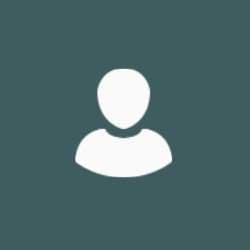
Dr Lee Gillam FBCS CITP FHEA
Academic and research departments
Computer Science Research Centre, School of Computer Science and Electronic Engineering.About
Biography
Reader in the Department of Computer Science, and previously Director of Learning & Teaching. Chartered IT Professional Fellow (FBCS CITP) and member of the EPSRC Peer Review College. Research interests include Cloud Computing and Edge Computing, Connected and Autonomous Vehicles, and Information Retrieval and Information Extraction. Founding Editor-in-Chief of the Springer Journal of Cloud Computing Advances, Systems and Applications (JoCCASA), an editor of two Springer books on Cloud Computing, and member of the Cloud Pro expert panel. Currently a CI on the EPSRC/JLR CARMA project for Cloud Connected and Edge Connected and Autonomous Vehicles. Previously, PI on the successfully completed TSB project (IPCRESS), collaborative with Jaguar Land Rover, to create a private search capability and PI on an EPSRC Project about Fair Benchmarking for Cloud Computing Systems, a co-author of two reports for EPSRC/JISC on Cloud Computing (Research Use Cases, and Costs) and a keen user and proponent of various Cloud infrastructures with several small grants received in support of this work. A key line of investigation in this work relates to service level agreement (SLA) driven Cloud brokerage. Participant in international research competitions (securing 4th place in the External Plagiarism Detection Task of the 2011 Uncovering Plagiarism, Authorship, and Social Software Misuse (PAN) competition, and addressing various tasks of other PAN competitions including for author identification, author profiling, and predator detection). Two patent filings (U.S. Patent filing US13/307,428, filed 30th November 2011; PCT/GB2012/000883, filed 30th November 2012). Has been responsible for software architectures for a number of systems developed for research projects supported by the EU's IT Research and Development programmes - TRANSTERM, POINTER, INTERVAL, ACE, SALT , GIDA, and PI on the eContent project LIRICS - and the UK EPSRC and ESRC - SAFE-DIS, SOCIS and FINGRID.
For further information, and full list of publications, see: Personal Pages
Teaching
Recent
COMM034 - Cloud Computing
COM3001 - Final Year Project
COMM002 - MSc Dissertation
Publications
Recent advances in smart connected vehicles and Intelligent Transportation Systems (ITS) are based upon the capture and processing of large amounts of sensor data. Modern vehicles contain many internal sensors to monitor a wide range of mechanical and electrical systems and the move to semi-autonomous vehicles adds outward looking sensors such as cameras, lidar, and radar. ITS is starting to connect existing sensors such as road cameras, traffic density sensors, traffic speed sensors, emergency vehicle, and public transport transponders. This disparate range of data is then processed to produce a fused situation awareness of the road network and used to provide real-time management, with much of the decision making automated. Road networks have quiet periods followed by peak traffic periods and cloud computing can provide a good solution for dealing with peaks by providing offloading of processing and scaling-up as required, but in some situations latency to traditional cloud data centres is too high or bandwidth is too constrained. Cloud computing at the edge of the network, close to the vehicle and ITS sensor, can provide a solution for latency and bandwidth constraints but the high mobility of vehicles and heterogeneity of infrastructure still needs to be addressed. This paper surveys the literature for cloud computing use with ITS and connected vehicles and provides taxonomies for that plus their use cases. We finish by identifying where further research is needed in order to enable vehicles and ITS to use edge cloud computing in a fully managed and automated way. We surveyed 496 papers covering a seven-year timespan with the first paper appearing in 2013 and ending at the conclusion of 2019.
In many production clouds, with the notable exception of Google, aggregation-based VM placement policies are used to provision datacenter resources energy and performance efficiently. However, if VMs with similar workloads are placed onto the same machines, they might suffer from contention, particularly, if they are competing for similar resources. High levels of resource contention may degrade VMs performance, and, therefore, could potentially increase users' costs and infrastructure's energy consumption. Furthermore, segregation-based methods result in stranded resources and, therefore, less economics. The recent industrial interest in segregating workloads opens new directions for research. In this article, we demonstrate how aggregation and segregation-based VM placement policies lead to variabilities in energy efficiency, workload performance, and users' costs. We, then, propose various approaches to aggregation-based placement and migration. We investigate through a number of experiments, using Microsoft Azure and Google's workload traces for more than twelve thousand hosts and a million VMs, the impact of placement decisions on energy, performance, and costs. Our extensive simulations and empirical evaluation demonstrate that, for certain workloads, aggregation-based allocation and consolidation is similar to 9.61% more energy and similar to 20.0% more performance efficient than segregation-based policies. Moreover, various aggregation metrics, such as runtimes and workload types, offer variations in energy consumption and performance, therefore, users' costs.
