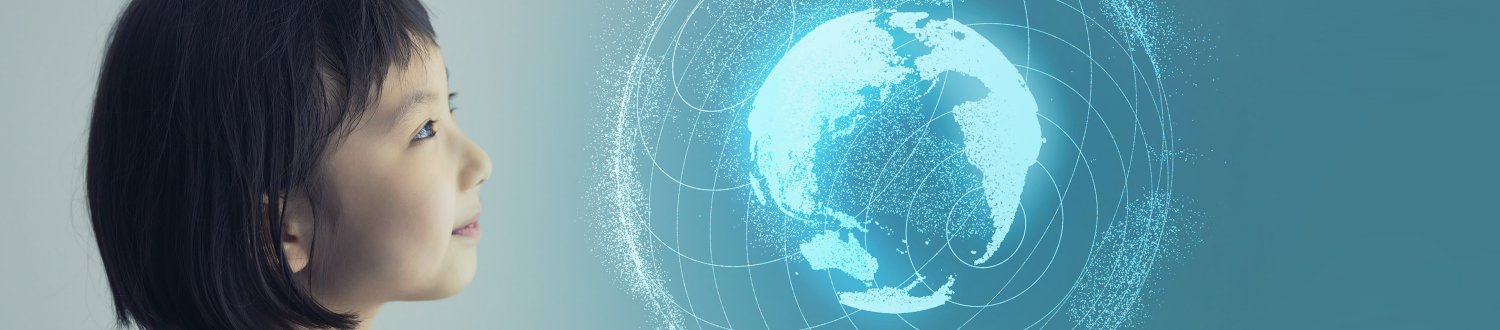
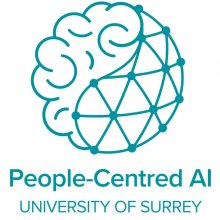
AI for education, information and entertainment
How can AI improve learning and access to trusted information?
AI is disrupting almost all aspects of our lives requiring new workplace skills, new approaches to life-long learning and the retraining of people for the AI enabled workplace. Training for AI leadership in business and the public sector is essential to realise responsible AI, corporate governance and shaping of the future workplace.
AI will transform the way we learn, communicate and access information enabling new forms of personalised education and media content, opening the possibility for personalised messaging in public services such as health. AI Education must include both responsible AI and transformational AI paradigms such as quantum computing.
AI for creative and entertainment
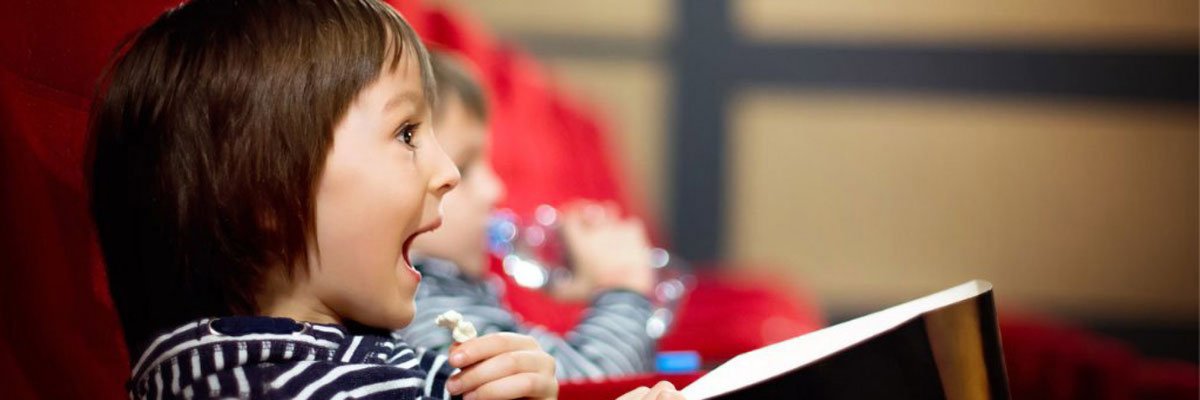
The last 20 years have seen a move from manual to automated digital processes for the creative industries, kick-starting a boom in virtual reality (VR), augmented reality (AR) and 3D spatial audio, and Surrey’s Centre for Vision, Speech and Signal Processing (CVSSP) is at the forefront of research in all three fields.
