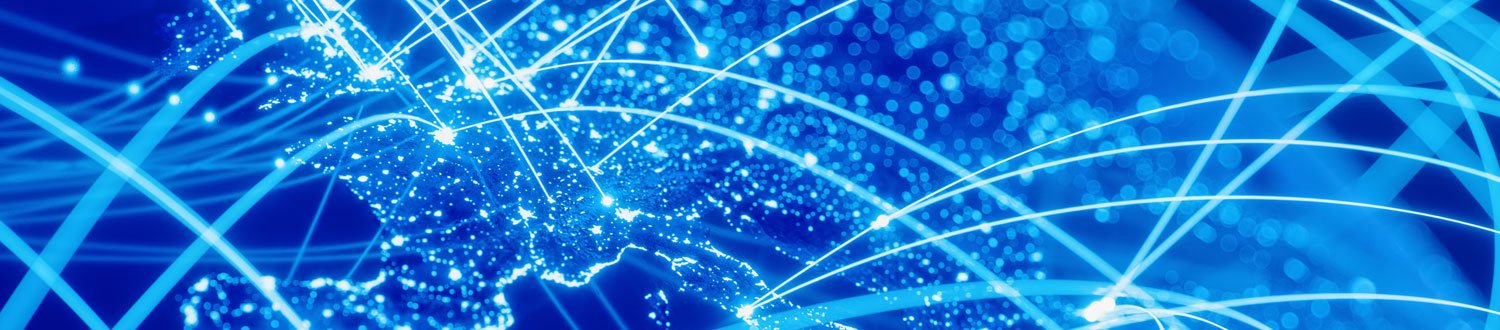
People
Our school is home to around 300 academic, professional services and technical support staff and research staff. We have over 250 PhD students, visiting lecturers and visiting researchers and more than 1,100 undergraduate and MSc students.
Our staff and students
Find out more about our staff and students and get in contact.