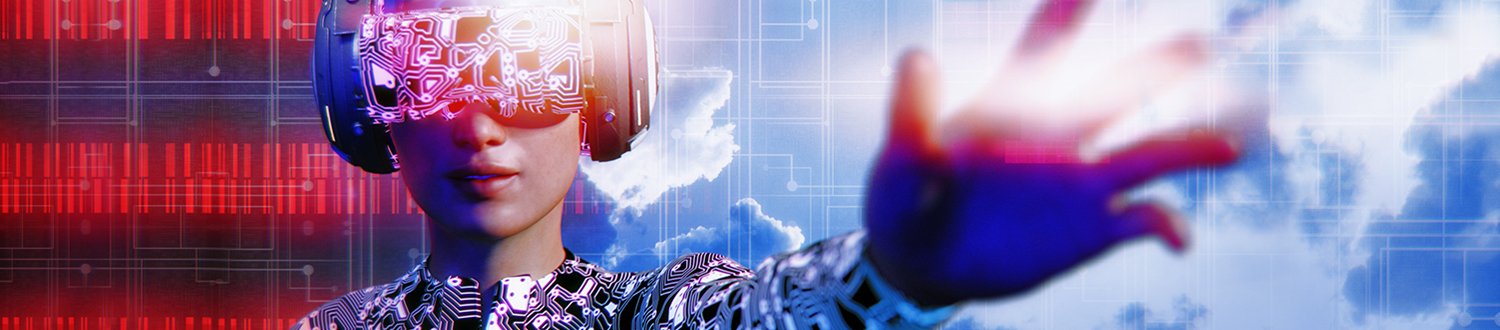
People
We are a collaborative community of academics, researchers, technicians and administrators, supported by additional staff and services within the Faculty of Engineering and Physical Sciences and across the University.
Our staff and students
Find out more about our staff and students and get in contact.
Meet the academic
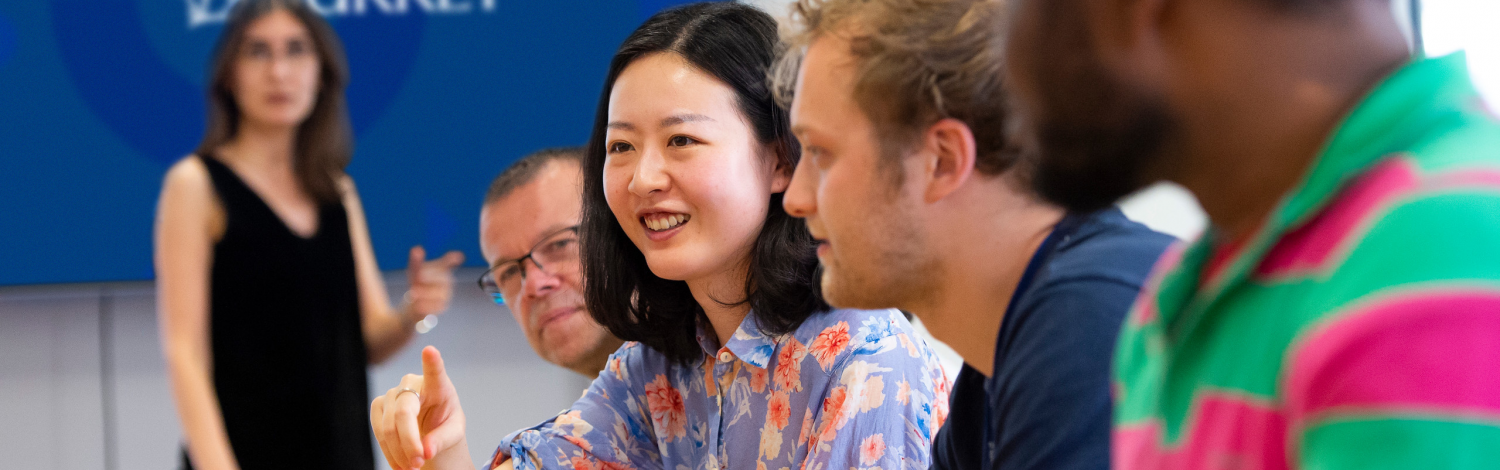
Staff opportunities
If you're interested in working with us then please see our open vacancies.