factor is derived to quantify the competition between the two active materials. This is demonstrated to be a useful indicator for active operating regions for each material and we demonstrate how it can be used to design cycling protocols for mitigating electrode degradation. The multi-material electrode model can be readily implemented into full-cell models and coupled with other physics to guide further development of lithium ion batteries with silicon-based electrodes.
The manufacturing difficulties of complex fractal-tree-like heat exchangers have limited their industrial applications, although many evidences have shown that they have significant advantages in heat transfer. Nevertheless, the emerging 3D printing technology has brought great opportunity for the development of complex structured device. In the present study, three-dimensional (3D) fractal-tree-like heat exchangers were designed and manufactured using 3D printing technology. Their performance was evaluated from both thermal and hydrodynamic perspectives, the flow characteristics were investigated in detail. The results show that a fractal-tree-like heat exchanger can improve hydrodynamic performance, reduce pressure drops and has great heat transfer ability. In general, the fractal-tree-like heat exchanger has a comprehensive advantage over the traditional spiral-tube exchangers as it has a higher value of coefficient of performance (COP). Furthermore, the 3D printing provides a visual, efficient, and precise approach in the present research. (C) 2018 Elsevier Ltd. All rights reserved.
This paper reports a computational demonstration and analysis of an innovative up-scaling counter-flow based microfluidic network to convert formic acid into electricity. This design consists of multi-dimensional T-shaped micro-scale channels that allow the inflow of liquid catholyte and anolyte from oppositely positioned inlets. It is revealed that the up-scaling strategy could effectively form primary and secondary counter-flow patterns, which are beneficial for high power output and fuel utilization at low flow rate operation. The design shows a breakthrough of the overall energy throughput and reactivity because of the full engagement of all available reaction sites. The energy loss mechanism introduced by the up-scaling network is also examined, demonstrating its performance intensification effect.
•The first 2D combined CH4-assisted SOEC and F-T synthesis model is developed.•A novel strategy towards low-carbon fuel generation is proposed.•Parametric studies are conducted with performance determining factors discussed.•The distribution of generated carbon-contained fuels (from C1 to C5+) is studied. CH4-assisted solid oxide electrolyzer cells (SOECs) can co-electrolyze H2O and CO2 effectively for simultaneous energy storage and CO2 utilization. Compared with conventional SOECs, CH4-assisted SOECs consume less electricity because CH4 in the anode provides part of the energy for electrolysis. As syngas (CO and H2 mixture) is generated from the co-electrolysis process, it is necessary to study its utilization through the subsequent processes, such as Fischer-Tropsch (F-T) synthesis to produce more value-added products. An F-T reactor can convert syngas into hydrocarbons, and thus it is very suitable for the utilization of syngas. In this paper, the combined CH4-assisted SOEC and F-T synthesis system is numerically studied. Validated 2D models for CH4-assisted SOEC and F-T processes are adopted for parametric studies. It is found that the cathode inlet H2O/CO2 ratio in the SOEC significantly affects the production components through the F-T process. Other operating parameters such as the operating temperature and applied voltage of the SOEC are found to greatly affect the productions of the system. This model is important for understanding and design optimization of the combined fuel-assisted SOEC and F-T synthesis system to achieve economical hydrocarbon generation.
Microfluidic technologies have emerged as a promising route for precision fabrication of uniform particles. Equipment required for a traditional microfluidic device manufacturing process is expensive and production efficiency is low. It's also difficult to separate a surfactant and continuous phase impurities from particles prepared by a liquid-liquid two-phase shear method. A novel air-blast microfluidic nozzle fabricated using low-cost and efficient 3D printing technology was proposed to prepare calcium alginate particles. Effects of control parameters and solution composition on particle diameters and homogeneity were systematically investigated. Results showed that inlet air pressure has the most significant impact. A nozzle with an outlet diameter of 300 mm can produce particles with diameters ranging from 360 mm to 2100 mm. Coefficients of variation (CV) range from 5.3% to 2.2%. For the same diameter particle prepared, a smaller nozzle outlet had a narrower particle diameter distribution and needed lower inlet air pressure. This method can be used to handle high viscosity solutions and high concentrations of solutions with insoluble solid particles. The prepared particles can be used in biological and pharmaceutical fields.
•A framework combing surrogate modeling and optimization algorithm is proposed.•The framework is used to optimize CL composition for improving maximum power density.•A 3D CFD full cell and CL agglomerate model is used for surrogate model development.•The validity of the framework is demonstrated in the paper.•The framework can guide the multi-variables optimization of complex systems. Catalyst layer (CL) is the core electrochemical reaction region of proton exchange membrane fuel cells (PEMFCs). Its composition directly determines PEMFC output performance. Existing experimental or modeling methods are still insufficient on the deep optimization of CL composition. This work develops a novel artificial intelligence (AI) framework combining a data-driven surrogate model and a stochastic optimization algorithm to achieve multi-variables global optimization for improving the maximum power density of PEMFCs. Simulation results of a three-dimensional computational fluid dynamics (CFD) PEMFC model coupled with the CL agglomerate model constitutes the database, which is then used to train the data-driven surrogate model based on Support Vector Machine (SVM), a typical AI algorithm. Prediction performance shows that the squared correlation coefficient (R-square) and mean percentage error in the test set are 0.9908 and 3.3375%, respectively. The surrogate model has demonstrated comparable accuracy to the physical model, but with much greater computation-resource efficiency: the calculation of one polarization curve will be within one second by the surrogate model, while it may cost hundreds of processor-hours by the physical CFD model. The surrogate model is then fed into a Genetic Algorithm (GA) to obtain the optimal solution of CL composition. For verification, the optimal CL composition is returned to the physical model, and the percentage error between the surrogate model predicted and physical model simulated maximum power densities under the optimal CL composition is only 1.3950%. The results indicate that the proposed framework can guide the multi-variables optimization of complex systems.
Mg-ion batteries offer a safe, low-cost, and high–energy density alternative to current Li-ion batteries. However, nonaqueous Mg-ion batteries struggle with poor ionic conductivity, while aqueous batteries face a narrow electrochemical window. Our group previously developed a water-in-salt battery with an operating voltage above 2 V yet still lower than its nonaqueous counterpart because of the dominance of proton over Mg-ion insertion in the cathode. We designed a quasi-solid-state magnesium-ion battery (QSMB) that confines the hydrogen bond network for true multivalent metal ion storage. The QSMB demonstrates an energy density of 264 W·hour kg −1 , nearly five times higher than aqueous Mg-ion batteries and a voltage plateau (2.6 to 2.0 V), outperforming other Mg-ion batteries. In addition, it retains 90% of its capacity after 900 cycles at subzero temperatures (−22°C). The QSMB leverages the advantages of aqueous and nonaqueous systems, offering an innovative approach to designing high-performing Mg-ion batteries and other multivalent metal ion batteries. The quasi-solid-state Mg-ion battery boasts 5× energy density, enhanced voltage, and excellent low-temperature performance.
The electrochemical reduction of CO2 to produce fuels and value-added organic chemicals is of great potential, providing a mechanism to convert and store renewable energy within a carbon-neutral energy circle. Currently the majority of studies report C-1 products such as carbon monoxide and formate as the major CO2 reduction products. A particularly challenging goal within CO2 electrochemical reduction is the pursuit of multi-carbon (C2+) products which have been proposed to enable a more economically viable value chain. This review summaries recent development across electro-, photoelectro- and bioelectro-catalyst developments. It also explores the role of device design and operating conditions in enabling C-C bond generation.
In this paper, a prototype of methanol microfluidic fuel cell with vapor feed anode configuration is proposed to improve the fuel and energy efficiency of the conventional liquid feed methanol microfluidic fuel cells. Peak power density of 55.4 mW cm(-2) can be achieved with this prototype under room temperature, which is 30% higher than its conventional liquid feed counterpart. Moreover, an energy efficiency of 9.4% is achieved, which is 27.5 times higher than its liquid feed counterpart. This superiority on both cell performance and energy efficiency is directly benefitted from its vapor feed anode configuration, which alleviates the fuel crossover, eliminates the fuel depletion boundary layer, and avoids the bulk anolyte wastage. The tradeoff between cell performance and fuel utilization for conventional liquid feed microfluidic fuel cells is also evaded. (C) 2015 Elsevier Ltd. All rights reserved.
"Fuel cells supply electricity by converting fuels via electrochemical reactions. The energy conversion process is highly efficient. The process is also clean as it produces no or low emission depending on the fuel used. There is a high potential that fuel cells can replace conventional batteries to achieve a higher environmental performance. However, in spite of the attractive system efficiencies and environmental benefits associated with fuel-cell technology, it has proven to be difficult to develop the scientific concepts into commercially viable industrial products. These problems have often come from both scientific and engineering issues. This edited book brings together leading researchers in various fields of engineering science from all over the world discussing the recent trends and needs on micro & nano-engineering of fuel cells, creating new engineering knowledge and optimizing the system on a micro and nano-scale. The small scales discussed in this book are particularly important, because (1) most of the underlying interactions governing the fuel cell performance occur at a scale from nanometers to microns; and (2) some engineering tasks that cannot be accomplished in macro-scale are proven achievable in micro and nano-scales. The book can be categorized into two subject areas: theoretical foundation research and applications research. The latest progress in the engineering science for electrolyte, electrode materials and whole-cell design are covered"--
Membraneless microfluidic fuel cells (M2FCs) are promising portable power sources, but they suffer from limited scalability. This paper presents a scaling-out strategy for general M2FC applications with their characteristics studied by both experiments and mathematical modeling. The present strategy addresses the issues of flow distribution non-uniformity and shunt current losses by integrating a well-designed fluid circuit. With the present strategy, parallel and series connections of four cells in an array results in a scaling-out efficiency of 93% and 82%, respectively. The effects of different parameters on the array performance as well as further device scalability are also investigated in this paper. Preferable conditions for the array operation include a high branch ionic resistance, small unit cell difference and high unit-cell performance, which can be achieved by appropriately designing the branch geometry, employing high-precision fabrication/assembly techniques and improving the single-cell materials/chemistries. It is expected that the present array can be incremented to 50 cells or above in series with over 75% efficiency as long as there is sufficiently high branch resistance or cell performance.
Microchannel reformer integrated with H-2 selective membrane offers an efficient, compact and portable way to produce hydrogen. The performance of a membrane-based microfluidic reformer is restricted by species diffusion limitation within the porous support of the membrane. Recent development in novel catalytic-supported membranes has the potential to enhance H-2 production by decimating the diffusion limitation. Loading a Pd-Ag layer on to a Ni-catalytic porous support, the membrane achieves both H-2 separation and production functions. In this study, a two-dimensional CFD model combined with chemical kinetics has been developed to simulate a microchannel autothermal reformer fed by methane. The species conversion and transport behaviors have been studied. The results show that the permeation process enhances the mass transport within the catalytic layer, and as a result, the reactions are intensified. Most notably, the effectiveness factor of the water-gas shift reaction as high as 6 is obtained. In addition, the effects of gaseous hourly space velocity (GHSV) on methane conversion and H-2 flux through the membrane are also discussed, and an optimal value of GHSV is suggested. Copyright (C) 2011, Hydrogen Energy Publications, LLC. Published by Elsevier Ltd. All rights reserved.
Conventional solid oxide fuel cells (SOFCs) could suffer from carbon deposition when fueled with hydrocarbons. For comparison, a new type of SOFC with porous electrolyte can resist carbon deposition because it allows oxygen molecules to transport from the cathode to the anode. As the transport of O-2 to the anode lowers the fuel cell performance and causes the risk of explosion, the rate of O-2 transport must be well controlled to ensure efficient and safe operation. Following our previous model, this paper focuses on electrolyte porosity optimization under various inlet methane mole fractions, inlet oxygen mole fractions and inlet gas flow rates. Furthermore, a new design with a partial porous electrolyte is proposed and numerically evaluated. The new design significantly improves the electrochemical performance compared with all-porous one. A conversion rate >90% from methane to syngas is achieved at the 0.33 inlet CH4 mole fraction with the new design. The results enhance the understanding of all porous solid oxide fuel cells and the mechanism underlying, inspiring novel designs of solid oxide fuel cells.
