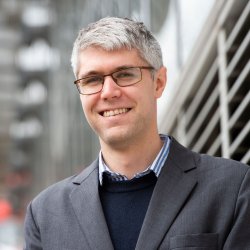
Dr Michael Short
Academic and research departments
School of Chemistry and Chemical Engineering, Institute for Sustainability.About
Biography
Dr Michael Short is an Associate Professor of Process Systems Engineering at the University of Surrey in the School of Chemistry and Chemical Engineering and Sustainability Fellow in the Surrey Institute for Sustainability. His research expertise are in the development mathematical optimisation tools to create software for process systems for automated optimal sustainable, process design, renewable energy systems, policymaking, process integration, data analysis, and process control. His research team works on applying mathematical modelling and optimisation techniques to develop software for decision support in a wide range of fields including energy planning, bioenergy, renewable energy, water, heat integration, reactor design, real-time optimisation of industrial processes, and pharmaceuticals. Dr Short's group collaborates with many academic, government and industrial partners to create impact from his research while making fundamental contributions in algorithms for mathematical optimisation and surrogate modelling. Dr Short was a 2021 EPSRC Impact Acceleration Account Commercialisation Fellow, is an Associate Member of the EPSRC Peer Review College, a Sustainability Fellow in the Surrey Institute for Sustainability, and an Associate Member of the Surrey Institute for People-Centred Artificial Intelligence. His work has been funded by EPSRC, BBSRC, Innovate UK, FCDO, British Council, CAPES (Brazil), NRF (South Africa), and industry.
Dr Short is currently the Principal Investigator for the £1.7 million EPSRC-funded project 'Artificial Intelligence Enabling Future Optimal Flexible Biogas Production for Net-Zero' (EP/Y005600/1) under the scheme Artificial Intelligence Enabling the UK's Net-Zero Targets.He leads the consortium involving Universities of Surrey, Southampton, Manchester, and Nottingham to create digital solutions for anaerobic digestion using artificial intelligence, site-wide optimisation, experimental programmes, life cycle assessment, and microbial community characterisation. Dr Short is also a Co-I in the £5 million Supergen Bioenergy Impact Hub, led by Prof Thornley at Aston University. He is digitalisation lead and develops new technologies for bioenergy process optimisation and digitalisation. In 2021, Dr Short was awarded 2 British Council research grants (combined £75k) to work on a trilateral research project with Malaysian and Japanese industrial and government partners to develop open-source software for long-term decarbonisation planning in Association of South East Asian Nations (ASEAN) countries in anticipation of COP26. The project developed the open-source energy planning software, DECO2. Since, the software has been expanded to include emissions trading systems and has been funded by the FCDO's RIDA scheme (£60k) to study Malaysian and Philippines energy systems with DECO2. Dr Short was a co-investigator on the https://www.surrey.ac.uk/research-projects/heat4all-economics-informed-optimisation-model-future-equitable-decarbonised-distributed-heating, (£50k) together with Dr Lirong Liu, Dr Mona Chitnis, and Prof Matt Leach, which worked with Woking Borough Council to develop optimisation models and system analysis to help inform policy and understand which technologies should be installed in social housing in Surrey to help the council to reach their decarbonisation targets. Dr Short, with Dr Oleksiy Klymenko, worked with Direk Ltd., funded by the Surrey Research Park's Collaborate scheme (combined £25k), to develop real-time optimisation and model predictive control algorithms with the help of machine learning techniques to minimise risks of viral infection and heating and cooling energy use in commercial buildings. This work is now funded as part of Direk's £1 million Trustworthy AI Innovate UK grant for building management systems. Dr Short also works with the NHS, University of Surrey and University of Edinburgh Estates and Facilities Management to help plan and design future decarbonised infrastructure to help the University campus reach their net-zero ambitions, obtaining funding from the Institute for Sustainability's Innovation Contest, and the EPSRC's Network+ on Decarbonisation of Heating and Cooling. Michael is also the PI of an SME Innovation Voucher project (£12k), together with Dr Melis Duyar, working to help Langham Brewery improve the sustainability of their beers. Dr Short also works with Dr Melis Duyar on the EPSRC-funded Adventurous Energy Grant (£250k) to help develop catalytic technologies for specialty chemicals from direct air capture of carbon dioxide.