The major reason for using a simulator, instead of a real test-bed, is to enable repeatable evaluation of large-scale cloud systems. CloudSim, the most widely used simulator, enables users to implement resource provisioning, and management policies. However, CloudSim does not provide support for: (i) interactive online services; (ii) platform heterogeneities; (iii) virtual machine migration modelling; and (iv) other essential models to abstract a real datacenter. This paper describes modifications needed in the classical CloudSim to support realistic experimentations that closely match experimental outcomes in a real system. We extend, and partially re-factor CloudSim to “PerficientCloudSim” in order to provide support for large-scale computation over heterogeneous resources. In the classical CloudSim, we add several classes for workload performance variations due to: (a) CPU heterogeneities; (b) resource contention; and (c) service migration. Through plausible assumptions, our empirical evaluation, using real workload traces from Google and Microsoft Azure clusters, demonstrates that “PerficientCloudSim” can reasonably simulate large-scale heterogeneous datacenters in respect of resource allocation and migration policies, resource contention, and platform heterogeneities. We discuss statistical methods to measure the accuracy of the simulated outcomes.
Internet of Things (IoT) is producing an extraordinary volume of data daily, and it is possible that the data may become useless while on its way to the cloud, due to long distances. Fog/edge computing is a new model for analysing and acting on time-sensitive data, adjacent to where it is produced. Further, cloud services provided by large companies such as Google, can also be localised to improve response time and service agility. This is accomplished through deploying small-scale datacentres in various locations, where needed in proximity of users; and connected to a centralised cloud that establish a multi-access edge computing (MEC). The MEC setup involves three parties, i.e. service-providers (IaaS), application-providers (SaaS), network-providers (NaaS); which might have different goals, therefore, making resource management difficult. Unlike existing literature, we consider resource management with-respect-to all parties; and suggest game-theoretic resource management techniques to minimise infrastructure energy consumption and costs while ensuring applications' performance. Our empirical evaluation, using Google's workload traces, suggests that our approach could reduce up to 11.95% energy consumption, and ~17.86% user costs with negligible loss in performance. Moreover, IaaS can reduce up-to 20.27% energy bills and NaaS can increase their costs-savings up-to 18.52% as compared to other methods.
Objectives: Global, Covid-driven restrictions around face-to-face interviews for healthcare student selection have forced admissions staff to rapidly adopt adapted online systems before supporting evidence is available. We have developed, what we believe is, the first automated interview grounded in Multiple Mini-Interview (MMI) methodology. This study aimed to explore test re-test reliability, acceptability, and usability of the system. Design, setting and participants: Multi-method feasibility study in Physician Associate (PA) programmes from two UK and one US university during 2019 - 2020. Primary, secondary outcomes: Feasibility measures (test-retest reliability acceptability and usability) were assessed using intra-class correlation (ICC), descriptive statistics, thematic and content analysis. Methods: Volunteers took (T1), then repeated (T2), the automated MMI, with a seven-day interval (+/- 2) then completed an evaluation questionnaire. Admissions staff participated in focus group discussions. Results: Sixty-two students and seven admission staff participated; 34 students and four staff from UK and 28 students and three staff from US universities. Good-excellent test-retest reliability was observed with T1 and T2 ICC between 0.62-0.81 (p
The online world, referred to by some as cyberspace, offers a wide variety of activities: reading written content, interacting with others, engaging with multimedia and playing games of various kinds, and obtaining goods and services. However, many will ignore the written agreements and laws that govern these activities, and because of this, cyberspace can turn into a dangerous place. This chapter explores a small sample of legal and ethical issues that can arise in the interface between cyberspaces and real places for those not paying attention to such matters. The authors note how laws in the physical world remain applicable in the “virtual” world, requiring knowledge of jurisdiction, and discuss the potential for creating systems that protect the user from harm by embedding adherence to laws and providing support for ethics. The authors further explain how such embedding can work to address the complex legalities and potential need for intervention in addictive situations in online gambling.
Infrastructure as a Service (IaaS) Clouds offer capabilities for the high-availability of a wide range of systems, from individual virtual machines to large-scale high performance computing (HPC) systems. But it is argued that the widespread uptake for such systems will only happen if Cloud providers, or brokers, are able to offer bilateral service level agreements (SLAs). In this paper, we discuss how to measure and use quality of service (QoS) information to be able to predict availability, quantify risk, and consider liability in case of failure. We demonstrate through this work that there is a pressing need for such an understanding and explore a set of benchmarks that offers an interesting characterisation of resource performance variability which can be quite significant. We subsequently identify how such information might be used both directly by a user and indirectly via a Cloud Broker in the automatic construction and management of SLAs which reference certain kinds of financial portfolios.
Purpose This paper discusses the National DNA Database (NDNAD) and some of the controversies surrounding it with reference to legal and ethical issues, focusing particularly on privacy and human rights. Governance of this database involves specific exemptions from the Data Protection Act (DPA), and this gives a rise to concerns regarding both the extent of surveillance on the UK population, and the possibility for harm to all citizens. This is of wider importance since every current citizen, and everybody who visits the UK, could become a record in the DNA database. Principally, we explore whether these exemptions would also imply exemptions for software developers from codes of practice and ethics of their professional societies as relate to constructing or maintaining such data and the database. Design/methodology/approach We make a comparison between the principles of the DPA, as would need to be followed by all other organizations handling personal data, professional responsibilities based codes of ethics of professional societies, and the current reality as reported in relation to the NDNAD and the exemptions offered through the DPA. Findings Primarily, if NDNAD were not exempted from certain provisions in the DPA, the potential for the kinds of data leakages and other mishandlings could largely be avoided without the need for further considerations over so-called “data minimization”. We see how the lack of afforded protection allows for a wide range of issues as relate at least to privacy. Originality/value This paper provides the first evaluation of the combination of law, codes of ethics and activities in the real world as related to NDNAD, with concomitant considerations for privacy, liberty and human rights. Originality is demonstrated through consideration of the implications of certain exemptions in the DPA in relation to crime and taxation and national security, and in relating the expected protections for personal data to widely reported evidence that such protections may be variously lacking. In addition, we provide a broad overview of controversies over certain newer kinds of DNA analysis, and other relatively recent findings, that seem generally absent from the vast majority of debates over this kind of analysis.