•The first theoretical model of optofluidic fuel cell (OFC) is established.•A dual-limiting mechanism for determining steps of OFC is discovered.•Enhancement on solar cell performance by microfluidics is achieved.•Experimental study is carried out to verify the proposed mechanism. As a new type of photoelectrochemical technologies, photocatalytic fuel cell (PFC) based on optofluidics is a promising way to provide environmental protection and generate renewable energy simultaneously. However, large-scale application of the PFC technology is hampered by its low operating current density. Here we present a theoretical model to investigate the irreversible loss in optofluidic fuel cell (OFC). Experiments have been carried out to support the theoretical analysis. Results indicate that charge transfer either within semiconductor or at the interface of semiconductor/electrolyte can be the limiting step of OFC under different circumstances and the performance of OFC is determined by the dual-limiting mechanism. The model provides a comprehensive understanding of photoelectrochemical system and offers guidelines for future research in this area.
This paper reports a computational demonstration and analysis of an innovative counter-flow-based microfluidic unit and its upscaling network, which is compatible with previously developed dual-electrolyte protocols and numerous other electrochemical applications. This design consists of multidimensional T-shaped microchannels that allow the effective formation of primary and secondary counter-flow patterns, which are beneficial for both high-performance regenerative H-2/O-2 redox cells and flow batteries at a low electrolyte flow-rate operation. This novel design demonstrates the potential to achieve high overall energy throughput and reactivity because of the full utilization of all available reaction sites. A computational study on energy and pressure loss mechanism during scale-out is also examined, thereby advancing the realization of an economical electrolyte-recycling scheme.
A novel facile and cost‐effective synthesis method for anatase TiO2 nanoparticles has been developed by using poly‐acrylic acid hydrogel as template at room temperature. The newly developed synthesis method avoids the use of hazardous reagents and/or hydrothermal steps, and enables production of highly active TiO2 nanoparticles from low cost inorganic titanium sources. The synthesized TiO2 nanoparticles have been studied in several applications including dye‐sensitized solar cells as a photoanode as well as in organics degradation of methyl orange in aqueous media. Good photocatalytic performances were obtained in both applications. A low‐cost, eco‐friendly and simple preparation method which allows the utilisation either organic or less expensive inorganic sources to obtain anatase TiO2 nanoparticles is reported in this work. The as‐prepared nanoparticles showed promising results in DSSCs as well as in dye degradation.
Understanding current distribution in operating Vanadium redox flow batteries (VRFBs) is vital for improving battery design and performance as the non-uniform current density distribution is known as a major cause of the corrosion and side reactions in VRFBs. However, the existing method for current density measurement in the VRFB with flow field is complex and the rib conductive structure of graphite plate is changed which reduces the measurement accuracy and reliability. In this study, we propose a novel pluggable current collector for in-operando current distribution measurements which greatly simplifies the current density distribution measurement process in VRFBs with flow field. The new method relies on eight pluggable graphite units in a flow plate frame to replicate the actual serpentine flow field structure, thereby providing higher measurement reliability compared to the existing methods which are mostly invasive. Using the new method, current density distributions at different electrode compression ratios (CR) and inflow electrolyte rates are investigated. It is found that insufficient supplemental reactant in electrode border region is the main reason for the uneven current density distribution and the current density non-uniformity is intensified in the initial and final stages of cycling process. Increasing CR can improve the current density in the electrode core region. The overall current density uniformity in a VRFB with serpentine flow field can be enhanced by increasing the inflow electrolyte rate. The developed method is believed to facilitate the understanding of the coupled local reactions and transport processes and guide on the future design of VRFBs. (c) 2021 Elsevier Ltd. All rights reserved.
Aqueous hybrid Na-Zn ion batteries (ASZIBs) are promising for large-scale energy storage due to their low cost and potential for high output voltage. However, most ASZIBs show limited discharge voltage (
Many researches have indicated that the side chain length of perfluorosulfonic acid (PFSA) polymers had nonnegligible effects on PFSA membrane properties. However, the effects have not been completely revealed yet. This paper uses molecular dynamics simulation to explore the effects of side chain length on the structure, oxygen transport and thermal conductivity of PFSA membrane. In PFSA membrane with longer side chains, the maximum size of water clusters is larger, while the connectivity of water clusters is poorer and size distribution of water clusters is more heterogenous. And the structural characteristics have a strong effect on the diffusion and solubility coefficient of oxygen molecules. The diffusion of oxygen molecules may be hindered due to the existence of small pores in PFSA membrane with longer side chains even at high water content, leading to a lower diffusion coefficient. In addition, with the increment of water content lambda, the solubility coefficient of oxygen molecules in PFSA membrane with shorter side chains decreases more quickly at lambda < 12, resulting in a most obvious difference of solubility coefficients for different side chain lengths when. is about 12. Moreover, the thermal conductivity of PFSA membrane with shorter side chains is higher. (C) 2019 The Electrochemical Society.
A new oxygen-annealing treatment for Pd supported on graphene was employed to enhance the electro-catalytic performance in formic acid microfluidic fuel cells. Experiments show that both the electro-oxidation reactivity and CO tolerance of the annealed catalyst are significantly improved. When testing the fuel cell, the maximum power density with annealed catalyst is 41% higher than the unannealed Pd@graphene catalyst, and 85% higher than Pd@Vulcan XC-72. This enhancement is attributed to the Pd-O bond formation during the oxygen annealing step, which induces greater CO electro-oxidation reactivity. (C) 2016 Hydrogen Energy Publications LLC. Published by Elsevier Ltd. All rights reserved.
CO2 can be converted to useful fuels by electrochemistry method. As an effective strategy to address greenhouse effect and energy storage shortage, electrochemical reduction of CO2 still needs major improvements on its efficiency and reactivity. This work demonstrates a switchable pH-differential dual electrolyte microfluidic reactor (DEMR) that enhances the thermodynamic property and raises the electrochemical performance based on a laminar flow membrane-less architecture. It is the first time that the CO2 electrochemical reduction reaction is freed from the hindrances of thermodynamic limitation. DEMR has also achieved a high reactivity and Faradaic efficiency, revealing the potential for larger-scale applications in future urban energy network, such as a carbon-neutral energy conversion system.
A two-dimensional numerical model for a photoelectrochemical (PEC) cell.for CO2 utilization and fuel production was developed using COMSOL Multiphysics and validated with published experimental data. The model couples computational fluid dynamics with electrochemical kinetics to account for the complex interactions inside the PEC driven by light absorption operating at the Shockyley-Queisser limit. Charge and species conservation, fluid flow and electrochemical characteristics are studied. The flow rate of CO2 is found to be the factor affecting the cell performance. Parametric study for operating conditions is necessary in future work. (C) 2019 The Authors. Published by Elsevier Ltd.
•The equality, diversity and inclusion in energy and AI has been discussed.•The importance of just transition has been highlighted.•Coded bias need to be eliminated for energy and AI research.•A call for action is presented. [Display omitted] .
[Display omitted] •PEMFC model with gaseous, liquid, and dissolved water conversion is developed.•Multi-objective optimization between EWRE and electrochemistry is conducted.•Optimized EWRE achieves 141 % with current density of 0.7163 A·cm−2.•Efficient water recovery contributes to extending the applications of PEMFC. Water generated by electrochemical reaction in the proton exchange membrane fuel cell (PEMFC) could be used as an important resource. Several studies have been carried out to recycle the water from PEMFC emissions, which verifies the feasibility of water recovery. However, the quantitative description of the water recovery mechanism inside PEMFC is not clear and the water recovery performance could be further improved from the perspective of internal mass transfer. In this paper, a two-dimensional model is developed to present the fluid flow and mass transfer inside a PEMFC. Also, a detailed investigation into the effects of operating parameters, including pressure, temperature, and relative humidity, on both the electrochemical performance and the water recovery performance is conducted. Besides, a multi-objective optimization is carried out for the performance optimization of PEMFC. The results show that the expanded water recovery efficiency (EWRE) of PEMFC is more sensitive to pressure and relative humidity than temperature. The optimum solution at 1 atm shows that EWRE reaches 141 % with the current density of 0.7163 A·cm−2. The operation strategy of decreasing the humidification temperature and increasing the relative humidity at the cathode inlet, and maintaining PEMFC working at a moderate temperature is proposed to keep the balance between the electrochemical and water recovery performance. This work contributes to the operation optimization of water recovery and to the application extension of PEMFC.
Solar photocatalytic fuel cell (PFC) is a promising technology for environmental-friendly wastewater treatment and simultaneous production of electricity. In this study, PFC was enhanced by using CdS quantum-dot-sensitized TiO2 nanorod array deposited onto FTO glass as effective photoanode. Moreover, gas diffusion electrode was employed to improve oxygen reduction reaction at the cathode. The material characterization shows that an array of 1.2-mu m TiO2 nanorods is decorated with 10-nm CdS quantum dots, which significantly improve solar light harvesting ability. The results of the PFC performance study indicate that light irradiation, acetic acid concentration, electrolyte pH and conductivity have significant influence on the short-circuit current and maximum power density. When the PFC operates at the optimum pH of 4.6, the short-circuit current and maximum power density are 1.79 mA/cm(2) and 1134 mW/cm(2), respectively. It is found that increasing the electrolyte conductivity is an effective approach to improve the PFC performance. The highest short-circuit current of 5.1 mA/cm(2) and maximum power density of 3980 mW/cm(2) are obtained with electrolyte having a conductivity of 63.1-mS/cm. In addition, the test results of various pure and practical organic substances in PFC further suggest that it is feasible to use sunlight as a driving force to clean up wastewater with simultaneous electricity production. (C) 2014 Elsevier B.V. All rights reserved.
Pursuing high energy efficiency and reaction rate is an effective direction in mitigating the energy and climate crisis. Mass transfer intensification is a powerful approach to enhance the energy efficiency and reaction rate in the energy conversion and storage processes. The nature-inspired channels are outstanding tools to uniformly distribute the reactants, fast remove products, and reduce the diffusion distance for mass transfer intensification in monolithic adsorbents, structured catalysts, and electrodes. Besides, hierarchical pores are also a contributor to improve the reactant diffusion rate in the matrix. Additive manufacturing, combining the design of printing material and post-treatment, provides a promising opportunity to accurately control the channels and adjust the pores in electrodes, catalysts, and adsorbents. This review summarizes the main state-of-the-art methods to design ideal channels and to control the sizes and amounts of pores for the optimum hierarchical structure design, as well as the techniques to realize the hierarchical structure by additive manufacturing. In addition, this review also communicates the recently unlocked advantages of the designable channels and hierarchical pores in applications of adsorptions, chemical reactions, and electrochemical energy storage. Lastly, a new approach to design and fabricate the hierarchical structures is proposed, along with its potential benefits.
Electrochemical and photoelectrochemical systems for hydrogen production and CO2 reduction are regarded as prospective technologies to achieve carbon-free energy vision. Electrolytes in different pH environment is desirable for each half electrochemical reaction to optimize the electrode kinetics and reduce the cost of noble metal catalysts. The bipolar membrane provides excellent opportunities to enable pH-differential operation. However, the effect of the bipolar membrane on electrochemical performance is not clarified yet. Here, a numerical modeling framework for bipolar membrane-based cells for electrochemical and photoelectrochemical applications was presented to study the viability of using bipolar membrane in the aspect of energy loss. The model for the first time successfully integrates the water dissociation at the bipolar membrane with the rest electrode kinetics and mass transfer, by treating the interfacial layer as a virtual electrode. Based on the model, the activation loss involved in the bipolar membrane devices were identified and compared with the ones with conventional monopolar membranes. A critical current density was identified for bipolar membranes, which is determined by the water dissociation performance of the membrane. Based on the critical current, the viable operation regions of using the bipolar membrane can be clarified for the electrochemical device. It is found that the bipolar membrane-based photoelectrochemical reactor has higher energy conversion efficiency than monopolar membrane configurations. However, the advantage of bipolar membrane becomes vanishing with photocurrent rising.