Dr Short received his B.Sc. (2011) and Ph.D. (2017) from the University of Cape Town in South Africa developing new approaches for optimising heat exchanger networks and pollutant recovery systems. After being awarded his PhD, Dr Short spent 2 years working as a Postdoctoral Research Fellow at the Center for Advanced Process Decision-making (CAPD) at Carnegie Mellon University with Prof. Lorenz T. Biegler in Pittsburgh. Their work focused on developing an open-source software package, KIPET, for chemists at Eli Lilly and Company for kinetic parameter estimation from spectra for use in drug development. Michael has worked on projects involving companies such as Eli Lilly, Pfizer, Dow Chemicals, and Carrier, and is a regular contributor for many leading journals and conferences in his field. He is an Editorial Board Member for the Journal of Water Process Engineering and Journal of Process Integration and Optimization for Sustainability, as well as a Topical coordinator for the European Federation of Chemical Engineers' Sustainability Section.
Areas of specialism
University roles and responsibilities
- Responsible for Departmental Media and Marketing
- EPSRC Impact Acceleration Account Commercialisation Fellow - 2021
- Sustainability Fellow in the Institute for Sustainability
- Associate Member of the Institute for People-Centred AI
- Departmental Outreach Lead
My qualifications
Previous roles
Affiliations and memberships
Business, industry and community links
Academic networks
Doctoral studentships:
We are always looking for motivated people to join our team. If you have an interest in computational modelling, software development, or mathematical optimisation to solve real-world problems in chemical engineering, supply chains, or energy, please enquire. If there are no studentships currently available on the Surrey website, we are willing to support and guide the application process via a number of scholarships, both internal and external.
Postdoctoral positions:
Postdoctoral opportunities are currently available to work on computational modelling and optimisation chemical systems and sustainable energy technologies. Our group develops software for decision-making, policy, control, and design of industrial processes and supply chains. We offer a supportive and flexible work environment.
Those interested in postdoctoral research in our diverse and friendly team are encouraged to inquire about possible opportunities and national or international postdoctoral fellowships (Newton Funding, Leverhulme Funds, Commonwealth Royal Commission, etc.). Please get in touch and we would happily support and guide the application process. More information on some postdoctoral funding routes are below:
Newton International Fellowships: https://royalsociety.org/grants-schemes-awards/grants/newton-international/
Royal Commission for the Exhibition of 1851: https://www.royalcommission1851.org/awards/?award=research
NERC Independent Research Fellowships: https://nerc.ukri.org/funding/available/fellowships/irf/
EPSRC Fellowships: https://epsrc.ukri.org/skills/fellows/
News
In the media
ResearchResearch interests
Dr Michael Short's research interests are in developing custom optimisation modelling approaches for a wide array of applications. His current applications include for the design of chemical processing units and integrated processes, supply chain optimisation, bioenergy, the design of distributed energy systems and renewables-integrated processes, building management, as well as in parameter estimation, data analysis and process control. His team uses mixed-integer nonlinear programming (MINLP), mixed-integer linear programming (MILP), and nonlinear programming (NLP) combined with machine learning models and artificial intelligence to create software, techniques and algorithms to solve large-scale problems with relevance to industries as wide-ranging as bioprocesses, pharmaceuticals, water treatment, petrochemicals, food and beverage, and energy systems.
Research projects
This research aims at developing a practical model to understand how fuel poverty could be minimised in the UK whilst simultaneously delivering upon net-zero targets for home heating.
- Start date: 1 November 2021
- End date: 30 April 2022.
Optimisation of Distributed Energy SystemsDistributed Energy Systems (DES) have been increasingly investigated as a viable alternative to ageing centralised energy networks. Optimisation models presented in literature for the design and operation of distributed energy systems often exclude the inherent nonlinearities, related to power flow and generation and storage units, to maintain an accuracy-complexity balance. Such models may provide sub-optimal or even infeasible designs and dispatch schedules. In DES, optimal power flow (OPF) is often treated as a standalone problem, consisting of highly nonlinear, nonconvex constraints related to the underlying distribution network. This aspect of the optimisation problem has often been overlooked by researchers in the process systems and optimisation area.