The increasing number of public clouds, the large and varied range of VMs they offer, and the provider specific terminology used for describing performance characteristics, makes price/performance comparisons difficult. Large performance variation of identically priced instances can lead to clouds being described as ‘unreliable’ and ‘unpredictable’. In this paper, we suggest that instances might be considered mispriced with respect to their deliverable performance – even when provider supplied performance ratings are taken into account. We demonstrate how CPU model determines instance performance, show associations between instance classes and sets of CPU models, and determine class-to-model performance characteristics. We show that pricing based on CPU models may significantly reduce, but not eliminate, price/performance variation. We further show that CPU model distribution differs across different AZs and so it may be possible to obtain better price/performance in some AZs by determining proportions of models found per AZ. However, the resources obtained in an AZ are account dependent, displays random variation and is subject to abrupt change.
The word “Paedophilia” has come a long way from its Greek origin of child-companionship to a Mental Disorder, Social Taboo and Criminal Offence. Various laws are in place to help control such behaviour, protect the vulnerable and restrain related criminal offences. However, enforcement of such laws has become a significant challenge with the advent of social media creating a new platform for this old crime. This move necessitates consideration of approaches that are suited to this new platform and the way in which it affects the Cycle of Entrapment. This paper reviews definitions of, and features of, paedophilia and other related –philias, and sexual offences against children, and seeks through the understanding of these to determine where specific detection approaches are effective. To this end, we present our own detection approach which is geared towards predatory behaviours, which can be a precursor to sexual offences against children, and which directly references this Cycle of Entrapment. Our approach has shown early promise with an F1 score of 0.66 for training data but only achieving 0.48 for testing data on a collection of chat logs of sexual predators. The results were later improved to achieve an F1 score of 0.77 for train and 0.54 for test data based on the approach.
This paper discusses a method for corpus-driven ontology design: extracting conceptual hierarchies from arbitrary domain-specific collections of texts. These hierarchies can form the basis for a concept-oriented (onomasiological) terminology collection, and hence may be used as the basis for developing knowledge-based systems using ontology editors. This reference to ontology is explored in the context of collections of terms. The method presented is a hybrid of statistical and linguistic techniques, employing statistical techniques initially to elicit a conceptual hierarchy, which is then augmented through linguistic analysis. The result of such an extraction may be useful in information retrieval, knowledge management, or in the discipline of terminology science itself.
This paper provides an overview of the dual challenges involved in protecting intellectual property while distributing business information that will need to be readable/viewable at some point such that it can be acted upon by parties external to the organization. We describe the principles involved with developing such a system as a means to engender trust in such situations – as a deperimeterized supply chain likely acting through the Cloud – discuss the requirements for such a system, and demonstrate that such a system is feasible for written text by formulating the problem as one related to plagiarism detection. The core of the approach, developed previously, has been shown to be effective in finding similar content (precision: 0.88), and has some robustness to obfuscation, without needing to reveal the content being sought.
In this paper we explore the distribution of training of self-organised maps (SOM) on grid middleware. We propose a two-level architecture and discuss an experimental methodology comprising ensembles of SOMs distributed over a grid with periodic averaging of weights. The purpose of the experiments is to begin to systematically assess the potential for reducing the overall time taken for training by a distributed training regime against the impact on precision. Several issues are considered: (i) the optimum number of ensembles; (ii) the impact of different types of training data; and (iii) the appropriate period of averaging. The proposed architecture has been evaluated in a grid environment, with clock-time performance recorded.
Datacenters provide an IT backbone for today's business and economy, and are the principal electricity consumers for Cloud computing. Various studies suggest that approximately 30% of the running servers in US datacenters are idle and the others are under-utilized, making it possible to save energy and money by using Virtual Machine (VM) consolidation to reduce the number of hosts in use. However, consolidation involves migrations that can be expensive in terms of energy consumption, and sometimes it will be more energy efficient not to consolidate. This paper investigates how migration decisions can be made such that the energy costs involved with the migration are recovered, as only when costs of migration have been recovered will energy start to be saved. We demonstrate through a number of experiments, using the Google workload traces for 12,583 hosts and 1,083,309 tasks, how different VM allocation heuristics, combined with different approaches to migration, will impact on energy effciency. We suggest, using reasonable assumptions for datacenter setup, that a combination of energy-aware ll-up VM allocation and energy-aware migration, and migration only for relatively long running VMs, provides for optimal energy efficiency.
