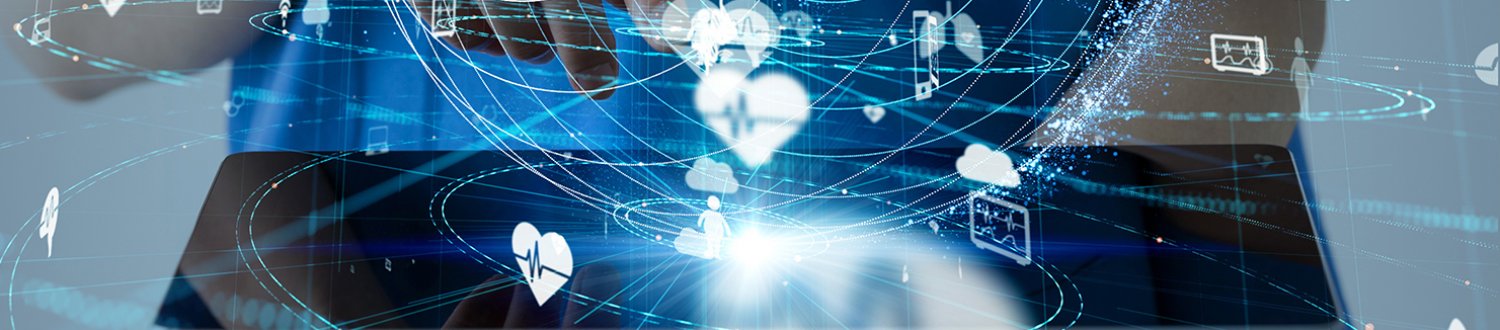
People
Find out more about our staff.
Faculty Executive Board
Executive Dean
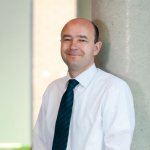
Professor Roberto La Ragione
is interim Pro-Vice Chancellor Executive Dean for FHMS
Associate deans
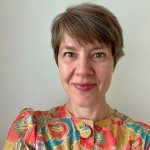
Professor Kathrin Cohen Kadosh
Associate Dean (Doctoral College) and Associate Professor Developmental Cognitive Neuroscience
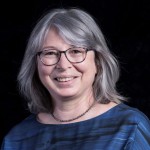
Professor Deborah Dunn-Walters
Walters Associate Dean (Research & Innovation) and Professor of Immunology
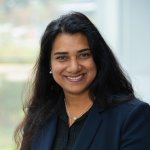
Dr Dynatra Subasinghe
Associate Dean (Education) and Senior Lecturer in Small Animal Clinical Practice
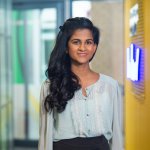
Dr Shelini Surendran
Associate Dean (International) and Associate Professor in Biosciences
Heads of schools
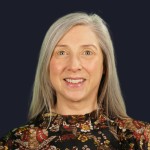
Professor Aimee Aubeeluck
Head of School of Health Sciences and Professor of Health Psychology
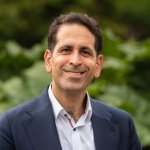
Professor Roi Cohen Kadosh
Head of School of Psychology and Professor of Cognitive Neuroscience
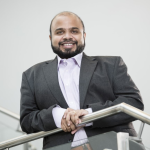
Professor Kamalan Jeevaratnam
Head of School of Veterinary Medicine and Professor of Clinical Physiology
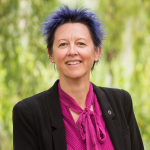
Professor Christine Rollier
Interim Head of School of Biosciences
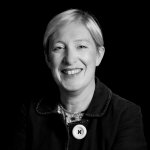
Professor Juliet Wright
Founding Dean of the School of Medicine
Professional services staff
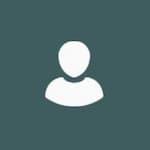
Natalie Downey
HR Business Partner
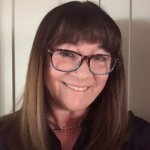
Deborah Lawson
Director of Faculty Operations
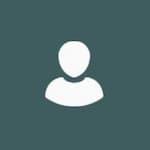
Emma Reynolds
Faculty Finance Manager
Staff by school
Visit our school pages to find out more about staff in the Faculty of Health and Medical Sciences.