Visual effects
The Centre has pioneered visual effects which are used to bring stories to life not only in sci-fi blockbusters but also for period dramas, live action sports broadcasts and many other types of entertainment content. It first developed the concept of 3D video capture in the mid-1990s, which opened the door to highly realistic animations based on the real movements of people and animals using AI and machine learning, and it has continued to push the boundaries of these techniques.
4D vision
More recently, CVSSP has developed the concept of ‘4D vision’ which uses a combination of multi-camera capture systems and advanced algorithms to model complex scenes in real time. This is enabling autonomous systems not only for entertainment but also for healthcare, assisted living, animal welfare and security applications.
A step-change in home audio
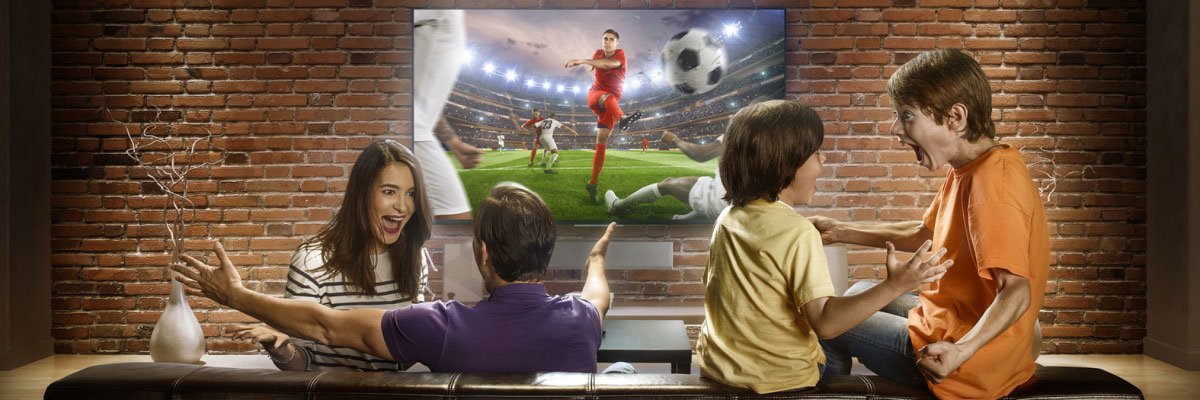
S3A spatial audio will for the first time, give consumers the sense of ‘being there’ at a live event such as a concert or football match from the comfort of their living room without the need for specialist equipment or a complex speaker set-up.
In the field of audio, CVSSP is investigating ‘machine listening’ algorithms that manipulate signals for speech and audio applications, with the ultimate aim of optimising the way audio content is delivered. This includes creating auditory perception computer models which can measure, control and optimise audio to automatically adapt to the listener; enhancing the audio description of images and TV programmes for visually impaired people, and separating audio sources (such as ‘cocktail party’ type speech).
As part of the EPSRC S3A Spatial Audio programme, a major collaboration with the BBC and the Universities of Salford and Southampton, CVSSP is working on enabling a fully immersive at-home listener experience based on spatial audio techniques.