This project, with Ishanki De Mel, aims to address the disparity between OPF and DES models, highlighting the importance of including elements of OPF in DES design and operational models to obtain feasible designs and operational schedules. The literature review identifies key works that have attempted to do so, and highlights several gaps that have remained despite these efforts. A methodology is proposed to develop new models that are capable of maintaining the accuracy-complexity balance, while consolidating DES and OPF and including detailed representations of key components (such as batteries). It involves three modelling routes, each designed to investigate how detailed modelling can impact DES objectives, designs, and operating schedules. Current model formulations are implemented and tested, where preliminary results shed light on the multi-faceted nature of DES and the need to make detailed optimisation models available to stakeholders who are interested in consolidating them. Finally, the project plan outlines how this methodology will be executed over the remaining years of the PhD, and the potential research outputs upon successful completion.
This British Council-funded project (GBP 50k) is a research collaboration led by University of Surrey's Dr Michael Short together with University of Nottingham Malaysia (Prof Dominic Foo) and University of Tokyo (Assoc. Prof Yasunori Kikuchi). The COP26 summit taking place in 2021 aims to accelerate the achievement of the Paris Agreement’s goals. To achieve these ambitious emissions targets, strategic planning methods for policymaking are essential. These should span entire nations’ emissions contributions, across sectors, and should be able to plan for achievable implementation of emissions reduction technologies, negative emissions technologies, within budgetary, time, and social uptake constraints.
ASEAN countries, as developing economies, have seen dramatic rises in CO2 emissions over the past 20 years (e.g. The CO2 per capita of Malaysia has risen from 5 t/y in 2000 to 8 t/y in 2018), and therefore it is important to develop tools that incorporate region-specific conditions. The project seeks to develop a decision-making software framework, based on rigorous mathematical optimisation models, for planning the decarbonisation of ASEAN countries, in line with commitments made while signing the Paris Agreement (PA).
The planning framework relies on a combination of proven, mature technologies such as the Carbon Emission Pinch Analysis (CEPA), developed by members of the project team over the past 10 years, and novel mathematical optimisation-based tools that provide rigorous guarantees on the qualities of the solution, subject to planning constraints such as budget, social resistance to uptake, efficiencies of interventions, and implementation time. The team will deliver significant outreach and engagement activities through multi-day workshops with project partners in emissions-intensive industries in Malaysia, as well as with government agencies to ensure that the software and solutions are data-driven, implementable and align with national strategies.
Learn more about the project:
Studying interactions between the catalytic upgrading of biomethane and fuel cells to obtain optimal flowsheetsIn this project, together with Anamika Kushwah and Dr Tomas Ramirez Reina, we use a combination of superstructure based flowsheet optimisation and experimental work to study and improve advanced energy conversion of biomass and next-generation SOFC (solid oxidised fuel cell) for green electricity production. The project includes modelling and simulation a hybrid hydrogen fuel cell and biofuel energy production system, including gasification and Fischer-Tropsch catalysis from biomass, using a combination of advanced optimisation modelling techniques and experimental work.
Together with Dr Oleksiy Klymenko, we develop models and techniques to estimate the viral transfer between individuals, objects, and the air in enclosed settings based on all known transmission pathways to determine which pathways are dominant towards risk of infection in specific scenarios and to quantitatively deterine the effect of various mitigation methods. Our model has been incorporated into a real-time application within Direk Ltd's sensing technologies to provide real-time information for building managers to reduce infections.
Optimization of integrated energy systems in aquacultureAquaculture is a fast-growing industry and is becoming an important source of protein, with this growth expected to continue. Recirculated aquaculture systems (RAS) are the most common and critical technology that can reuse the water in the system and be recirculated. RAS control the indoor environment to make harvesting times predictable and maximize the yield. The quality and temperature control of water makes the energy consumption of RAS large, so energy management is an essential part of system design. It is therefore important to establish an economic and sustainable electricity-heat integrated energy system for aquaculture.