Infrastructure Clouds offer large scale resources for rent, which are typically shared with other users—unless you are willing to pay a premium for single tenancy (if available). There is no guarantee that your instances will run on separate hosts, and this can cause a range of issues when your instances are co-locating on the same host including: mutual performance degradation, exposure to underlying host failures, and increased threat surface area for host compromise. Determining when your instances are co-located is useful then, as a user can implement policies for host separation. Co-location methods to date have typically focused on identifying co-location with another user’s instance, as this is a prerequisite for targeted attacks on the Cloud. However, as providers update their environments these methods either no longer work, or have yet to be proven on the Public Cloud. Further, they are not suitable to the task of simply and quickly detecting co-location amongst a large number of instances. We propose a method suitable for Xen based Clouds which addresses this problem and demonstrate it on EC2—the largest Public Cloud Infrastructure.
Cloud computing continues to play a major role in transforming the IT industry by facilitating elastic on-demand provisioning of computational resources including processors, storage and networks. This is necessarily accompanied by the creation, and refreshes, of large-scale systems including cluster, grids and datacenters from which such resources are provided. These systems consume substantial amounts of energy, with associated costs, leading to signi cant CO2 emissions. In 2014, these systems consumed 70 billion kWh of energy in US; this is 1.8% of the US total energy consumption, and future consumption is expected to continue around this level with approximately 73 billion kWh by 2020. The energy bills for major cloud service providers are typically the second largest item in their budgets due to the increased number of computational resources. Energy effciency in these systems serves the providers interests in saving money to enable reinvestment, reduce supply costs and also reduces CO2 emissions. In this paper, we discuss energy consumption in large scale computing systems, such as scientfii c high performance computing systems, clusters, grids and clouds, and whether it is possible to decrease energy consumption without detrimental impact on service quality and performance. We discuss a number of approaches, reported in the literature, that claim to improve the energy efficiency of such large scale computing systems, and identify a number of open challenges. Key fi ndings include: (i) in clusters and grids, use of system level efficiency techniques might increase their energy consumption; (ii) in (virtualized) clouds, efficient scheduling and resource allocation can lead to substantially greater economies than consolidation through migration; and (iii) in clusters, switching off idle resources is more energy efficient, however in (production) clouds, performance is affected due to demand fluctuation.
How do human beings tell the difference between truths and lies, and avoid being deceived? And is it possible for a machine to determine the veracity of any given statement or set of statements prior to incorporating such statements in a knowledge base, or to determine whether the deception even exists at the statement level? This paper reviews past research in deception and its detection to explore such questions. We focus in on various inconsistencies, contradictions, and other difficulties in recent deception research, and show how the nature of the deception largely dictates the methods that can be deployed effectively in detection by reference to several experiments on materials which can have a strongly deceptive framing.
Simulations are often used to evaluate the performance of various scheduling and migration techniques in the context of large computing systems such as clouds and datacenters. To ensure that simulations match the real platform as close as possible, plausible assumptions and accurate statistical models are used in designing simulations; and that could also offer accurate results. However, it is not always possible that similar numerical results would also be achievable in a real cloud test-bed. The reason is that a simulator only abstracts a model and, hence, a system; but does not always reflect the real world scenarios. Therefore, the solution of any research problem using numerical simulation (experimentation) is not just to find a result, but also to ensure the quality and accuracy of the estimated results. CloudSim is largely used in the cloud research community to evaluate the performance of various resource allocation and migration policies. However, resources such as CPU, memory and application heterogeneities are not modelled yet. Moreover, its accuracy is rarely addressed. In this paper, we: (i) describe an extension to CloudSim that offers support for resource (CPU) and application heterogeneities; and (ii) demonstrate several techniques that could be used to measure the accuracy of results obtained in simulations, particularly, in the extended CloudSim. Based on our evaluation, we suggest that the accuracy and precision of the extended version of the CloudSim simulator may be as high as ∼ 98.63% for certain energy and performance efficient resource allocation and consolidation with migration policies in heterogeneous datacenters.
The performance of Cloud systems is a key concern, but has typically been assessed by the comparison of relatively few Cloud systems, and often on the basis of just one or two features of performance. In this paper, we discuss the evaluation of four different Infrastructure as a Service (IaaS) Cloud systems – from Amazon, Rackspace, and IBM – alongside a private Cloud installation of OpenStack, using a set of five so-called micro-benchmarks to address key aspects of such systems. The results from our evaluation are offered on a web portal with dynamic data visualization. We find that there is not only variability in performance by provider, but also variability, which can be substantial, in the performance of virtual resources that are apparently of the same specification. On this basis, we can suggest that performance-based pricing schemes would seem to be more appropriate than fixed-price schemes, and this would offer much greater potential for the Cloud Economy.
The trading of virtual machines, storage and other Cloud Computing resources on commodity exchanges has generated considerable interest from both academia and the financial services market. With multiple sellers providing appropriately equivalent virtual machines they become fungible, offering the opportunity for users to swap instances from one seller with instances from another when required, easing concerns over vendor lock-in, availability and provider failure. However, heterogeneity in the hardware from which they are provisioned is likely inevitable, given heterogeneity already found on a number of Public Clouds, where it results in performance variation across instances of the same type, and consequently variation in Cloud costs at the same price. To address this problem we propose a Cloud Service Broker (CSB) that acquires and re-sells instances on the basis of their current performance. The service reduces performance risk for users, but comes at a cost. We determine the average markup the CSB must add to the base instance price to cover the costs of operating a pool capable of satisfying a given proportion of requests. We show how increases in heterogeneity on the underlying commodity exchange lead to lower costs for the CSB, and that the largest degree of heterogeneity we consider, on the basis of real-world findings, leads to the best outcome: an average markup of just 12% on the buy price from the exchange will satisfy 95% of requests. This creates opportunities for CSB profitability to be explored on the basis of selling performance-assured instances at prices that will account for this markup.