AI for language and learning
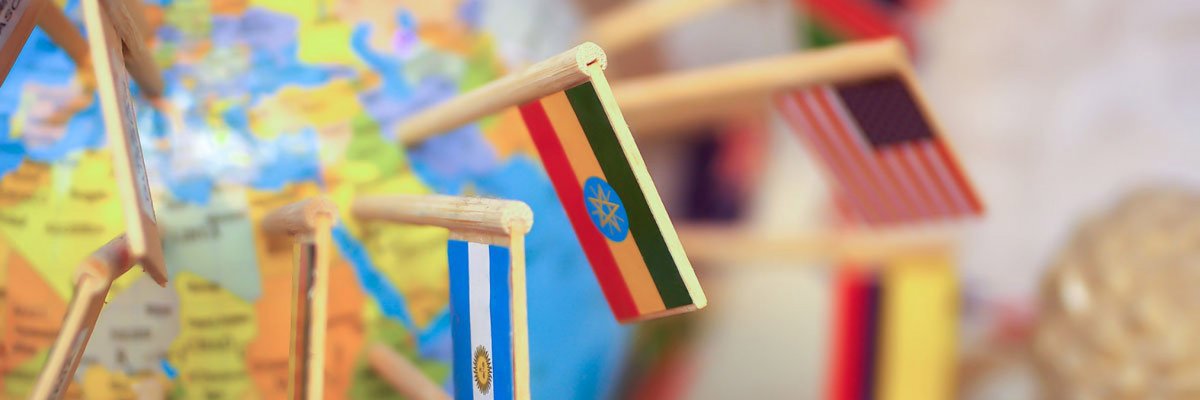
One core objective being addressed at Surrey is building a bridge between an AI system (for example computer vision) and natural language. The Centre for Vision, Speech and Signal Processing (CVSSP) is developing deep neural networks that enable cross-modal (language to vision) searches of video crime scene footage where the description of the scene may be provided by a witness in the form of natural language. Interestingly, this research is opening the door to multimodal processing, where different modalities (vision, language, audio) are jointly brought to bear on data analysis tasks.
Translation automation
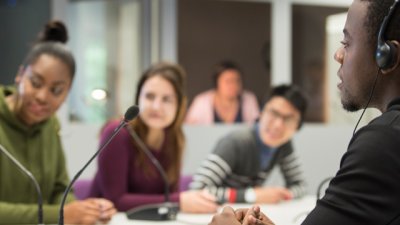
The evolution of AI is having a transformational impact on the translation industry, but while automation has the potential to make translation easier and more cost-effective, it also raises a number of issues. Surrey’s Centre for Translation Studies (CTS) is undertaking wide-ranging research in this field, focusing on translation of written texts, spoken-language interpreting, localisation (of websites, apps and chatbots), and subtitling and audio descriptions (translation of images into verbal descriptions). In June 2019, the Centre began a pioneering programme which aims to identify the social, ethical and economic consequences of AI automation in translation, and promote solutions which improve the inclusion of diverse and marginalised groups in society
Distance interpreting for video conferences
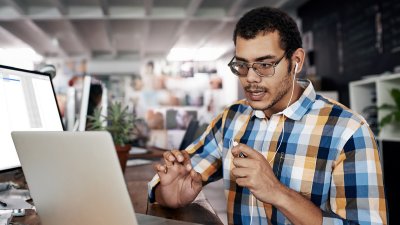
Other research focuses on distance interpreting for video conferences, where the interpreter delivers their services from a remote location. In this scenario, automatic speech recognition and augmented reality can be used to reduce the interpreter’s cognitive load and avoid a reduction in interpreting quality.
Sign language
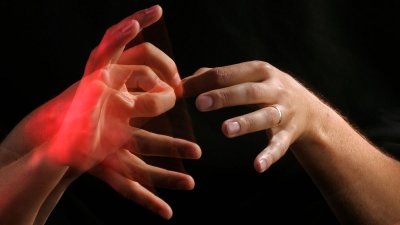
A key theme running through Surrey’s research is the way AI can be exploited to help create a fairer, more inclusive society – and this is especially relevant in the field of language. Translation of sign language into written language has long been an area of research for CVSSP and in 2018 the Centre succeeded in developing the world’s first end-to-end translation system – a complex task requiring an understanding of the interplay of face, body, hands and grammar. This tool has the potential to make a difference to the lives of the 250,000 people who use British Sign Language, enabling the deaf community to participate more fully in the digital revolution.