Renewable energy has low carbon emissions, sustainability benefits, and low generation costs. However, the nature of renewable generation is stochastic, and inaccuracy of output forecast brings a significant challenge to system operations, which brings risks to the system and markets. To determine the operation schedule, such as the unit on or off status and generators production level, the unit commitment (UC) optimization problem is solved a day ahead to ensure that power generators meet the forecasted load at a minimum cost of system operation. AC optimal power flow is a nonconvex and complex problem and so is computationally expensive and cannot guarantee global solutions. Developed algorithms need to ensure fast computation to global optimality for real-time applications. In addition, these models do not typically explicitly consider the uncertainty in the model and forecasts.
Optimization under uncertainty in large-scale power systems operations with renewable energy has recently become a topic of significant interest. This project, together with Ruosi Zhang, we will develop a data-driven algorithm, to solve the model predictive control problem of optimal power flow and unit commitment problem for a RAS, in order to minimize the costs associated with these complex systems.
Sustainable BreweriesWe are developing optimisation-based software and techniques to analyse microbrewery energy usage and process flows to perform life cycle assessment and identify process improvements and energy saving retrofits to make microbreweries more sustainable. The first project, funded by an SME Innovation Voucher, is with Langham Breweries in the South Down National Park. We have helped them to identify optimal ways to reduce energy consumption, retrofit renewable energy technologies, and suggested process improvements to lower the carbon footprints and processing costs of their beers.
Dr Short works together with Dr Melis Duyar's Adventurous Energy Project (https://www.ukri.org/news/adventurous-ideas-to-make-net-zero-a-reality/), which aims to pull the building blocks of important chemicals, such as carbon or nitrogen, directly from the air for the carbon-negative creation of chemicals for fuels, fertilisers and consumer products. His contribution is in developing system models and optimal experimental design techniques to help guide the development of novel processes and catalysts.
Research collaborations
Dr Short regularly collaborates with industrial and academic partners from around the world, with active projects with:
- Prof Lorenz T. Biegler (Carnegie Mellon University)
- Assoc Prof. Adeniyi Isafiade (University of Cape Town)
- Prof Dominic Foo (University of Nottingham Malaysia)
- Dr Salvador Garcia-Munoz (Eli Lilly, Imperial College London, Carnegie Mellon University)
- Prof Raymond Tan (De La Salle University)
Indicators of esteem
Best Speaker Award (First Place) - The 4th Sustainable Process Integration Laboratory Scientific Conference, Energy, Water, Emission & Waste in Industry and Cities, 2020
Member of EPSRC Early Career Forum
Topic Editor for MDPI's Processes Journal's Reviewer Board
Review Editor for Frontiersin Sustainable Chemical Process Design
Session Chair for American Institute of Chemical Engineers (AIChE) Annual Meeting 2021, Boston.
Associate Member of the Surrey Centre for People-Centred Artificial Intelligence
2021 Surrey IAA Commercialisation Fellow
Associate College Member of the EPSRC Peer Review College
Digitalisation lead in the Supergen Bioenergy Impact Hub
Fellow of the Surrey Institute for Sustainability
Research interests
Dr Michael Short's research interests are in developing custom optimisation modelling approaches for a wide array of applications. His current applications include for the design of chemical processing units and integrated processes, supply chain optimisation, bioenergy, the design of distributed energy systems and renewables-integrated processes, building management, as well as in parameter estimation, data analysis and process control. His team uses mixed-integer nonlinear programming (MINLP), mixed-integer linear programming (MILP), and nonlinear programming (NLP) combined with machine learning models and artificial intelligence to create software, techniques and algorithms to solve large-scale problems with relevance to industries as wide-ranging as bioprocesses, pharmaceuticals, water treatment, petrochemicals, food and beverage, and energy systems.
Research projects
This research aims at developing a practical model to understand how fuel poverty could be minimised in the UK whilst simultaneously delivering upon net-zero targets for home heating.
- Start date: 1 November 2021
- End date: 30 April 2022.
Distributed Energy Systems (DES) have been increasingly investigated as a viable alternative to ageing centralised energy networks. Optimisation models presented in literature for the design and operation of distributed energy systems often exclude the inherent nonlinearities, related to power flow and generation and storage units, to maintain an accuracy-complexity balance. Such models may provide sub-optimal or even infeasible designs and dispatch schedules. In DES, optimal power flow (OPF) is often treated as a standalone problem, consisting of highly nonlinear, nonconvex constraints related to the underlying distribution network. This aspect of the optimisation problem has often been overlooked by researchers in the process systems and optimisation area.
