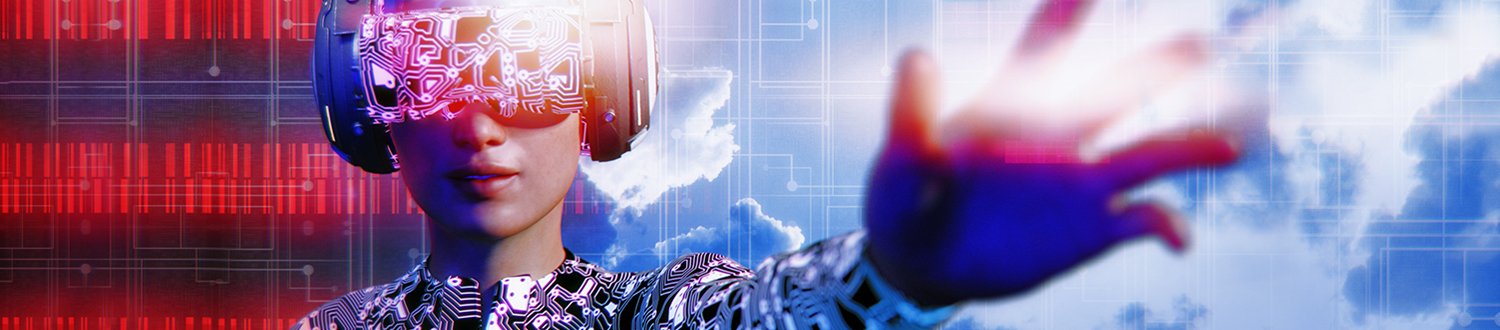
Research
Our vision
'Creating machines that can see, hear and understand the world around them.'
Launched over 30 years ago, the Centre for Vision, Speech and Signal Processing (CVSSP) at the University of Surrey, owes its leading position to the strong foundations from which it has grown and the many talented individuals who have contributed to the Centre’s research.
Over the past three decades, in collaboration with industry, CVSSP has led both fundamental scientific advances and practical application of AI and machine perception for the benefit of society and the economy. A central goal of our research is creating machines that can see and hear to understand and interact with the world around them. CVSSP expertise spans audio, vision, machine learning, AI, medical imaging, signal processing and robotics. The Centre has an outstanding track record of pioneering research leading to successful technology transfer with UK industry and spin-out companies.
Today, CVSSP is a thriving multi-cultural community of more than 150 researchers conducting world-leading research in audio-visual machine perception. We develop ground-breaking technologies to improve people’s lives: from facial recognition for security and medical image understanding for cancer detection, through to 3D audio and video for film production and robots that can work safely alongside people.
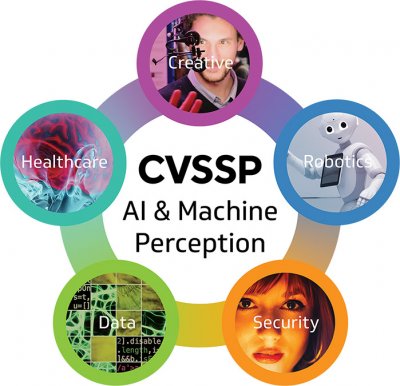
CVSSP advances research in artificial intelligence (AI) and machine perception focusing on cross-disciplinary areas including: Creative technology, healthcare, security and data.
CVSSP research areas
Our research has pioneered new technologies for the benefit of society and the economy, with applications spanning healthcare, security, entertainment, robotics, autonomous vehicles, communication and audio-visual data analysis.