•Develops a high-accuracy data-driven surrogate model of PEMFCs.•Realizes the efficient multi-physics-resolved digital twin of PEMFCs.•Demonstrates the PEMFC healthy operation envelope and state map.•Proposes application prospects of combining physical models and data-driven methods. The development of multi-physics-resolved digital twins of proton exchange membrane fuel cells (PEMFCs) is significant for the advancement of this technology. Here, to solve this scientific issue, a surrogate modelling method that combines a state-of-the-art three-dimensional PEMFC physical model and data-driven model is proposed. The surrogate modelling prediction results demonstrate that the test-set relative root mean square errors (rRMSEs) of the multi-physics fields range from 3.88% to 24.80% and can mirror the multi-physics field distribution characteristics well. In summary, for multi-physics field prediction, the data-driven surrogate model has a comparable accuracy to the comprehensive 3D physical model; however, it considerably reduces the cost of computation and time and achieves the efficient multi-physics-resolved digital-twin. Two model-based designs based on the as-developed digital twin framework, i.e. the PEMFC healthy operation envelope and the PEMFC state map, are demonstrated. This study highlights the potential of combining data-driven approaches and comprehensive physical models to develop the digital twin of complex systems, such as PEMFCs. [Display omitted]
•A microfluidic approach is designed for measuring diffusion in zinc electrolytes.•ZnSO4 diffusion at different concentrations is measured simultaneously.•The measured diffusion coefficients agree well with literature values.•The method is 10 times faster than the conventional method based on diffusion cells. Rechargeable zinc batteries have emerged as an inexpensive and safe post-lithium-ion battery technology and have received increasing research interest. Developing suitable electrolytes and understanding their transport properties lie at the heart of successful zinc battery technologies as the battery behaviour is a strong function of ion transport in the electrolytes. To accelerate the research and development process, herein we demonstrate a low-cost and high-throughput approach to measure the diffusion in zinc electrolytes at different concentrations simultaneously. The new approach combines Raman microspectroscopy and a multiplexed microfluidic chip with integrated micromixers, concentration gradient generators and a Y-sensor array. Aqueous-based zinc sulphate electrolytes, widely used in zinc batteries, were used for a proof-of-concept. The measured diffusion coefficients for different electrolyte concentrations show good agreement with literature values. With four electrolyte samples in this study, the developed approach requires minimum 0.5 mL of the electrolyte solutions and 30 mins, which is over ten times faster than a typical diffusion measurement with the conventional electrochemical approach in restricted-diffusion cells. The microfluidic chip is readily scalable to further increase the throughput, and can be extended to for use of measuring different (i.e. organic and aqueous) and even mixtures of electrolytes (i.e. ethylene carbonate and dimethyl carbonate) as well as salts (Li+, Na+, Mg2+, etc.).
In this study, facile synthesis of hollow TiO2 spheres composed of a high percentage of reactive facets (similar to 85%) is successfully prepared with TiOSO4 and HBF4 by the hydrothermal method. Results reveal that hollow TiO2 spheres of 605 nm to 1.21 mu m in size are in the anatase phase with sulfur doping. The variation in shell morphologies (e. g. polyhedron and nanosheet) can be realized by adjusting the reactant concentrations, while the molar ratio of TiOSO4 to HBF4 is maintained at 5 : 3. Based on the time-dependent morphology evolution study, the growth mechanism of hollow structure formation via self-templating and dissolution-recrystallization processes is discussed. The effects of reactant concentrations on TiO2 morphology are investigated to understand the dual roles of HBF4. Results also indicate that hollow TiO2 spheres with nanosheetmorphology having 85% (001) facets exhibit 1.4-5 times higher performance than their counterparts in photocatalytic hydrogen production. The enhanced photocatalytic activity is ascribed to the combined effects of their unique hollow structure, high BET specific surface area (139.1 m(2) g(-1)) and high percentage of exposed reactive facets (85%). This study demonstrates a promising strategy for large-scale production of hollow TiO2 spheres using a template-and surfactant-free process for photocatalysis applications.
Aluminum-air cells have attracted a lot of interests because they have the highest volumetric capacity density in theory among the different metal-air systems. To overcome the self-discharge issue of aluminum, a microfluidic aluminum-air cell working with KOH methanol-based anolyte was developed in this work. A specific capacity up to 2507 mAh g−1 (that is, 84.1% of the theoretical value) was achieved experimentally. The KOH concentration and water content in the methanol-based anolyte were found to have direct influence on the cell performance. A possible mechanism of the aluminum reactions in KOH methanol-based electrolyte was proposed to explain the observed phenomenon. •A concept of inorganic/organic hybrid electrolyte cell is demonstrated in Al-air cells.•A non-direct counter-flow microfluidic platform is developed.•High anodic specific capacity up to 2507 mAh g−1 is achieved.•The reaction mechanisms of aluminum in methanol-based KOH anolyte are investigated.
This article reports a safe, low-cost, and industrially applicable magnetite supported on activated carbon catalyst that can be magnetically retrieved from the solid and reused multiple times without the need of a regeneration step. The FeOx/C catalyst improved the bio-oil yield by 19.7 ± 0.96 % when compared to the uncatalysed reaction at 320 °C for the HTL of draff (brewer’s spent grains). The use of homogeneous Na2CO3 base as a catalyst and co-catalyst, improved carbon extraction into the aqueous phase. The exceptional catalytic activity can be attributed to the Fe3O4 phase which can produce in-situ H2 that improves the biomass decomposition and oil property with an energy recovery of ∼84 %. The FeOx/C catalyst was separated using magnetic retrieval and maintained its catalytic activity even up to 5 reaction cycles showing potential as a cheap catalyst for HTL reactions and can be scaled-up for industrial applications.
Solid oxide electrolysis cell (SOEC) is a clean and highly efficient technology that converts electrical energy into chemical energy, which is regarded as a promising approach to enable hydrogen production, carbon dioxide utilization and nitrogen reduction, and more. One of the main challenges faced by SOEC is performance descending. Numerical modelling is expected to provide insights on performance evolution and material degradation. Different assumptions and methodologies are applied to model the physicochemical processes in a length scale from molecule scale to system scale. It is rational and can be brought great convenience into modelling to make assumptions and simplifications when focusing on a single length scale, however, valuable details can be missing. Multiscale modelling is expected to maintain more information and generate a systematic understanding of the working mechanism of SOEC. In this paper, the modelling methods at various scale levels are summarized. Then, the recent advances in numerical studying on SOEC are reviewed with a focus on multiscale modelling works bridging two or more contiguous length scales. The challenges and future research directions are proposed to promote the multiscale modelling.
Body sensor network is a promising medical technology to address the overwhelming global aging, which requires advanced micro power sources with high energy density, long lifetime and good biocompatibility. Potential candidates include batteries, fuel cells, energy harvesters and supercapacitors, each of which have their own merits and demerits. Batteries are currently the most mature product which has been extensively employed in the body sensor network, but the improvement of their energy density is relatively sluggish due to the cumbersome material storage. On the contrary, micro fuel cells can achieve much higher energy density because of their lightweight fuel and oxidant, which can be refueled instantly when applied in wearable sensors. For implanted devices, both abiotic and biotic fuel cells can utilize the dissolved glucose in the body fluid as fuel. As for energy harvesters, they can receive energy from both the external environment such as solar and radiofrequency, and the human body itself such as body heat and motion. Finally, supercapacitor is an effective energy storage component especially for energy harvesters, which can achieve excellent cycle stability. In general, the selection of micro power sources should consider the specific requirement from the specific sensor node, so that a body sensor network with hybrid power supply is much more reliable than that with single type of energy supply. With the continuous development of different power technologies, the body sensor network is expected to be more lightweight, unobtrusive and reliable, leading to a low-cost and ubiquitous healthcare in the near future. •A review of micro power sources for future body sensor network is presented.•Relevant batteries, fuel cells, energy harvesters and supercapacitor are summarized.•Suitable power sources for wearable sensors and implantable sensors are compared.•Hybrid power strategy is proposed for the development of future body sensor network.
As a carbon capture and utilization (CCU) technology, gas diffusion electrode (GDE) based electrochemical CO2 reduction reaction (eCO2RR) can convert CO2 to valuable products, such as formate and CO. However, the electrode parameters and operational conditions need to be studied and optimised to enhance the performance and reduce the net cost of the eCO2RR process before its industrial application. In this work, a machine learning algorithm, i.e., extended adaptive hybrid functions (E-AHF) is combined with a multi-physics model for the data-driven three-objective optimisation and techno-economic analysis of the GDE-based eCO2RR process. The effects of eight design variables on the product yield (PY), CO2 conversion (CR) and specific electrical energy consumption (SEEC) of the process are analysed. The results show that the R2 of the E-AHF model for the prediction of PY, CR and SEEC are all higher than 0.96, indicating the high accuracy of the developed machine learning algorithm for the prediction of the eCO2RR process. The process performance experiences a notable improvement after optimisation and is affected by a combination of eight variables, among which the electrolyte concentration having the most significant impact on PY and CR. The optimal trade-off single-pass PY, CR and SEEC are 3.25×10-9 kg s-1, 0.663% and 9.95 kWh kg-1 based on flow channels with 1 cm in length, respectively. The SEEC is reduced by nearly half and PY and CR are improved more than two times after optimisation. The production cost of the GDEbased eCO2RR process was approximately $378 t-1product (CO and formate), much lower than that of traditional CO2 utilisation factories ($835 t-1product). The electricity cost accounted for more than 80% of the total cost, amounting to $318 t-1, indicating that cheaper and cleaner electricity sources would further reduce the production cost of the process, which is the key to the economics of this technology.
This work evaluated the practicability and economy of the enhanced weathering (EW)-based CO2 capture in series packed bubble column (S-PBC) contactors operated with different process configurations and conditions. The S-PBC contactors are designed to fully use the advantages of abundant seawater and highly efficient freshwater through a holistic M4 model, including multi-physics, machine learning, multi-variable and multi-objective optimisation. An economic analysis is then performed to investigate the cost of different S-PBC configurations. A data-driven surrogate model based on a novel machine learning algorithm, extended adaptive hybrid functions (E-AHF), is implemented and trained by the data generated by the physics-based models. GA and NSGA-II are applied to perform single- and multi-objective optimisation to achieve maximum CO2 capture rate (CR) and minimum energy consumption (EC) with the optimal values of eight design variables. The R2 for the prediction of CR and EC is higher than 0.96 and the relative errors are lower than 5%. The M4 model has proven to be an efficient way to perform multi-variable and multi-objective optimisation, that significantly reduces computational time and resources while maintaining high prediction accuracy. The trade-off of the maximum CR and minimum EC is presented by the Pareto front, with the optimal values of 0.1014 kg h−1 for CR and 6.1855 MJ kg−1CO2 for EC. The calculated net cost of the most promising S-PBC configuration is around 400 $ t−1CO2, which is about 100 $ t−1CO2 lower than the net cost of current direct air capture (DAC), but compromised by slower CO2 capture rate.