Improving datacenter energy efficiency becomes increasingly important due to energy supply problems, fuel costs and global warming. Virtualisation can help to improve datacenter energy efficiency through server consolidation which involves migrations that can be expensive in terms of extra energy consumption and performance loss. This is because, in clouds, Virtual Machines (VMs) of the same instance class running on different hosts may perform quite differently due to resource heterogeneity. As a result of variations in performance, different runtimes will exist for a given workload, with longer runtimes potentially leading to higher energy consumption. For a large datacenter, this would both reduce the overall throughput, and increase overall energy consumption and costs. In this paper, we demonstrate how the performance of workloads across different CPU models leads to variability in energy efficiencies, and therefore costs.We investigate through a number of experiments, using the Google workload traces for 12,583 hosts and 492,309 tasks, the impact of migration decisions on energy efficiency when performance variations of workloads are taken into account. We discuss several findings, including (i) the existence of a trade-off between overall energy consumption and performance (hence cost), (ii) that higher utilization decreases the energy efficiency as it offers fewer chances to CPU management tools for energy savings, and (iii) how our migration approach could save up to 3.66% energy, and could improve VMs performance up to 1.87% compared with no migration. Similarly, compared with migrate all, the proposed migration approach could save up to 2.69% energy, and improve VMs performance up to 1.01%. We discuss these results for different combinations of VM allocation, migration policies and different benchmark workloads1.
ObjectivesGlobal, COVID-driven restrictions around face-to-face interviews for healthcare student selection have forced admission staff to rapidly adopt adapted online systems before supporting evidence is available. We have developed, what we believe is, the first automated interview grounded in multiple mini-interview (MMI) methodology. This study aimed to explore test–retest reliability, acceptability and usability of the system.Design, setting and participantsMultimethod feasibility study in Physician Associate programmes from two UK and one US university during 2019–2020.Primary, secondary outcomesFeasibility measures (test–retest reliability, acceptability and usability) were assessed using intraclass correlation (ICC), descriptive statistics, thematic and content analysis.MethodsVolunteers took (T1), then repeated (T2), the automated MMI, with a 7-day interval (±2) then completed an evaluation questionnaire. Admission staff participated in focus group discussions.ResultsSixty-two students and seven admission staff participated; 34 students and 4 staff from UK and 28 students and 3 staff from US universities. Good-excellent test–retest reliability was observed at two sites (US and UK2) with T1 and T2 ICC between 0.65 and 0.81 (p
This paper proposes a distributed control strategy for homogeneous platoon systems with external disturbances under random packet drop scenario which can occur due to underlying network among the vehicles in a platoon. An linear matrix inequality (LMI) based approach is used to obtain the controller gains for ensuring the stability with bounded H∞ norm for such systems. Effectiveness of the proposed method is demonstrated with numerical results considering different network topologies in a platoon under single packet drop. The variation of H∞ norm bound for different number of platoon members under the different structure of network topologies and the packet drop has been studied in this paper.
In this paper, we explore a distributed computing architecture that address on-vehicle and off-vehicle computation as will be needed to support connected and autonomous/automated driving. We suggest a need for computation to be more local to vehicles in order to reduce end-to-end latency, and identify the key role that mobile/multi-access edge computing (MEC), over 5G telecommunications, may be able to play. We also characterize the present state-of-the-art in edge computing offerings from major Cloud Computing providers, and the extent to which these can meet such needs.
This paper proposes a discrete time distributed state feedback controller design strategy for a homogenous vehicle platoon system with undirected network topology which is resilient to both external disturbances and random consecutive network packet drop. The system incorporates a distributed state feedback controller design by satisfying bounded H-infinity norm using Lyapunov-Krasovskii based linear matrix inequality (LMI) approach that ensures internal stability and performance. The effect of packet drops on internal stability in terms of stability margin are studied for a homogenous vehicle platoon system with undirected network topology and external disturbance. The variation of stability margin, representing absolute value of least stable close-loop pole, is also studied for two common undirected network topologies for vehicle platooning, i.e., bidirectional predecessor following (BPF) and bidirectional predecessor leader following (BPLF) topologies by varying platoon members, packet drop rates with number of contiguous packets dropped. Results demonstrate that the control strategy best satisfies the requirement of maintaining a desired inter-vehicular distance with constant spacing policy and leader trajectory using two network topologies: BPF and BPLF. We show how these topologies are robust in terms of ensuring internal stability and performance to maintain cooperative motion of vehicle platoon system with different number of followers, random multiple consecutive packet drops and external disturbance.
This paper briefly describes the approach taken to the subtask of Text Alignment in the Plagiarism Detection track at PAN 14. We have now reimplemented our PAN12 approach in a consistent programmatic manner, courtesy of secured research funding. PAN 14 offers us the first opportunity to evaluate the performance/consistency of this re-implementation. We present results from this re-implementation with respect to various PAN collections.