This project, with Ishanki De Mel, aims to address the disparity between OPF and DES models, highlighting the importance of including elements of OPF in DES design and operational models to obtain feasible designs and operational schedules. The literature review identifies key works that have attempted to do so, and highlights several gaps that have remained despite these efforts. A methodology is proposed to develop new models that are capable of maintaining the accuracy-complexity balance, while consolidating DES and OPF and including detailed representations of key components (such as batteries). It involves three modelling routes, each designed to investigate how detailed modelling can impact DES objectives, designs, and operating schedules. Current model formulations are implemented and tested, where preliminary results shed light on the multi-faceted nature of DES and the need to make detailed optimisation models available to stakeholders who are interested in consolidating them. Finally, the project plan outlines how this methodology will be executed over the remaining years of the PhD, and the potential research outputs upon successful completion.
This British Council-funded project (GBP 50k) is a research collaboration led by University of Surrey's Dr Michael Short together with University of Nottingham Malaysia (Prof Dominic Foo) and University of Tokyo (Assoc. Prof Yasunori Kikuchi). The COP26 summit taking place in 2021 aims to accelerate the achievement of the Paris Agreement’s goals. To achieve these ambitious emissions targets, strategic planning methods for policymaking are essential. These should span entire nations’ emissions contributions, across sectors, and should be able to plan for achievable implementation of emissions reduction technologies, negative emissions technologies, within budgetary, time, and social uptake constraints.
ASEAN countries, as developing economies, have seen dramatic rises in CO2 emissions over the past 20 years (e.g. The CO2 per capita of Malaysia has risen from 5 t/y in 2000 to 8 t/y in 2018), and therefore it is important to develop tools that incorporate region-specific conditions. The project seeks to develop a decision-making software framework, based on rigorous mathematical optimisation models, for planning the decarbonisation of ASEAN countries, in line with commitments made while signing the Paris Agreement (PA).
The planning framework relies on a combination of proven, mature technologies such as the Carbon Emission Pinch Analysis (CEPA), developed by members of the project team over the past 10 years, and novel mathematical optimisation-based tools that provide rigorous guarantees on the qualities of the solution, subject to planning constraints such as budget, social resistance to uptake, efficiencies of interventions, and implementation time. The team will deliver significant outreach and engagement activities through multi-day workshops with project partners in emissions-intensive industries in Malaysia, as well as with government agencies to ensure that the software and solutions are data-driven, implementable and align with national strategies.
Learn more about the project:
In this project, together with Anamika Kushwah and Dr Tomas Ramirez Reina, we use a combination of superstructure based flowsheet optimisation and experimental work to study and improve advanced energy conversion of biomass and next-generation SOFC (solid oxidised fuel cell) for green electricity production. The project includes modelling and simulation a hybrid hydrogen fuel cell and biofuel energy production system, including gasification and Fischer-Tropsch catalysis from biomass, using a combination of advanced optimisation modelling techniques and experimental work.
Together with Dr Oleksiy Klymenko, we develop models and techniques to estimate the viral transfer between individuals, objects, and the air in enclosed settings based on all known transmission pathways to determine which pathways are dominant towards risk of infection in specific scenarios and to quantitatively deterine the effect of various mitigation methods. Our model has been incorporated into a real-time application within Direk Ltd's sensing technologies to provide real-time information for building managers to reduce infections.
Aquaculture is a fast-growing industry and is becoming an important source of protein, with this growth expected to continue. Recirculated aquaculture systems (RAS) are the most common and critical technology that can reuse the water in the system and be recirculated. RAS control the indoor environment to make harvesting times predictable and maximize the yield. The quality and temperature control of water makes the energy consumption of RAS large, so energy management is an essential part of system design. It is therefore important to establish an economic and sustainable electricity-heat integrated energy system for aquaculture.