While low carbon jet fuels (LCJF) offer a viable alternative to conventional jet fuels in terms of reducing aviation emissions, the higher fuel costs may be passed on to customers in the form of increased ticket prices. However, there has been little research into the public's willingness to pay (WTP) for LCJF use. Our study addresses this gap by exploring citizen's perceptions of, attitudes toward, and willingness to pay a premium ticket price. We conducted an online survey among UK citizens (N = 1008) who flew at least once a year. We used ordered logistic regression to predict the factors that influence WTP for LCJF. The findings confirmed the existence of three factors that explain air travellers' WTP: social trust, perceived risks, and attitude. Although the overall perception of the benefits of LCJF outweighs the associated risks, the level of awareness of LCJF use is rather low. Despite a favourable attitude toward LCJF use, the majority of respondents were unwilling to pay more for carbon-neutral air travel. Our research contributes to and expands the literature on the current debates about acceptance and WTP for LCJF and energy transitions. Additionally, the findings of our study encourage public and corporate managers to leverage the identified key factors to inform and structure campaigns to increase the acceptability of LCJF use.
The graded distribution of Pt loading in the catalyst layer (CL) and the porosity of the gas diffusion layer (GDL) significantly affect the spatial distributions of electrochemical reaction and mass transport rates, thus influencing the cell performance and durability. A sophisticated physics-based model is established to study the influence of graded Pt loading and GDL porosity at the cathode, with their distribution func-tion obeying the elliptic equation along the in-plane and through-plane directions, on the current density and its uniformity at a given cell voltage. To reduce the computational time and resources, an RNN algorithm-based data-driven surrogate model is developed to assist in the identification of the relation-ship between the design parameters and the objective functions. Latin hypercube sampling (LHS) method is implemented for sampling and then the initial data acquisition is conducted for training and testing the surrogate model. Results show that the machine learning (ML) algorithm could effectively assist the opti-mal design of the functionally graded electrode, and the surrogate model achieves > 97.9 % prediction accuracy for current density and less than 0.13 root mean square error (RMSE) for current homogeneity. Both the individual variation of Pt loading and GDL porosity and their interaction are respectively anal-ysed. Results also indicate that the inhomogeneous Pt distribution improves the current density. On the contrary, GDL porosity has a greater impact on the cell performance since current density monotonically increases with the homogeneous GDL porosity. When both the inhomogeneous distributions of Pt loading and GDL porosity are simultaneously considered, the homogeneity of current density is improved. However, the improvement of the homogeneity of current density (increases by 54 %) sacrifices the max-imum current density (reduces by 22 %).(c) 2022 The Author(s). Published by Elsevier Ltd. This is an open access article under the CC BY license (http://creativecommons.org/licenses/by/4.0/).
•Major losses include thermodynamic, kinetic and electrical aspects.•Acid-base interface for the specific setting of a PMEC is simulated.•The elevated reactor performance is validated experimentally and numerically. This paper reports a mathematical model for calculating various losses in a pH differential microfluidic electrolytic cell (PMEC) for CO2 to formic acid conversion. The microfluidic characteristics of the cell were examined, and in respect of electro-chemical equilibrium states, major limiting factors such as mass transfer constraints, kinetic losses and overpotentials, were considered and acid–base interface and neutralisation losses therein identified. Losses of electrical resistance on electrodes and within micro-channels were quantified, and computational results were validated against previous experimental data. To the best of our knowledge, the model is the first for determining dual electrolyte arrangements and associated losses and can be used to develop parametric optimisation strategies.
Numerous advanced oxidation processes (AOPs) have been developed for conditioning sewage sludge due to their excellent dewaterability, which can reduce the water content of sludge to below 60 wt %. However, the application of different chemical reagents for sludge dewatering inevitably causes varying degrees of environmental impact and economic pressure. It is still questionable whether advanced sludge dewatering processes can reduce environmental and economic burdens. In this work, life cycle assessment (LCA) and life cycle cost (LCC) of three types of AOPs (Fe2+/H2O2, Fe2+/Ca(ClO)2, and Fe2+/Na2S2O8) were compared to the traditional conditioner (Fe3+/CaO) for the first time based on pilot-scale study data to measure the optimal choice of environmental and economic performance. Fe2+/Ca(ClO)2 and Fe2+/H2O2 exhibited the highest and lowest environmental and economic burdens, respectively. Among them, the total environmental impact and total cost of Fe2+/H2O2 were equivalent to 54−62% and 56−66% of other processes, respectively. In the sludge treatment routes, the sludge conditioning and landfill stages were the largest contributors to the environmental impact and economic cost. The greenhouse gas emissions and particulate matter generation were the main impact indicators of LCA and LCC. In addition, the superior dewatering performance did not result in significant environmental benefits. The obtained LCA and LCC results will support the selection and application of sludge pretreatment processes in wastewater treatment plants and contribute to the improvement and development of the technology.
The use of flow-through porous electrodes is identified as a promising approach to enhance the performance of microfluidic fuel cell (MFC). In this study, a dimensionless computational model is developed to analyze the distribution characteristics of the electrochemical reactions in flow-through porous electrodes of MFC. One important finding is that the electrochemical reactions mainly occur in the vicinity of the anode and cathode outlets. Based on this observation, the new design of partial modification at reactive sites is proposed to reduce the MFC cost. It is found that MFC with partial modification can achieve comparable performance with that of conventional full electrode modification, demonstrating the feasibility of partial modification method. The partial modification also offers a promising alternative to avoid unwanted secondary reaction, such as hydrogen evolution. (C) 2015 Published by Elsevier Ltd.
•Plasmonic heating effect of Au nanoparticles under continuous flow conditions.•Optical and photothermal properties of nanofluids investigated.•Au nanofluid showed higher absorption and photothermal performance.•Flow rate and nanoparticles concentration affected the photothermal performance. The aim of this study is to enhance our understanding on photothermal performance of plasmonic Au/TiO2 nanoparticles by conducting a well-controlled experiment under continuous flow conditions. Herein, plasmonic heating experiments of Au nanoparticles decorated on TiO2 nanoparticles were performed using 532 nm laser irradiation. Different parameters, such as Au loading, concentration of nanoparticles, flow rate and laser intensity that could affect the optical and photothermal properties of the nanofluids were studied. The results revealed that the photothermal performance of the nanofluids was remarkably increased in the presence of Au. Particularly, Au nanofluid exhibits a significant higher temperature achieving up to 32 °C compared to that of TiO2 nanofluid (22.5 °C) and water-based fluid (20.5 °C) which is attributed to the localized surface plasmon resonance effect on the surface of Au nanoparticles. The concept of continuous-flow based plasmonic heating of Au/TiO2 nanofluid, with considerable optical and thermal properties, is a promising approach in efficient photothermal applications such as thermal energy supply in industrial chemical processes.
Fuel cell coupled with biomass-derived fuel processor can convert renewable energy into a useful form in an environmental-friendly and CO2-neutral manner. it is considered as one of the most promising energy supply systems in the future. Biomass-derived fuels, such as ethanol, methanol, biodiesel, glycerol, and biogas, can be fed to a fuel processor as a raw fuel for reforming by autothermal reforming, steam reforming, partial oxidation, or other reforming methods. Catalysts play an important role in the fuel processor to convert biomass fuels with high hydrogen selectivity. The processor configuration is another crucial factor determining the application and the performance of a biomass fuel processing system. The newly developed monolithic reactor, micro-reactor, and internal reforming technologies have demonstrated that they are robust in converting a wide range of biomass fuels with high efficiency. This paper provides a review of the biomass-derived fuel processing technologies from various perspectives including the feedstock, reforming mechanisms, catalysts, and processor configurations. The research challenges and future development of biomass fuel processor are also discussed. (C) 2008 Elsevier Ltd. All rights reserved.
This review discusses a range of in situ/operando techniques based on optical microscopy reported in literatures for studying electrochemical energy systems. Compared to other techniques (scanning probe microscopy, electron microscopy, X‐ray microscopy), optical microscopy offers many advantages including the simplicity of the instrument and operation, cost effectiveness, and nondestructive nature. In the past few decades, significant advances in the field of optical microscopy have been made, enabling new opportunities of more elaborate studies on electrochemical energy systems. Herein, different methodologies are compared, with the emphasis on experimental setup designs and findings, to illustrate their aptness. Optical microscopy offers many advantages including the simplicity, cost effectiveness, and nondestructive nature. In the past few decades, significant advances in the field of optical microscopy have been made, enabling new opportunities of more elaborate studies on electrochemical energy systems. This review discusses a range of in situ/operando techniques based on optical microscopy reported in literatures for studying electrochemical energy systems.
This paper reports a theoretical investigation on the orientation-dependence of the performance of membraneless microfluidic fuel cell (MMFC) and the relevant gravity effects. A coupled computational fluid dynamics (CFD)/electrochemical model is used for the analysis. The results show that gravity significantly affects the hydrodynamics in the microchannel and consequently reduces the cell performance dramatically with certain orientations. This important phenomenon has been widely ignored in previous works. Parametric analysis offers useful insights for enhancing the MMFC designs that minimize the adverse effects towards a feature of orientation-independence. (C) 2011 Elsevier Ltd. All rights reserved.
Over the last decades, long-term fossil fuel shortage and growing global demand for energy ignited an interest in developing alternative and "green" approaches for fuel production. One promising way to generate solar fuels is photocatalytic or photoelectrochemical (PEC) CO2 reduction. Although various research efforts were undertaken to improve the efficiency and stability of PEC devices, many questions remain that have not been adequately answered due to lack of understanding of the reaction mechanisms and underlying limiting factors. In this study, we illustrate the thermodynamics of single or double junction photo-absorbing materials. Finally, this research work investigated the efficiency limits of solar-driven CO2 conversion into ethanol as a two-step process with formate as intermediate. (C) 2019 The Authors. Published by Elsevier Ltd.
Solar-fuel formation via photoelectrochemical (PEC) routes using water and CO2 as feedstock has attracted much attention. Most PEC CO2 reduction studies have been focused on the development of novel photoactive materials; however, there is still a lack of understanding of the key limiting factors of this process. In this study, the theoretical limits of Solar-to-Fuel (STF) efficiencies of single- and dual-junction photo-absorbing materials are illustrated for single-step multi-electron CO2 reduction into fuels including HCOO-, CO, CH3OH and C2H5OH. It is also highlighted that STF efficiency depends on the route of two-step PEC CO2 reduction process using CH3OH as a model fuel. Finally, it is illustrated the beneficial role of alternative strategies such as dual-junction photo-absorbing electrodes, externally applied bias and subsequent reactor chambers on the maximum theoretical efficiencies of PEC CO2 reduction.
With the rapid growth and development of proton-exchange membrane fuel cell (PEMFC) technology, there has been increasing demand for clean and sustainable global energy applications. Of the many device-level and infrastructure challenges that need to be overcome before wide commercialization can be realized, one of the most critical ones is increasing the PEMFC power density, and ambitious goals have been proposed globally. For example, the short- and long-term power density goals of Japan's New Energy and Industrial Technology Development Organization are 6 kilowatts per litre by 2030 and 9 kilowatts per litre by 2040, respectively. To this end, here we propose technical development directions for next-generation high-power-density PEMFCs. We present the latest ideas for improvements in the membrane electrode assembly and its components with regard to water and thermal management and materials. These concepts are expected to be implemented in next-generation PEMFCs to achieve high power density. This Perspective reviews the recent technical developments in the components of the fuel cell stack in proton-exchange membrane fuel cell vehicles and outlines the road towards large-scale commercialization of such vehicles.
[Display omitted] •A multi-physics and multi-flow model of PEM fuel cell is developed and validated.•Inhomogeneous Pt loading across channel and rib areas is investigated.•About 70% cell performance is retained without platinum placed under the rib.•Studying effects of multiple parameters on Pt utilisation under the ribs and resulting cell performance.•Optimal under-the-rib Pt loading improves cell performance and saves Pt usage. The non-uniform distribution of platinum across the channel and rib area within the cathode catalyst layers of proton exchange membrane fuel cells was experimentally and numerically studied. A two-dimensional, two-phase flow, isothermal model was developed to investigate the influence of various parameters on the current density with respect to electrode surface area and platinum loading. Approximately 70% cell performance was retained when no platinum was placed under the rib. Owing to the slow oxygen supply to the rib area, increasing the platinum loading significantly reduced the effective oxygen concentration under the rib, leading to low platinum utilisation in this region. The large channel/rib width ratio and highly porous gas diffusion layer improved the cell performance when the conductivity was sufficiently high. The optimal platinum loading under the rib matched the oxygen consumption rate under the rib and the rate of oxygen supply to the rib area from the channel.