The large number of Cloud Infrastructure Providers offering virtual machines in a variety sizes, but without transparent performance descriptions,makes price/performance comparisons difficult.This presents opportunities for Cloud Service Brokers (CSBs). A CSB could offer transparent price/performance comparisons, or performance discovery. This paper explores the kinds of performance measures such CSBs should use. Using three quite different benchmarks, povray, bzip2 and STREAM, we show a 17%, 77% and a 340% increase in performance,respectively, from worst to best in an existing large Cloud. Based on these results, we propose a discovery service for best price/performance for use in aggregation of heterogeneous resources.
Variable compute performance has been widely reported on for virtual machine instances of the same type, and price, on Public Infrastructure Clouds. This has led to the proposal of a number of so called ‘instance seeking’ or ‘placement gaming’ strategies, with the aim of obtaining better performing instances for the same price for a given workload. However, a number of assumptions made in models presented in the literature fail to hold for real large-scale Public Infrastructure Clouds. We demonstrate, using data from our experiments on EC2, the problems of such assumptions, discuss how these models are likely to underestimate the costs involved, and demonstrate why such literature requires a better Cloud Compute Model.
Encouraged by results from our approaches in previous PAN workshops, this paper explores three different approaches using stopword cooccurrence. High frequency patterns of co-occurrence can be used to some extent as identifiers of an author’s style, and have been demonstrated to operate similarly across certain languages - without requiring deeper linguistic knowledge. However, making best use of such information remains unresolved. We compare results from applying three approaches overs such patterns: a frequency-mean-variance framework; a positional-frequency cosine comparison approach, and a cosine distance-based approach. A clearly advantageous approach across all languages and genres is yet to emerge.
This paper briefly describes the approach taken to Persian Plagiarism Detection based on modification to the approach used for PAN between 2011 and 2014 in order to adapt to Persian. This effort has offered us the opportunity to evaluate detection performance for the same approach with another language. A key part of the motivation remains that of undertaking plagiarism detection in such a way as to make it highly unlikely that the content being matched against could be determined based on the matches made, and hence to allow for privacy.
This paper accompanies a keynote speech given at the 8th International Conference on Cloud Computing and Services Science, CLOSER 2018. The keynote offered an overview of ‘traditional’ and ‘new’ Cloud Computing, and what we might appreciate of each. In respect to ‘traditional’, issues of performance and energy efficiency, and the potential conflict between these, were discussed, as well as how these were still relevant to ‘new’ Cloud. Key to the ‘new’ Cloud is the advent of so-called function-as-a-service and edge, to which these issues of performance and lessons learned from energy efficiency can be applied. Important to this is to establish what we mean by edge as distinct from other things as may be similarly referred to. The relevance of new Cloud, then, to Connected and Autonomous Vehicles offers for an industry vertical that could exploit such formulations, and attempts to do this will lead to a variety of technical and research questions. Also, with a person in America having been killed by a vehicle acting autonomously near to the timing of this talk, safety concerns should never be far from thinking in addressing such questions.
In this paper, we discuss the nature of variability in compute performance in Infrastructure Clouds and how this presents opportunities for Cloud Service Brokers (CSB) in relation to pricing. Performance variation in virtual machines of the same type and price raises specific issues for end users: (i) the time taken to complete a task varies with performance, and therefore costs also vary (ii) the number of instances required to meet a certain problem scale within a given time being variable, so costs depend on variations in scale needed to meet the requirement; (iii) different computational requirements are better satisfied by different hardware, and understanding the relationship between instance types and available resources implies further costs. We demonstrate such variability problems empirically in a Public Infrastructure Cloud, and use the data gathered to discuss performance price issues, and how a CSB may re-price instances based on their performance.
Most current Infrastructure Clouds are built on shared tenancy architectures, with resources shared amongst large numbers of customers. However, multi tenancy can lead to performance issues (so-called “noisy neighbours”) and also brings potential for serious security breaches such as hypervisor breakouts. Consequently, there has been a focus in the literature on identifying co-locating instances that are being affected by noisy neighbours or suggesting that such instances are vulnerable to attack. However, there is limited evidence of any such attacks in the wild. More beneficially, knowing that there is co-location amongst your own Virtual Machine instances (siblings) can help to avoid being your own worst enemy: avoiding your instances acting as your own noisy neighbours, building resilience through ensuring hostbased redundancy, and/or reducing exposure to a single compromised host. In this paper, we propose and demonstrate a test to detect co-locating sibling instances on Xen-based Clouds, as could help address such needs, and evaluate its efficacy on Amazon’s EC2.
In this paper we explore the distribution of training of self-organised maps (SOM) on Grid middleware. We propose a two-level architecture and discuss an experimental methodology comprising ensembles of SOMs distributed over a Grid with periodic averaging of weights. The purpose of the experiments is to begin to systematically assess the potential for reducing the overall time taken for training by a distributed training regime against the impact on precision. Several issues are considered: (i) the optimum number of ensembles; (ii) the impact of different types of training data; and (iii) the appropriate period of averaging. The proposed architecture has been evaluated in a Grid environment, with clock-time performance recorded.
Previous PAN workshops have offered us the opportunity to explore three different approaches using basic statistics of stopword pairs for author verification. In this PAN, we were able to select our ‘best’ approach and explore the question of how authors writing about different subjects would necessarily adapt to term lengths specific to the subject. The adaptation required is, essentially, a redistribution of frequency: where longer terms occur. We introduce the notion of a ‘topic cost’ which increases the propensity for matching. Results show AUC and C1 scores of 0.51, 0.46 and 0.59 for Dutch, Greek and Spanish respectively. The English results are not yet available, as the evaluation system was unable to run the approach due to as yet unknown reasons.
