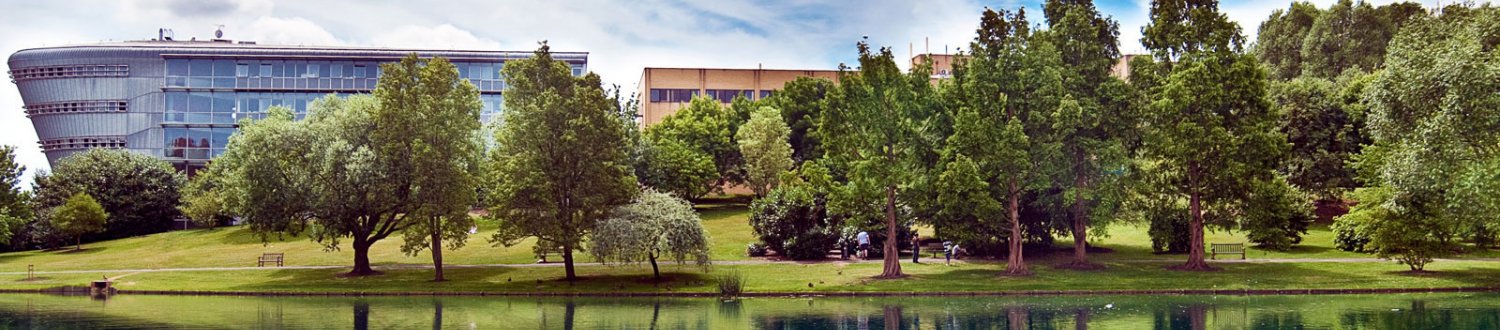
Research
We are pursuing a wide range of research topics within the scope of mathematical and computational biology. Some typical methods that we use are given below.
We are pursuing a wide range of research topics within the scope of mathematical and computational biology. Some typical methods that we use are given below.