The membraneless microfluidic fuel cell (MFC) is a promising micro-scale power source with potentially wide applications. MFC commonly relies on the co-laminar microfluidic platform in which redox streams flow in parallel in a microchannel. The nature of this cell architecture limits the mass transport inside the cell, often resulting in low power density. To overcome the issues, we propose an innovative concept of chaotic flow-based fuel cell (CFFC), which is built on a counter-flow microfluidic platform with the flow channel patterned with micro-ridges. A CFD/electrochemical model is used to predict the performance and investigate the underlay mechanism of the CFFC. Two theoretical upper bounds, i.e., the limiting current and limiting fuel conversion for conventional MFC, are derived. Through the results, it is found that the generation of chaotic flow inside the patterned activation zone enables the CFFC to exceed the theoretical limitations and work with over-limiting current for high-power output. Meanwhile, the interfacial mixing and crossover is minimized by the counter-flow microfluidics, allowing for over-limiting fuel conversion to useful electricity output. The achievement of unprecedented operating regime demonstrated in this study open up a new direction towards optimization, operating and design of the MFC. (C) 2011 Elsevier B.V. All rights reserved.
In recent years, the use of additive manufacturing (AM) has been demonstrated in the fabrication of components in polymer electrolyte membrane fuel cell, solid oxide fuel cell (SOFC), microbial fuel cell (MFC) and laminar flow-based fuel cell (LFFC). Various AM technologies have been successfully demonstrated in fuel cell manufacturing include material extrusion, powder bed fusion, vat photopolymerization and binder jetting. One of the unique advantages of AM is the ability to handle sophisticated design with features ranging from macro to micro scales, which are inaccessible by conventional manufacturing technologies. Well-designed complex 3D structures were reported to have the potential for increasing the performance of fuel cells. Therefore, AM presents itself as a promising fabrication method to promote the development of fuel cells. Besides, AM also showed its specialty in time-saving, flexibility, and on-demand manufacturability in the fabrication of fuel cell components. In spite of the prospects of AM in fuel cells, more studies and researches are required to overcome challenges, such as availability of material, manufacturing quality, and the costs. This review focuses on the advantages and applications of additive manufacturing that enable improvement in fuel cell performance. The critical challenges and directions for future development are also highlighted.
PEMFC (proton exchange membrane or polymer electrolyte membrane fuel cell) is a potential candidate as a future power source for automobile applications. Water and thermal management is important to PEMFC operation. Numerical models, which describe the transport and electrochemical phenomena occurring in PEMFCs, are important to the water and thermal management of fuel cells. 3D (three-dimensional) multi-scale CFD (computational fluid dynamics) models take into account the real geometry structure and thus are capable of predicting real operation/performance. In this study, a 3D multi-phase CFD model is employed to simulate a large-scale PEMFC (109.93 cm2) under various operating conditions. More specifically, the effects of operating pressure (1.0-4.0 atm) on fuel cell performance and internal water and thermal characteristics are studied in detail under two inlet humidities, 100% and 40%. It is found that the PEMFC performance increases with the increment of operating pressure as a result of the increased reactant concentration. Meanwhile, the pressure drop through the PEMFC is decreased under high operating pressure because of the large inlet gas density. Additionally, for low humidity operation, pressurized inlet flows increase the water vapor concentration, which is helpful to improve the membrane hydration and hence reduce the Ohmic loss.
The local pH variation near the surface of CO2 reduction electrodes is important but hard to study. We develop a continuous-flow Raman electrochemical cell that enables the first experimental study of the local pH near a CO2 reduction gas diffusion electrode under reaction conditions. At zero current, CO2 chemically reacts with the 1 M KOH electrolyte at the interface to form HCO3- and CO32-. The local pH on the cathode surface is 7.2, and the HCO3- concentration profile extends a distance of 120 mu m into the electrolyte, which verifies that the nominal overpotential reduction from using alkaline electrolyte originates from the Nernst potential of the pH gradient layer at the cathode/electrolyte interface. The CO2-OH- neutralization reaction and the pH gradient layer still persist, albeit to a reduced extent, at CO2 reduction current densities up to 150 mA/cm(2).
Computational and experimental studies have been performed to investigate the effects of current collector design on the performance of microfluidic fuel cell (MFC) with flow-through porous electrodes. Characteristics of electron transport in MFC with flow-through porous electrodes are investigated based on a three-dimensional computational model. The lateral electron transport in the porous electrode is found to encounter high resistance. To improve the cell performance, influences of different current collector design parameters on the transport resistances are examined. Physical origins for the influences of different design parameters are also discussed. The results demonstrate that current collector position is the most influential factor due to the non-uniform flow rate distribution. In the experimental study, cell performances revealed maximum power density when current collectors were located at the high flow rate region. An increase of 61% was observed when the current collectors moved from the conventional exposed ends of the electrodes to the high flow rate regions in the electrode active area. Based on the results, some general rules are set for the current collector designs of MFCs.
In this work, a simulation study of MFC based on counter-flow structure was implemented. The results indicate that the counter-flow channel enables effective suppression on fuel diffusion mixing thus intensifies fuel utilization. However, the potential loss caused by resistance of electrolyte hinder the performance significantly. Decrease in flow rate leads to enhancement on fuel utilization in spite of improving diffusion loss at the same time. (C) 2014 The Authors. Published by Elsevier Ltd. This is an open access article under the CC BY-NC-ND license (http://creativecommons.org/licenses/by-nc-nd/3.0/).
Utilizing CO2 in an electro-chemical process and synthesizing value-added chemicals are amongst the few viable and scalable pathways in carbon capture and utilization technologies. CO2 electro-reduction is also counted as one of the main options entailing less fossil fuel consumption and as a future electrical energy storage strategy. The current study aims at developing a new electrochemical platform to produce low-carbon e-biofuel through multifunctional electrosynthesis and integrated co-valorisation of biomass feedstocks with captured CO2. In this approach, CO2 is reduced at the cathode to produce drop-in fuels (e.g., methanol) while value-added chemicals (e.g., selective oxidation of alcohols, aldehydes, carboxylic acids and amines/amides) are produced at the anode. In this work, a numerical model of a continuous-flow design considering various anodic and cathodic reactions was built to determine the most techno-economically feasible configurations from the aspects of energy efficiency, environment impact and economical values. The reactor design was then optimized via parametric analysis.
lA comprehensive multi-physics two-phase flow model was developed.lThe inhomogeneous GDL deformation was numerically studied.lEffective diffusion coefficient and electrical conductivity were analyzed.lTrade-off of electrons and mass transport determines the optimal compression ratio.lThe model could predict the optimal compression ratio of various carbon papers. Gas diffusion layers play a critical role in the operation of proton exchange membrane fuel cells. As the most compressible component in proton exchange membrane fuel cells, the non-uniform deformation mainly occurs on the interface between bipolar plates and gas diffusion layers caused by the special channel-rib geometry of the flow field, which results in a non-uniform variation of physical properties of gas diffusion layers, such as porosity, effective electrical conductivity, and gas diffusivity, consequently affects the cell performance. In this paper, a two-dimensional, across-the-channel, multi-physics and two-phase flow model based on the spherical agglomerate assumption is developed to investigate the complicated relationships between the non-uniform deformation and variation of physical properties of the gas diffusion layers, as well as the cell performance. A modified diffusion coefficient is introduced to describe the effect of the variation of species concentration on the effective diffusion coefficient based on the Bruggeman formula. Simulation results show that an optimal cell performance can be achieved by balancing the variation of porosity, effective electrical conductivity, and effective gas diffusion coefficient with respect to different degrees of deformation of gas diffusion layers.
In this paper, a novel aluminizing pretreatment method was developed to enable a strong adhesion of Al2O3 layers on the aluminum-free stainless steel substrate for the application of Fischer–Tropsch microreactor. The Co/Re/W/Al2O3 catalyst coated on a microreactor fabricated with 316L stainless steel was tested for Fischer–Tropsch synthesis and showed a good activity. Ultrasonic vibration test results showed that the average weight loss of the coating on the aluminized substrate was only one-eighth of that on the untreated plate. A high C5+ productivity of 759.2mgC5+/gcath was achieved at 220°C and 20atm under a gas hourly space velocity (GHSV) of 21,000h−1, and C5+ selectivity increased with decreasing CO conversion rate. Product distribution shifted toward short-chain hydrocarbons and hydrogenated products at high operation temperatures. Catalyst coating thickness was also optimized to inhibit CH4 production and adjust the olefin/paraffin ratio. •Novel aluminizing method was developed for Fischer–Tropsch microreactor.•The Co/Re/W/Al2O3 catalyst on 316L stainless steel showed a good activity.•A high C5+ productivity was achieved.•Catalyst coating thickness was also optimized.
•A novel multicriteria (MCDM) framework using AHP and q-ROLPBM operator is presented.•We incorporate stakeholders’ degree of pessimistic and optimistic rate for all criteria.•Environmental and economic are the most crucial dimensions followed by social and technical ones.•e-fuel and e-biofuel are found to be the two top ranked production pathways.•Findings are valuable for policy/investment development for sustainable fuel production. It is widely believed that alternative low carbon fuels (ALCF) can be instrumental in achieving the transportation sector’s decarbonization goal. Unlike conventional fossil-based fuels, ALCF can be produced through a combination of different chemical processes and feedstocks. The inherent complexity of the problem justifies the multi-criteria decision-making (MCDM) approach to support decision-making in the presence of multiple criteria and data uncertainty. In this paper, we propose a novel stakeholder participation-based MCDM framework integrating experts' perspectives on ALCF production pathways using the analytics hierarchy process (AHP) and the q-rung orthopair linguistic partition Bonferroni mean (q-ROLPBM) operator. The key merit of our approach lies in treating criteria of different dimensions as heterogeneous indicators while considering the mutual influence between criteria within the same dimension. The proposed framework is applied to evaluate four ALCF production pathways against 13 criteria categorised under economic, environmental, technical, and social dimensions for the case of the United Kingdom (UK). Our analysis revealed the environmental and the economic dimensions to be the most important, followed by the social and technical evaluation dimensions. The e-fuel followed by the e-biofuel are found to be the two top-ranked production pathways that utilise the electrochemical reduction process and its combination with anaerobic digestion. These findings, along with our recommendations, provide decision-makers with guidelines on ALCF production pathway selection and formulate effective policies for investment.
Novel urchin-like microparticles with photocatalytic activity and magnetically separable properties were prepared by a layer-by-layer assembly process. Photocatalytic degradation was investigated using two types of mercury vapor lamps: an ozone generating lamp emitting at both 254 nm and 185 nm as well as a germicidal lamp emitting at 254 nm only. This novel photocatalyst demonstrated superior photocatalytic activity in the mineralization of phenol under UVC illumination compared with the commercial P25, Degussa TiO2, especially in repeated usage. Importantly, this photocatalyst can be quickly separated for reuse simply by using a magnet. The merits of 3D spiny nanostructured TiO2 microparticle photocatalysts are high specific surface area, good permeability, reduced charge recombination rate and high catalytic activity while the incorporation of magnetically separable properties enables rapid and easy retrieval of the suspended photocatalyst after use.
