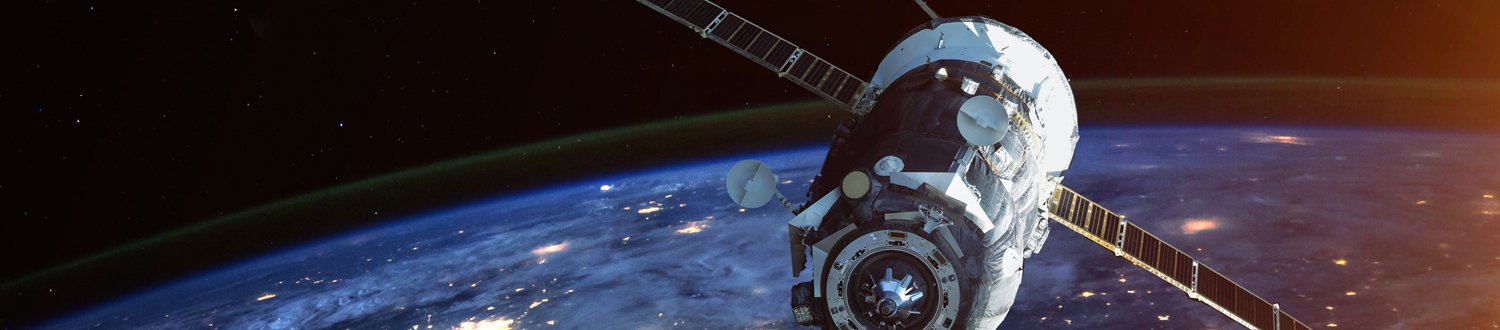
Remote sensing and Earth observation
Remote sensing covers the technologies and techniques enabling the retrieval of information about an object, a phenomenon or a process without being in contact with it.
Our Remote Sensing and Earth Observation group develops models, applications and tools from remotely sensed data with the primary goal of monitoring our planet and its resources.
Our mission
Our interests range from disaster management (flooding monitoring, oil spill detection, post-earthquake damage assessment, conflict observations etc.) to maritime domain awareness (ship detection and classification, illegal fishing detection) to urban areas monitoring, crops classification and soil moisture retrieval.
We are determined to generate impact with our research, whether environmental, economic or societal, and have the ambition to influence policies and regulations with the information extracted from satellite data.
Research lead
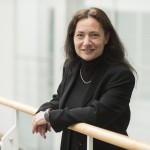
Dr Raffaella Guida
Reader in Satellite Remote Sensing
Synthetic Aperture Radar
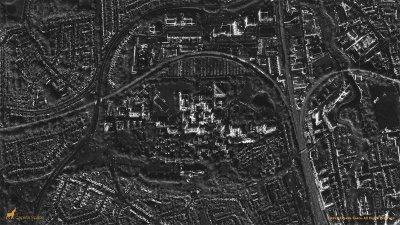
Synthetic Aperture Radar (SAR) is a crucial space technology today thanks to its light - and weather-independent characteristics.
Understanding, interpreting and extracting features from SAR data through ad-hoc processing enable us to provide useful insight to end-users and support decision making processes, especially in situations where other space sensors fail in providing persistent monitoring.
Scattering models for Synthetic Aperture Radar
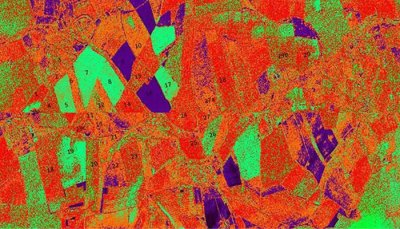
Man-made and natural features differently interact with the electromagnetic radiation. In the case of Synthetic Aperture Radar (SAR) technology and signals, the geometric and dielectric characteristics of the target affect the radar cross section measured by a radar system.
By defining and introducing new scattering models we are able to link the targets parameters and the radar orbital and setting parameters. With proper inversion of the scattering models we manage to extract relevant target features from the SAR dataset.
Data fusion
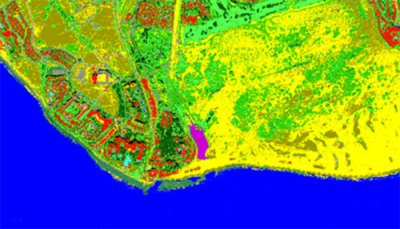
Nowadays a larger and diversified offer of satellite data is available to researchers. However, the fusion of such data is not a trivial task as it requires careful analysis of the temporal gap between datasets as well as of the different information content provided by each dataset.
We develop data fusion algorithms enabling the design of flexible and multi-source Earth observation (EO) solutions with significantly reduced error on the parameter estimated or the class identified.
Data quality assessment for future missions
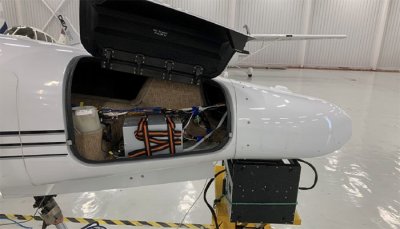
Understanding the benefits of a new Earth observation (EO) mission - and assessing the quality of the data before the mission design is completed - is fundamental to positively influence the design itself and early identify future potential customers.
In collaboration with our industrial partners, we carry out this assessment with ad-hoc designed airborne campaigns where the aircraft carries on board a payload very similar to the one designed for the spaceborne mission. We then process the airborne datasets in such a way to mimic those available in future from the spaceborne mission and complete an assessment on the overall quality.
Collaborations
Our group has established international collaborations with universities, space agencies and governments worldwide including JAXA, NASA, the Mexican Space Agency, DOST Philippines, the Algerian Space Agency, and the Mauritius Research and Innovation Council to name a few.
Our research is funded by Surrey Satellite Technology Ltd, Airbus, the UK National Physical Laboratories, EPSRC, the UK Space Agency, Chevron USA and the Nigerian Petroleum Technology Development Fund.
We welcome visiting students, researchers and professors from all our partner institutions.
Research projects
Meet the team
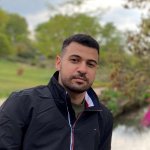
Al Al Hinai
Postgraduate Research Student
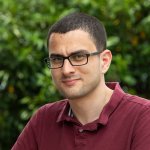
Dr Abdelhakim Benoudjit
Flood monitoring using Synthetic Aperture Radar (SAR) images
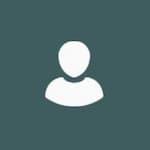
Chedi Chedi
Oil spill characterization for detection and monitoring of oil pollution in marine environment using SAR data
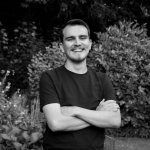
Dr Maximilian Kerslake
Research Fellow