Previous PAN workshops have afforded evaluation of our approaches to author verification/identification based on stopword cooccurrence patterns. Problems have tended to involve comparing one document to a small set of documents (n
Tasks such as Authorship Attribution, Intrinsic Plagiarism detection and Sexual Predator Identification are representative of attempts to deceive. In the first two, authors try to convince others that the presented work is theirs, and in the third there is an attempt to convince readers to take actions based on false beliefs or ill-perceived risks. In this paper, we discuss our approaches to these tasks in the Author Identification track at PAN2012, which represents our first proper attempt at any of them. Our initial intention was to determine whether cues of deception, documented in the literature, might be relevant to such tasks. However, it quickly became apparent that such cues would not be readily useful, and we discuss the results achieved using some simple but relatively novel approaches: for the Traditional Authorship Attribution task, we show how a mean-variance framework using just 10 stopwords detects 42.8% and could be obtain 52.12% using fewer; for Intrinsic Plagiarism Detection, frequent words achieved 91.1% overall; and for Sexual Predator Identification, we used just a few features covering requests for personal information, with mixed results.
To some the next iteration of Grid and utility computing, Clouds offer capabilities for the high-availability of a wide range of systems. But it is argued that such systems will only attain acceptance by a larger audience of commercial end-users if binding Service Level Agreements (SLAs) are provided. In this paper, we discuss how to measure and use quality of service (QoS) information to be able to predict availability, quantify risk, and consider liability in case of failure. We explore a set of benchmarks that offer both an interesting characterisation of resource performance variability, and identify how such information might be used both directly by a user and indirectly via a Cloud Broker in the automatic construction of SLAs.
This practically-focused reference presents a comprehensive overview of the state of the art in Cloud Computing, and examines the potential for future Cloud and Cloud-related technologies to address specific industrial and research challenges. This new edition explores both established and emergent principles, techniques, protocols and algorithms involved with the design, development, and management of Cloud-based systems. The text reviews a range of applications and methods for linking Clouds, undertaking data management and scientific data analysis, and addressing requirements both of data analysis and of management of large scale and complex systems. This new edition also extends into the emergent next generation of mobile telecommunications, relating network function virtualization and mobile edge Cloud Computing, as supports Smart Grids and Smart Cities. As with the first edition, emphasis is placed on the four quality-of-service cornerstones of efficiency, scalability, robustness, and security.
The concept of clouds seems to blur the distinctions between a variety of technologies that encompass grid services, web services and data centres, and leads to ...
Defeating plagiarism detection systems involves determining effective approaches for greatest impact at lowest cost with the least likelihood of detection. Relatively simple techniques have been applied elsewhere for avoiding plagiarism detection, demonstrated at the last HEA-ICS conference. In this paper, we discuss defeats for seven plagiarism detection systems, including Essayrater, Seesources, PlagiarismDetector, and the popular Turnitin. We report on initial results of human experiments undertaken on visual similarity to assess the risk of human detection of changes. The systems evaluated are variously susceptible to sufficient numbers of small alterations to characters or words in the text Our results suggest, at minimum, to use at least 2 such systems in combination to reduce the likelihood of failed detection and increase the difficulty for the determined, and yet somehow lazy, plagiarist – otherwise, the discovery and dissemination of simple defeats for plagiarism detection software may mean that we may as well just “Turnitoff”.
Previous PAN workshops have offered us the opportunity to explore three different approaches using basic statistics of stopword pairs for author verification. In this PAN, we were able to select our ‘best’ approach and explore the question of how authors writing about different subjects would necessarily adapt to term lengths specific to the subject. The adaptation required is, essentially, a redistribution of frequency: where longer terms occur. We introduce the notion of a ‘topic cost’ which increases the propensity for matching. Results show AUC and C1 scores of 0.51, 0.46 and 0.59 for Dutch, Greek and Spanish respectively. The English results are not yet available, as the evaluation system was unable to run the approach due to as yet unknown reasons.
Cyberspace offers up numerous possibilities for entertainment and leisure, and can be a rich source for information. Unfortunately, it can also be a dangerous place for the unwary or ill-informed. In this chapter, we discuss some of the legal and ethical issues that can arise in the interface between cyberspaces and real places for virtual tourists. We mention the difficulties posed by variations in laws in the physical world, and how these make for problems in the virtual world. We discuss how it is possible to create systems that embed adherence to laws and provide support for ethics in order to avoid harm to the unwary or ill-informed. We show how we have applied such principles in a machine ethics system for online gambling.
To attract more users to commercially available computing, services have to specify clearly the charges, duties, liabilities and penalties in service level agreements (SLAs). In this paper, we build on our existing work in SLAs by making easy measurements for a specific application run within a commercial cloud. An outcome of this work is that certain application may run better than in a grid or HPC environment, and this backs a recent hypothesis.