•Hollow carbon fibers derived from natural cotton was successfully prepared by pyrolysis method.•TiO2 nanorods immobilized on carbon fibers by a facile hydrothermal method showed high photocatalytic activity.•The enhancement was due to the reduced band gap, improved dye adsorption capacity and effective electron–hole separation. In this study, TiO2 nanorods were successfully immobilized on carbon fibers by a facile pyrolysis of natural cotton in nitrogen atmosphere followed by a one-pot hydrothermal method. Carbonized cotton fibers (CCFs) and TiO2-CCFs composites were characterized using field-emission scanning electron microscope (FE-SEM), transmission electron microscopy (TEM), X-ray photoelectron spectroscopy (XPS), Raman spectroscopy, X-ray diffractometer (XRD), diffuse reflectance UV–vis spectroscopy (DRS) and photoluminescence (PL) spectroscopy. Results implied that the band gap narrowing of TiO2 was achieved after integration of CCFs. Dye adsorption isotherm indicated that the maximum dye adsorption capacity (qm) of CCFs-1000 (13.4mg/g) was 2 times higher than that of cotton fibers and qm of TiO2-CCFs-1000 (9.0mg/g) was 6–7 times higher than that of TiO2 nanorods. Photocatalytic activity of TiO2 nanorods prepared with 3mL Ti(OBu)4 showed the highest photocatalytic activity. TiO2-CCFs-1000 exhibited higher activity than TiO2 immobilized on CCFs-400, CCFs-600 and CCFs-800. Good photostability of TiO2-CCFs-1000 was found for dye degradation under visible light irradiation. The enhancement of photocatalytic dye degradation was due to the high adsorptivity of dye molecules, enhanced light adsorption and effective separation of electron–hole pairs. This work provides a low-cost and sustainable approach to immobilize nanostructured TiO2 on carbon fibers for environmental remediation.
•A 3D model for MFC with flow-through porous electrodes is developed.•Characteristics of electron transport in MFC are investigated.•Effects of different current collector design parameters are examined.•Design principles of current collectors are derived.•Experimental investigation is performed to verify the key findings. Design optimization of current collectors has been performed to reduce the significant ohmic resistance observed in microfluidic fuel cell (MFC) with flow-through porous electrodes. A three-dimensional computational model is developed to investigate the electron transport characteristics in the porous electrodes, where lateral electron transport is found to encounter high resistance. Influences of different current collector design parameters on the transport resistances are examined and analyzed. The modeling results indicate that current collector position is the most influential factor due to the non-uniform flow rate distribution. Optimal current collector position is located at the high flow rate region instead of the conventional exposed end of the porous electrode. Experimental studies are performed to support the modeling analysis. The experimental results demonstrate that the optimized current collector position can boost the maximum power density by 61%. This study highlights the significance of the current collector design in achieving high performance MFC with flow-through porous electrodes. Based on the results, some general rules have been set for the current collector designs in this energy system, which can provide useful guidance for the future development of MFC.
A hybrid aluminum/hydrogen/air cell system is developed to solve the parasitic hydrogen-generating problem in an alkaline aluminum/air battery. A H2/air fuel cell is integrated into an Al/air battery so that the hydrogen generated by the parasitic reaction is utilized rather than wasted. A systematic study is conducted to investigate how the parasitic reaction and the added H2/air cell affect the performance of the aluminum/air battery. The aluminum/air sub-cell has an open circuit voltage of 1.45 V and the hydrogen/air sub-cell of 1.05 V. The maximum power density of the entire hybrid system increases significantly by ∼20% after incorporating a H2/air sub-cell. The system maximum power density ranges from 23 to 45 mW cm−2 in 1–5 M NaOH electrolyte. The hybrid system is adaptable in concentrated alkaline electrolyte with significantly improved power output at no sacrifice of its overall efficiency. [Display omitted] •A self-sustaining hybrid cell system consisting of an Al/air battery and a H2/air fuel cell.•Effectively utilizing the H2 generated by the parasitic reaction rather than dumping it.•Investigating the Al parasitic reaction with mixed potential theory and practical experiments.•The system is adaptable in concentrated alkaline electrolytes without efficiency degradation.•Room temperature operation with system power density higher than direct alcohol fuel cells.
In this work, mesostructured KIL-2 silica materials were synthesized and functionalized by impregnation with two different amino sources, polyethylenimine (PEI) and Tetraethylenepentamine (TEPA), for their application to low temperature CO2 capture. Infrared spectroscopy verified that amino groups were successfully incorporated on the surface of the silica support and within its interparticle porosity. N2 sorption analysis of the synthesized silica materials showed a decrease in surface area and total pore volume as a result of the impregnation with the amine groups. The CO2 capture capacity of prepared sorbents was evaluated by CO2 adsorption/desorption isotherms at 25°C and 90°C. The highest sorption capacity was achieved at 90°C for KIL-2-TEPA material (4.35 mmol/g ads), which is significantly higher than values previously reported in the literature for similar type of disordered mesopore structure materials under similar conditions.
•A hybrid machine learning strategy is developed for complex chemical processes.•Continuum lumping kinetic is embedded into the neural network framework.•NSGA-II is used for multi-objective optimisation of the hydrocracking process. Catalytic chemical processes such as hydrocracking, gasification and pyrolysis play a vital role in the renewable energy and net zero transition. Due to the complex and non-linear behaviours during operation, catalytic chemical processes require a powerful modelling tool for prediction and optimisation for smart operation, speedy green process routes discovery and rapid process design. However, challenges remain due to the lack of an effective modelling and optimisation toolbox, which requires not only a precise analysis but also a fast optimisation. Here, we propose a hybrid machine learning strategy by embedding the physics-based continuum lumping kinetic model into the data-driven artificial neural network framework. This hybrid model is adopted as the surrogate model in the multi-objective optimisation and demonstrated in the benchmarking of a hydrocracking process. The results show that the novel hybrid surrogate model exhibits the mean square error less than 0.01 by comparing with the physics-based simulation results. This well-trained hybrid model was then integrated with non-dominated-sort genetic algorithm (NSGA-II) as the surrogate model to evaluate and optimise the yield and selectivity of the hydrocracking process. The Pareto front from the multi-objective optimisation was able to identify the trade-off curve between the objective functions which is essential for the decision-making during process design. Our work indicates that adopting the hybrid machine learning strategy as the surrogate model in the multi-objective optimisation is a promising approach in various complex catalytic chemical processes to enable an accurate computation as well as a rapid optimisation. [Display omitted]
Aluminum-ion batteries, emerging as a promising post-lithium battery solution, have been a subject of increasing research interest. Yet, most existing aluminum-ion research has focused on electrode materials development and synthesis. There has been a lack of fundamental understanding of the electrode processes and thus theoretical guidelines for electrode materials selection and design. In this study, by using density functional theory, we for the first time report a first-principles investigation on the thermodynamic and kinetic properties of aluminum intercalation into two common TiO2 polymorphs, i.e., anatase and rutile. After examining the aluminum intercalation sites, intercalation voltages, storage capacities and aluminum diffusion paths in both cases, we demonstrate that the stable aluminum intercalation site locates at the center of the O-6 octahedral for TiO2 rutile and off center for TiO2 anatase. The maximum achievable Al/Ti ratios for rutile and anatase are 0.34375 and 0.36111, respectively. Although rutile is found to have an aluminum storage capacity slightly higher than anatase, the theoretical specific energy of rutile can reach 20.90 Wh kg(-1), nearly twice as high as anatase (9.84 Wh kg(-1)). Moreover, the diffusion coefficient of aluminum ions in rutile is 10(-9) cm(2) s(-1), significantly higher than that in anatase (10(-20) cm(2) s(-1)). In this regard, TiO2 rutile appears to be a better candidate than anatase as an electrode material for aluminum-ion batteries.
Photoelectrochemical (PEC) water splitting offers a promising way to produce hydrogen and harvest solar energy, however, its low efficiency has made it less economically attractive than other hydrogen production methods. Herein we present a numerical model of PEC cells considering quasi-fermi level splitting and interfacial kinetics to understand the charge transfer process and explore the approaches to increase the energy conversion efficiency. The non-linear change of photocurrent with light intensity under concentrated illumination is for the first time captured by a model. Based on the model, the operation regions of a PEC cell are mapped. Pathways to further promote the energy efficiency of PEC are proposed from the aspect of kinetics and thermodynamics. A new method that enables a precise evaluation of the theoretical boundaries of energy conversion efficiency of photocatalysts is developed taking into account the thermodynamics barrier. [Display omitted] •First PEC model considering quasi-fermi level splitting and interfacial kinetics.•Nonlinear photocurrent response under concentrated sunlight captured and explained.•Theoretical maximum hydrogen yield of photoelectrode is reevaluated.
This study numerically investigates liquid water dynamics in a novel hybrid sinusoidal flow channel of a proton exchange membrane fuel cell (PEMFC). The two-phase flow is examined using a three-dimensional, transient computational fluid dynamics (CFD) simulation employing the coupled level set and volume of fluid (VOF) method. Simulations for hybrid and non-hybrid sinusoidal flow channels, including a straight flow channel, are compared based on their water exhaust capacities and pressure drops. Additionally, the effects of inlet gas velocity, wall wettability, and droplet interaction in the flow channel on the dynamic behaviour of liquid water are investigated. Results reveal that the novel hybrid sinusoidal channel designs are consistent in terms of quicker water removal under varying hydrophilic wall conditions. Also, it is found that the liquid surface coverage, detachment, and removal rate depends on droplet proximity to the walls, inlet gas velocity, and wall contact angle. Also, the time a droplet makes contact with the side walls affect the discharge time. Additionally, there is an improvement in the gas velocity magnitude and vertical component velocity across the hybrid sinusoidal channel designs. Therefore, the unique geometric configuration of the proposed hybrid design makes it a viable substitute for water management in PEMFC applications.
Proton exchange membrane (PEM) fuel cells with an off-gas recirculation anode (ORA) or dead-ended anode (DEA) are widely adopted in engineering. However, those two hydrogen flow arrangements may cause anodic water and nitrogen accumulation in comparison with the flow-through anode (FTA) mode, which causes significant performance degradation. In this paper, a two-dimensional cold-start model is developed with detailed consideration of water phase changes and the nitrogen crossover phenomenon. A simplified electrochemical module is built to calculate the current density distribution in the model. The simulation results are consistent with the experimental data at both sub-zero temperatures and normal operating temperatures. The effects of hydrogen flow arrangements, flow configurations, and startup strategies are investigated during startup process from subzero to normal operating temperatures. Much less ice is generated in counter-flow cases than in co-flow cases during constant current operation. A relatively lower startup voltage can effectively shorten the cold-start process and enhance the cold-start capacity for the PEM fuel cell. The ORA mode has the best hydrogen flow arrangement due to its general abilities, including higher hydrogen utilization efficiency, higher anodic nitrogen tolerance, better output performance and better startup capability. (C) 2020 Hydrogen Energy Publications LLC. Published by Elsevier Ltd. All rights reserved.
In this study, we explore the feasibility of laser as source of photons for plasmonic heating of metal nanoparticles. Au decorated TiO2 nanoparticles with different Au wt.% loading were prepared using deposition-precipitation method and their physical and optical properties were characterized by X-Ray diffraction (XRD), Diffuse reflectance spectra (DRS) and Raman spectroscopy. The enhancement of the optical properties of Au plasmonic nanoparticles arises from localized surface plasmon resonance (LSPR) effect achieved under 532 nm laser irradiation. Additionally, the photothermal performance of Au/TiO2 nanofluid was tested compared with other nanofluids under visible laser irradiation. The results revealed that the temperature of Au/TiO2 nanofluid was significantly higher compared to that of bare TiO2 and pure milli-Q water, attributing to the plasmonic excitation of Au nanoparticles under laser irradiation.