AI and astrophysics
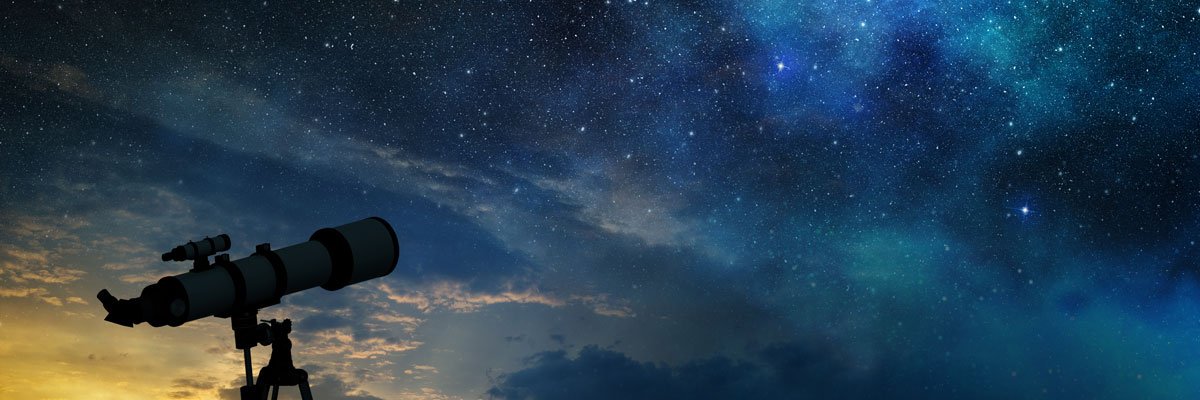
In the field of astrophysics, the advent of ground-based and space-based missions has delivered six-dimensional space-velocity coordinates and up to 20 elemental abundances for more than a million stars in our Milky Way. Deciphering the history of the Milky Way, and the role played by dark matter, requires highly complex computational models. A key objective within Surrey’s School of Mathematics and Physics – in collaboration with CVSSP and the Alan Turing Institute – is therefore to develop new machine learning tools for deriving stellar orbits and ages.
Predicting possible combinations
Within nuclear physics, a major challenge is to understand and predict the properties of all possible combinations of neutrons and protons, or ‘nuclear isotopes’. Isotopes with masses above 100 are typically difficult, if not impossible, to compute using the current generation of computers and theoretical techniques. Surrey’s Theoretical Nuclear Physics group is investigating the use of AI techniques to solve this challenge in two ways.
Firstly, where high performance computing tools are at the limits of their capabilities, researchers are using neural networks to provide a basis for systematic extrapolations of nuclear properties. Secondly – since modern computers cannot store the wavefunctions of nuclear systems with more than 20 particles – they are employing machine learning techniques to directly encapsulate the information of many-body systems, optimising wavefunctions in order to find the best variational solutions for the smallest nuclear systems.
The long-term objective is to extend these techniques to simulate not only the properties of static nuclei but also their dynamics, in order to provide systematic, improvable calculations which could be relevant for processes such as nuclear fusion.
Optimising nanophotonic devices
The development of novel nanophotonic materials is critical for enabling the next generation of technologies which will be used in information processing and communication, energy harvesting, healthcare and biophotonics, and quantum information processing on nanophotonics platforms.
Recent developments in AI have dramatically changed the way that nanophotonic devices are designed, with deep learning algorithms able to provide an almost instantaneous design solution after the learning phase. At Surrey we are investigating how deep learning techniques can be applied to optimise nanophotonic device performance by exploiting the correlation between material properties, structural geometry, topology, and advanced functionalities such as the strength of light-matter interaction in a quantum computing device. We are also focused on identifying and optimising the optical performance of novel types of cavities, low-loss waveguides and optical non-linear devices which can serve as photonic axons, dendrites and somas in the all-optical implementation of artificial neural networks.