In this paper we elaborate a near-duplicate and plagiarism detection service that combines both Crowd and Cloud computing in searching for and evaluating matching documents. We believe that our approach could be used across collaborating or competing Enterprises, or against the web, without any Enterprise needing to reveal the contents of its corporate (confidential) documents. The Cloud service involves a novel document fingerprinting approach which derives grammatical patterns but does not require grammatical knowledge and does not rely on hash-based approaches. Our approach generates a lossy and highly compressed document signature from which it is possible to generate fixed-length patterns as fingerprints or shingles. Fingerprint sizes are established by estimating likely random hit rates resulting from the size of the pattern and target search. Our Cloud service is geared towards enabling detection of Clowns, those who may attempt to, or have, leaked confidential or sensitive information, or have otherwise plagiarized, without needing to provide a copy of the original information. Crowds are to be used to validate results emerging from systematic evaluation of the service, ensuring that service modifications continue to act effectively and enabling continuous scaling-up. We discuss the formulation of the service and assess the efficacy of the fingerprinting approach by reference to an international benchmarking competition where we believe our system achieves top 5 performance (Precision=0.96 Recall=0.39).
In this paper, we present a new approach to writing tools that extends beyond the rudimentary spelling and grammar checking to the content of the writing itself. Linguistic methods have long been used to detect familiar lexical patterns in the text to aid automatic summarization and translation of documents. We apply these methods to determine the quality of the text and implement new techniques for measuring readability and providing feedback to authors on how to improve the quality of their documents. We take an extended view of readability that considers text cohesion, propositional density, and word familiarity. We provide simple feedback to the user detailing the most and least readable sentences, the sentences most densely packed with information and the most cohesive words in their document. Commonly used verbose words and phrases in the text, as identified by The Plain English Campaign, can be replaced with user-selected replacements. Our techniques were implemented as a free download extension to the Open Office word processor generating 6,500 downloads to date.
In this paper, we explore deception in its various guises. We identify the difference between lies and deception, and highlight the consideration of medium and message in both deception and its detection. We envisage a Web Filter for deception which could be used equally well as an assistive service for human readers, and as a mechanism for a system that learns from the web. To this end, we also explore whether the research appears to be sufficient to allow for the construction of such a filter.
Encouraged by results from our approaches in previous PAN workshops, this paper explores three different approaches using stopword cooccurrence. High frequency patterns of co-occurrence can be used to some extent as identifiers of an author’s style, and have been demonstrated to operate similarly across certain languages - without requiring deeper linguistic knowledge. However, making best use of such information remains unresolved. We compare results from applying three approaches overs such patterns: a frequency-mean-variance framework; a positional-frequency cosine comparison approach, and a cosine distance-based approach. A clearly advantageous approach across all languages and genres is yet to emerge.
Various papers have reported on the differential performance of virtual machine instances of the same type, and same supposed performance rating, in Public Infrastructure Clouds. It has been established that instance performance is determined in large part by the underlying hardware, and performance variation is due to the heterogeneous nature of large and growing Clouds. Currently, customers have limited ability to request performance levels, and can only identify the physical CPU backing an instance, and so associate CPU models with expected performance levels, once resources have been obtained. Little progress has been made to predict likely performance for instances on such Public Clouds. In this paper, we demonstrate how such performance predictions could be provided for, predicated on knowledge derived empirically from one common Public Infrastructure Cloud. Copyright © 2014 SCITEPRESS - Science and Technology Publications.
In this paper we report on our high-performance plagiarism detection system which is able to process the PAN plagiarism corpus for the external plagiarism detection task within relatively short timescales in contrast to previously reported state-of-the-art, and still produce a reasonable degree of performance (PAN 11, 4th place, PlagDet=0.2467329, Recall=0.1500480, Precision=0.7106536, Granularity=1.0058894). At the core of our system is a simple method which avoids the use of hash-type approaches, but about which we are unable to disclose too many details due to a patent application in progress. We optimised our performance using the PAN10 collection, and used the best parameters for the final submission. We anticipated a relatively similar performance at PAN11, modulo changes to the plagiarism cases, and 4th place this year put us between participants who had been 5th and 6th in PAN 10.
In this paper, we discuss the potential use of cloud computing for hosting and analysis of email. In particular, we are working towards the development of executable acceptable use policies (execAUPs) that assist organizations in preventing certain kinds of detrimental employee activities. We consider requirements for execAUPs, and outline initial efforts in using Microsoft's Azure as an environment for providing hosted storage for such research.
We propose a method for improving object recognition in street scene images by identifying and filtering out background aspects. We analyse the semantic relationships between foreground and background objects and use the information obtained to remove areas of the image that are misclassified as foreground objects. We show that such background filtering improves the performance of four traditional object recognition methods by over 40%. Our method is independent of the recognition algorithms used for individual objects, and can be extended to generic object recognition in other environments by adapting other object models
Service Level Agreements (SLAs) become increasingly important in Clouds, Grids and Utilities. SLAs which provide bilaterally beneficial terms are likely to attract more consumers and clarify expectations of both consumers and providers. This chapter extends our existing work in SLAs through evaluating application- specific costs within a commercial Cloud, a private Eucalyptus Cloud, and a Grid-based system. We assess the total runtime, as well as the wait time due to scheduling or the booting time of a virtual instance. With relatively short processes, this start-up overhead becomes insignificant. In undertaking these experiments, we have provided some justification for a recent hypothesis relating to a preference for job completion time over raw compute performance [7].
Additional publications
For full list of publications, see: Personal Pages