Graphene oxide (GO) induced enhancement of elastomer properties showed a great deal of potential in recent years, but it is still limited by the barrier of the complicated synthesis processes. Stereolithography (SLA), used in fabrication of thermosets and very recently in "flexible" polymers with elastomeric properties, presents itself as simple and user-friendly method for integration of GO into elastomers. In this work, it was first time demonstrated that GO loadings can be incorporated into commercial flexible photopolymer resins to successfully fabricate GO/elastomer nanocomposites via readily accessible, consumer-oriented SLA printer. The material properties of the resulting polymer was characterized and tested. The mechanical strength, stiffness, and the elongation of the resulting polymer decreased with the addition of GO. The thermal properties were also adversely affected upon the increase in the GO content based on differential scanning calorimetry and thermogravimetric analysis results. It was proposed that the GO agglomerates within the 3D printed composites, can result in significant change in both mechanical and thermal properties of the resulting nanocomposites. This study demonstrated the possibility for the development of the GO/elastomer nanocomposites after the optimization of the GO/"flexible" photoreactive resin formulation for SLA with suitable annealing process of the composite in future.
As an emerging technology for CO2 utilization, electrochemical CO2 reduction reaction (ECO2RR) systems incorporating gas diffusion electrodes (GDE) have the potential to transform CO2 to valuable products efficiently and environment-friendly. In this work, a two-dimensional multiphase model capturing the details of the catalyst layer in a GDE that produces formate with byproducts is established and quantitatively validated against experimental data. This model is capable of describing the mixture gas and aqueous species transportation, electron conduction processes, and a series of interrelated chemical and electrochemical reactions. Specific electrical energy consumption (SEEC) and product yield (PY) have been introduced and used to examine the GDE scalability and evaluate the system performance. The results predict the optimal values for applied cathode potential and catalyst loading and porosity. The effect of inlet gas composition and velocity is also evaluated. Moreover, this study predicts that the GDE is scalable as it retains a stable performance as its geometrical surface area varies. This model together with the simulation findings contributes to the improved understanding of GDE-based CO2 conversion as needed for the future development toward successful industrial applications.
High-temperature co-electrolysis of CO2/H2O through the solid oxide electrolysis cells (SOECs) is a promising method to generate renewable fuels and chemical feedstocks. Applying this technology in flexible scenario, especially when combined with variable renewable powers, requires an efficient optimisation strategy to ensure its safety and cost-effective in the long-term operation. To this purpose, we present a hybrid simulation method for the accurate and fast optimisation of the co-electrolysis process in the SOECs. This method builds multiphysics models based on experimental data and extends the database to develop the deep neural network and genetic algorithm. In the case study, thermal-neutral condition (TNC) is set as the optimisation target in various operating conditions, where the SOEC generates no waste heat and needs no auxiliary heating equipment. Small peak-temperature-gradient (PTG) inside the SOEC is found at the TNC, which is vital to prevent thermal failure in the operation. For the cell operating with 1023 K and 1123 K of inlet gas temperatures, the smallest PTGs reach 0.09 and 0.31 K mm-1 at 1.13 and 1.19 V, respectively. Finally, a 4-D map is presented to show the interactions among the applied voltage, required power density, inlet gas composition, and temperature under the TNC. The proposed method can be flexibly modified based on different optimisation targets for various applications in the energy sector.
Vapor-feed microfluidic fuel cell (VF-MFC) has various advantages against the conventional liquid-feed microfluidic fuel cell, such as simpler fluidic management, higher fuel utilization, flow rate insensitiveness, and so on. To better understand the mechanisms behind its superiority and to further optimize its performance, a 3D isothermal numerical model has been developed in this work. The computational results agree very well with the previous and present experimental data, proving the validity of the current model for the VF-MFC simulation. Through this model, it is found that the dissolved fuel in the VF-MFC is well-controlled within a thin boundary layer nearby the anode catalyst surface, which can not only satisfy the demand of anode oxidation reaction but also greatly alleviate the wastage of fuel. In this manner, the VF-MFC can achieve satisfactory power output and high fuel utilization at the same time. In addition, the boundary layer effect on electrolyte flow rate can keep the fuel concentration in the thin layer relatively stable at different flow rates, which may be the reason behind the insensitiveness of VF-MFC performance to electrolyte flow rate. To further improve its power output and fuel efficiency, effects of the fuel evaporation area and the anode open ratio have also been thoroughly investigated with the present model. It is found that an evaporation-reaction area ratio of 11.1 is sufficient for the present VF-MFC, while a smaller fuel evaporation area can lead to improved fuel utilization at the expense of lower power output. To improve both the fuel utilization and power output, the electrode area towards the channel outlet is increased while keeping the vapor entrance area constant, i.e. the anode open ratio is reduced. By this strategy, the VF-MFC can achieve 48% higher power output and elevated fuel utilization from 27.5% to 41.8%, when an anode open ratio of 1:3 is adopted. (C) 2017 Elsevier Ltd. All rights reserved.
Porous energy materials are essential components of many energy devices and systems, the development of which have been long plagued by two main challenges. The first is the 'curse of dimensionality', i.e. the complex structure-property relationships of energy materials are largely determined by a high-dimensional parameter space. The second challenge is the low efficiency of optimisation/discovery techniques for new energy materials. Digitalisation of porous energy materials is currently being considered as one of the most promising solutions to tackle these issues by transforming all material information into the digital space using reconstruction and imaging data and fusing this with various computational methods. With the help of material digitalisation, the rapid characterisation, the prediction of properties, and the autonomous optimisation of new energy materials can be achieved by using advanced mathematical algorithms. In this paper, we review the evolution of these computational and digital approaches and their typical applications in studying various porous energy materials and devices. Particularly, we address the recent progress of artificial intelligence (AI) in porous energy materials and highlight the successful application of several deep learning methods in microstructural reconstruction and generation, property prediction, and the performance optimisation of energy materials in service. We also provide a perspective on the potential of deep learning methods in achieving autonomous optimisation and discovery of new porous energy materials based on advanced computational modelling and AI techniques.
This study aims to investigate how multiple parameters affect the two-phase flow in compressed gas diffusion layer (GDL). A stochastic model is adopted to reconstruct the GDL microstructures. Solid mechanics simulations on the reconstructed GDL microstructures are performed, based on the finite element method (FEM). Various pore morphologies and distributions of compressed GDLs are observed. Two-phase flow in GDL is simulated using a volume of fluid (VOF) model. Corner droplet (on the GDL surface) and water flow (emerging from GDL bottom) are considered. It is found that two-phase flow in the GDL is highly influenced by compression, fiber diameter, porosity, and GDL thickness. The results indicate that a larger fiber diameter or higher porosity contributes to the water transport due to larger average pore size. Furthermore, water removal from a thicker GDL is more difficult, whereas water transport in the lower part of a compressed thick GDL is easy. (C) 2019 Hydrogen Energy Publications LLC. Published by Elsevier Ltd. All rights reserved.
This Review provides an overview of electrochemical techniques that are implemented in addressing gaseous CO2 towards the synthesis of a particular fuel (i.e. formic acid). The electrochemical reaction mechanism, as well as the advancement of electrodes, catalyst materials, and reactor designs are reviewed and discussed. To date, the electrolytic cell is the dominant reaction site and, based on which, various catalysts have been proposed and researched. In addition, relevant work regarding reactor design optimization for the purpose of alleviating restrictions of the current CO2 electrochemical reduction system are summarized, including low reactant-transfer rate, high reaction overpotential, and low product selectivity. The use of microfluidic techniques to build microscale electrochemical reactors is identified to be highly promising to largely increase the electrochemical performance. Finally, future challenges and opportunities of electrochemical reduction of CO2 are discussed.
Regenerative fuel cells are a potential candidate for future energy storage, but their applications are limited by the high cost and poor round-trip efficiency. Here we present a switchable pH-differential unitized regenerative fuel cell capable of addressing both the obstacles. Relying on a membraneless laminar flow-based design, pH environments in the cell are optimized independently for different electrode reactions and are switchable together with the cell process to ensure always favorable thermodynamics for each electrode reaction. Benefiting from the thermodynamic advantages of the switchable pH-differential arrangement, the cell allows water electrolysis at a voltage of 0.57 V, and a fuel cell open circuit voltage of 1.89 V, rendering round-trip efficiencies up to 74%. Under room conditions, operating the cell in fuel cell mode yields a power density of 1.3 W cm−2, which is the highest performance to date for laminar flow-based cells and is comparable to state-of-the-art polymer electrolyte membrane fuel cells. •A pH-differential technique breaking the thermodynamic limitation from window of water.•High output power density and round-trip efficiency recorded.•Electrode potentials moved closer to equilibrium status and hence reduce loss.•Heat generation and interfacial neutralization well controlled.•Feasible cyclic operation in the novel microfluidic reactor.
•A data-driven model for multi-condition dynamic operations is proposed.•The proposed method has a good performance in predicting cold-start processes.•An optimization scheme for operating conditions of cold start is proposed.•The optimized start-up strategy can reduce start-up time by 26.7%. The reliability of proton exchange membrane fuel cell (PEMFC) tightly depends on the suitable operating conditions during dynamic operations. This study proposes an optimization framework to determine the optimal control strategy for PEMFC cold starts underpinned by a novel artificial intelligence method, to improve cold-start capacity and shorten the start-up time. The effects of constant and dynamic currents on PEMFC cold starts under various initial temperatures are studied. The numerical results from a developed PEMFC dynamic model show that the constant current slope strategy (CCSS) is more efficient than the constant current strategy (CCS) in respect of the cold-start time. In the CCSS study, a too-large current slope can lead to a voltage undershoot and then cause a failed cold start, but a too-small current slope can result in a long start-up process in the investigated range of the operating conditions. A data-driven model is developed for dynamic prediction and real-time optimization during the cold start by a semi-recurrent sliding window (SW) method coupled with artificial neural networks (NN) with the simulation data. Based on this NN-SW model, the specific safety–critical operating condition curve under the CCSS has been identified. A real-time adaptive control strategy (RACS) is further proposed to optimize the operating current during the PEMFC cold starts with various initial temperatures. Compared to the optimal CCSS, RACS proves to be more robust and efficient for PEMFC cold-start startups. Based on RACS, the start-up time for an initial temperature of −20 °C can be cut down by 26.7%. Furthermore, the ice predictions by the NN-SW model are also tested and the results are satisfying with an average R2 = 0.9773.
Photocatalytic CO2 conversion into usable chemical fuels is considered as an ideal way to tackle problems such as energy shortage and global warming simultaneously. In this "kill two birds with one stone" approach, CO2 is used as feedstock and abundant solar light as energy source. For this purpose, a photocatalytic micro-reactor was designed in order to overcome problems of conventional photo-reactors including low surface-area-to-volume ratio, poor mass and photon transfer. Common materials such as Fluorine-doped Tin oxide (FTO) glass, Polymethyl methacrylate (PMMA) and surlyn that widely used in photoelectrochemical and solar cells were employed for the fabrication of the reactor. The feasibility and performance of the proposed reactor was tested in the challenging case of photocatalytic CO2 reduction on TiO2 thin film. The experimental results confirmed that one of the main products of CO2 reduction was methanol. Maximum methanol concentration reached 162 nM at a flow rate of 120 nL/min. (C) 2017 The Authors. Published by Elsevier Ltd.
Fuel cell vehicles (FCVs) have shown the potential of commercialization in recent years. The concerns on the startup ability of proton exchange membrane (PEM) fuel cell stack from subfreezing temperature have risen. The hydrogen oxygen catalytic reactions assisted cold start method is developed and analyzed in this study. It utilizes a small amount of hydrogen/ air mixture to react at low temperature in the catalyst layers (CLs) through platinum catalyst. The interactions between this assisted method and various startup modes are the major issue to be discussed. Anode catalytic reaction with air mole fraction higher than 16% is effective to assist a 30-cell stack starting from 25 degrees C within 13 s in maximum power mode. However, cathode catalytic reaction cannot sustain a successful startup. The anode humidification effect plays an important role to reduce the stack resistance, and to increase the inherent heat generation rate. In maximum power mode and high current density constant power mode, anode catalytic reaction assisted cold start can be achieved within 10-20 s from 40 degrees C. Anode air mole fraction must be higher than 18% to ensure the successful cold start in these two modes. For constant power mode, the operating power must be lower than 12W per cell. In constant current mode, when the current density is low, there would be less demand for anode catalytic reaction to achieve successful startup from 40 degrees C, indicating that lower current density operations have better survivability in low temperature. Nevertheless, much longer start duration is required for lower operating current. Generally, high current density operating mode with high air mole fraction is a more practical and energy efficient cold start strategy, as the startup time can be reduced significantly. Cold start from about 20 degrees C without ice accumulation is feasible using this method, which may have reduced concern about degradation. Increasing the volume of CL (porosity and thickness) also helps reduce the ice formation. Copyright (C) 2015, Hydrogen Energy Publications, LLC. Published by Elsevier Ltd. All rights reserved.
In part A of this review, we have introduced the progress of the research and the application status of unitized regenerative proton exchange membrane fuel cells. In addition to this Proton Exchange Membrane (PEM)-based Unitized Regenerative Fuel Cell (URFC), other URFC technologies with different electrolytes have also been reported in the literature, which form the basis for emphasis in this part of the review. Unitized Regenerative Alkaline Fuel Cells (UR-AFC) have long been utilized for aerospace applications, while the recent development of Anion Exchange Membrane (AEM) has stimulated their further development, especially on the AEM-based UR-AFCs. Vast research works have been reported on the bifunctional oxygen catalyst development, while the latest UR-AFC prototypes are also being briefly introduced. Despite their potential cost-efficiency and better reactivity, cell performance and round-trip efficiency of the current UR-AFCs are still lower than their PEM-based counterparts. Unitized regenerative solid oxide fuel cell, which is more commonly cited as Reversible Solid Oxide Fuel Cell (RSOFC), is a high-temperature URFC technology with superior performance and reversibility. Review works conducted on this type of URFC are separated into two categories, that is, RSOFC with oxygen ion conducting electrolyte and RSOFC with proton ion conducting electrolyte. Despite the highest efficiency among various URFC technologies, the application of RSOFCs, however, is restricted by their limited long-term stability and poor cycle ability. Unitized regenerative microfluidic fuel cell, also referred to as the reversible microfluidic fuel cell, is a newly-emerging URFC research trend which benefits a lot from its membraneless configuration. However, limited research works have been conducted on this new technology.
Microfluidic fuel cell (MFC) is a promising fuel cell type because its membraneless feature implies great potential for low-cost commercialization. In this study, an energy and exergy analysis of MFC is performed by numerical simulation coupling computational fluid dynamics (CFD) with electrochemical kinetics. MFC system designs with and without fuel recirculation are investigated. The effects of micropump efficiency, fuel flow rate and fuel concentration on the MFC system performance are evaluated. The results indicate that fuel recirculation is preferred for MFC to gain higher exergy efficiency only if the efficiency of the micropump is sufficiently high. Optimal cell operating voltage for achieving the highest exergy efficiency can be obtained. Parasitic effect will cause a significant reduction in the exergy efficiency. An increase in the fuel concentration will also lead to a reduction in the exergy efficiency. Increasing the fuel flow rate in a MFC with fuel recirculation will cause a fluctuating variation in the exergy efficiency. On the other hand, in a one-off MFC system, the exergy efficiency decreases with increasing fuel flow rate. The present work enables better understanding of the energy conversion in MFC and facilitates design optimization of MFC. Copyright (C) 2013, Hydrogen Energy Publications, LLC. Published by Elsevier Ltd. All rights reserved.
•A hybrid deep learning model for FCC performance is developed.•The hybrid model reduces the prediction error by up to 9%.•The hybrid model outperformed the DNN model with limited data availability.•The hybrid model shows a significantly greater generalization ability. Fluid catalytic cracking (FCC) is one of the most important processes in the renewable energy as well as petrochemical industries. The prediction and understanding of the FCC performance in a real industrial environment is still challenging, as this is a highly complex process affected by many extremely non-linear and interrelated factors. In this paper, a novel hybrid predictive framework for FCC is developed by integrating a data-driven deep neural network with a physically meaningful lumped kinetic model, powered by orders of magnitude greater number of high-quality data from a modem automated FCC process. The results show that the novel hybrid model exhibits best predictions with regards to all the evaluation criteria such as Mean Absolute Percentage Error, Pearson coefficient, and standard deviation. It indicates that the hybrid data-driven deep learning with mechanistic kinetics model creates a better approach for fast prediction and optimization of complex reaction processes such as FCC.
Graphene, composed of single-layered sp2 graphite, with its superb physical and chemical properties, has attracted scientists in different areas, including electronics, medicine, and chemicals. Its applications in green energy were extensively studied in the past two decades. Results from different studies show that graphene-based products with different structures (2D and 3D graphene) can effectively enhance green energy conversion and storage compared to traditional energy materials like metal and metal oxides. This review focuses on the historical development of graphene, the variation of graphene products, and mainstream researches in graphene-based green energy applications carried out in recent years, such as fuel cell, solar cell, lithium-ion batteries (LIBs), supercapacitor, dye-sensitized solar cell (DSSC), and photoelectrochemical water-splitting cell. The development of graphene-based materials integrated with different 3D printing technology and the application of their products in green energy are also discussed together with a forecast on the development of graphene-based materials in the future. •Development of raw graphene (bottom-up and top-down) is highlighted.•Various modifications of graphene-based materials for energy applications are reviewed.•Use of graphene-based materials in green energy electrodes is discussed.•Recent development of graphene in green energy via 3D printing is introduced.•Perspective on graphene energy materials development is given.
This paper numerically investigates a portable fuel processor, i.e., micro-scale auto-electrolytic cell (AEC), for on-site hydrogen production in an economical, spontaneous and controllable manner. The AEC in this study consists of a galvanic couple of magnesium and steel in sodium chloride solution. A single Laplace's equation with boundary conditions determined from electrode reaction kinetics is solved for the potential inside the AEC. A dynamic mesh model based on arbitrary Lagrangian–Eulerian description is applied to track the moving boundary of the dissolving magnesium anode. Based on the model, the spatio-temporal distributions of potential, current density, hydrogen generation rate and other important parameters associated with the AEC are obtained. A great enhancement of hydrogen generation rate is found achievable by miniaturizing the AEC. In addition, parametric analyses are also performed focusing on important geometric factors. The study suggests that it would be better to arrange a number of micro-scale AEC units together to attain a desired total hydrogen output. The present study contributes to a better understanding of the hydrogen generation characteristics of AECs, hence facilitates their future development. The developed model can also serve as a useful tool to study other similar electrochemical systems. ► We model an auto-electrolytic process for hydrogen production. ► A good performance is demonstrated with micro-scale auto-electrolytic cell. ► Distributions of important parameters inside the cell are analyzed. ► The cell geometry is optimized to achieve a better performance.
Energy storage capacity has been a major limiting factor in pursuit of increasing functionality and mobility for portable devices. To increase capacity limits, novel battery designs with multi-electron redox couples and increased voltages have been listed as a priority research direction by US Department of Energy. This study leverages the benefits of microfluidics technology to develop a novel type of mixed-pH media aluminium-air cell, which incorporates the advantages of aluminium's trivalence and mixed-pH thermodynamics. Experimentally, an open circuit potential of 2.2V and a maximum power density of 176 mW/cm2 are measured from the new cell, which are respectively 37.5% and 104.6% higher compared to a conventional alkaline aluminium-air cell.
This paper presents a model integrating computational fluid dynamics (CFD) with electrochemical kinetics for predicting the performance of laminar flow-based fuel cell (LFFC). In the modeling analysis, we study the effect of parasitic current caused by the mixing of fuel and oxidant under critical operating conditions, which are between the ideal conditions without any crossover effect and the cell failure conditions due to too serious parasitic effects. The results show that the parasitic effect would cause a significant deviation of the cell performance compared with the ideal process when the LFFC is working under various critical conditions. The results also indicate that the parasitic current is an important factor for designing an efficient LFFC with high fuel utilization and high power density. (C) 2011 Elsevier Ltd. All rights reserved.
In recent years, 3D printing has gained a great deal of attention in the energy field, with numerous reports demonstrating its application in fabrication of electrochemical devices. The near-complete freedom of design offered by 3D printing technologies make them very appealing, since complex 3D parts can be directly fabricated. However, its application in photochemistry and solar energy harvesting remains, so far, an uncharted territory. In this work, a photoactive monomer was incorporated into commercially available 3D printing resin, which was subsequently used to successfully fabricate 3D photosensitizing structures for singlet oxygen generation. Results indicate that the SLA fabricated small-scale (0.1 ml) photoactive continuous flow reactor shows activity in singlet oxygen synthesis reaction under visible light irradiation (420 nm).
A microfluidic fuel cell is a low cost, easily fabricated energy device and is considered a promising energy supplier for portable electronics. However, the currently developed microfluidic fuel cells that are fed with hydrocarbon fuels are confronted with a bubble problem especially when operating at high current density conditions. In this work, a gas-emission anode is presented to eliminate the gas accumulation at the anode. This gas-emission anode is verified as a valid design for discharging gaseous products, which is especially beneficial for stable operation of microfluidic fuel cells. The electrochemical performance of a counter-flow microfluidic fuel cell equipped with a gas-emission anode was measured. The results indicate that the specific design of the gas-emission anode is essential for reducing the oxygen reduction reaction parasitic effect at the anode. Fuel utilization of 76.4% was achieved at a flow rate of 0.35 µl min−1. Current-voltage curves of single electrodes were measured and the parasitic effect at the anode was identified as the main performance limiting factor in the presented anode design.
Utilising photoelectrochemical (PEC) devices to produce sustainable fuels from water and CO 2 is a very attractive strategy, in which sunlight is used to convert the greenhouse gas (CO 2 ) into a usable form of stored chemical energy. While significant progress has been made in the development of efficient photoactive catalysts for PEC reactions, limited efforts have been focused on the reactor design where continuous flow microfluidic PEC reactors are particular promising. In this work, a range of CuO-based thin films were used as photocathodes in a continuous flow microfluidic PEC reactor using CO 2 -saturated aqueous NaHCO 3 solution under simulated AM 1.5 solar irradiation for up to 12 h. The highest photocurrent density obtained was for the α-Fe 2 O 3 /CuO photoelectrode yielding −1.0 mA cm −2 at 0.3 V vs. RHE and initial results indicated a solar-to-fuel (STF) efficiency of 0.48%. While the CuO, Cu 2 O and CuO–Cu 2 O photoelectrodes virtually only formed formate, the bilayer α-Fe 2 O 3 /CuO photocathode produced methanol in addition to formate indicating that combined copper and iron oxides in continuous flow microfluidic PEC cells have great potential of direct solar conversion into useful chemicals.
A LHP which had a flat disk-shaped evaporator with a transparent cover was manufactured to observe the flow motion in the evaporating zone and the compensation chamber, and two-layer Cu-Ni composite wicks were also proposed to compare the thermal performance with a copper wick, the total thickness of all wicks was 5 mm. It was observed that there were bubbles accumulated on the upper side of the copper wick during the incipience of the start-up, and heat pipe effect was shown during the operation at the heat load of 120W, due to the high thermal conductivity of the copper material. In addition, the Cu3Ni2 wick, which has 3 mm thickness of copper layer attached at the heat source to enhance the evaporation efficiency and 2 mm thickness of the nickel layer facing to the compensation chamber, is the optimal wick for the LHP. The LHPs with a Cu3Ni2 wick could start fast in about 170 s, and the evaporator wall temperature could be maintained under the allowable temperature of 85 degrees C at the heat load ranged from 30 to 120W. It can be concluded that an optimal wick for the LHPs is that the balance between the efficient evaporation close to heat source and low back conduction problem (heat leak) should be achieved simultaneously. Crown Copyright (c) 2014 Published by Elsevier Ltd. All rights reserved.