Renewable energy has low carbon emissions, sustainability benefits, and low generation costs. However, the nature of renewable generation is stochastic, and inaccuracy of output forecast brings a significant challenge to system operations, which brings risks to the system and markets. To determine the operation schedule, such as the unit on or off status and generators production level, the unit commitment (UC) optimization problem is solved a day ahead to ensure that power generators meet the forecasted load at a minimum cost of system operation. AC optimal power flow is a nonconvex and complex problem and so is computationally expensive and cannot guarantee global solutions. Developed algorithms need to ensure fast computation to global optimality for real-time applications. In addition, these models do not typically explicitly consider the uncertainty in the model and forecasts.
Optimization under uncertainty in large-scale power systems operations with renewable energy has recently become a topic of significant interest. This project, together with Ruosi Zhang, we will develop a data-driven algorithm, to solve the model predictive control problem of optimal power flow and unit commitment problem for a RAS, in order to minimize the costs associated with these complex systems.
We are developing optimisation-based software and techniques to analyse microbrewery energy usage and process flows to perform life cycle assessment and identify process improvements and energy saving retrofits to make microbreweries more sustainable. The first project, funded by an SME Innovation Voucher, is with Langham Breweries in the South Down National Park. We have helped them to identify optimal ways to reduce energy consumption, retrofit renewable energy technologies, and suggested process improvements to lower the carbon footprints and processing costs of their beers.
Dr Short works together with Dr Melis Duyar's Adventurous Energy Project (https://www.ukri.org/news/adventurous-ideas-to-make-net-zero-a-reality/), which aims to pull the building blocks of important chemicals, such as carbon or nitrogen, directly from the air for the carbon-negative creation of chemicals for fuels, fertilisers and consumer products. His contribution is in developing system models and optimal experimental design techniques to help guide the development of novel processes and catalysts.
Research collaborations
Dr Short regularly collaborates with industrial and academic partners from around the world, with active projects with:
- Prof Lorenz T. Biegler (Carnegie Mellon University)
- Assoc Prof. Adeniyi Isafiade (University of Cape Town)
- Prof Dominic Foo (University of Nottingham Malaysia)
- Dr Salvador Garcia-Munoz (Eli Lilly, Imperial College London, Carnegie Mellon University)
- Prof Raymond Tan (De La Salle University)
Indicators of esteem
Best Speaker Award (First Place) - The 4th Sustainable Process Integration Laboratory Scientific Conference, Energy, Water, Emission & Waste in Industry and Cities, 2020
Member of EPSRC Early Career Forum
Topic Editor for MDPI's Processes Journal's Reviewer Board
Review Editor for Frontiersin Sustainable Chemical Process Design
Session Chair for American Institute of Chemical Engineers (AIChE) Annual Meeting 2021, Boston.
Associate Member of the Surrey Centre for People-Centred Artificial Intelligence
2021 Surrey IAA Commercialisation Fellow
Associate College Member of the EPSRC Peer Review College
Digitalisation lead in the Supergen Bioenergy Impact Hub
Fellow of the Surrey Institute for Sustainability
Supervision
Postgraduate research supervision
Optimisation of Distributed Energy Systems
Postgraduate Researcher: Ishanki A. De Mel
Distributed Energy Systems (DES) have been increasingly investigated as a viable alternative to ageing centralised energy networks. Optimisation models presented in literature for the design and operation of distributed energy systems often exclude the inherent nonlinearities, related to power flow and generation and storage units, to maintain an accuracy-complexity balance. Such models may provide sub-optimal or even infeasible designs and dispatch schedules. In DES, optimal power flow (OPF) is often treated as a standalone problem, consisting of highly nonlinear, nonconvex constraints related to the underlying distribution network. This aspect of the optimisation problem has often been overlooked by researchers in the process systems and optimisation area.
This project aims to address the disparity between OPF and DES models, highlighting the importance of including elements of OPF in DES design and operational models to obtain feasible designs and operational schedules. The literature review identifies key works that have attempted to do so, and highlights several gaps that have remained despite these efforts. A methodology is proposed to develop new models that are capable of maintaining the accuracy-complexity balance, while consolidating DES and OPF and including detailed representations of key components (such as batteries). It involves three modelling routes, each designed to investigate how detailed modelling can impact DES objectives, designs, and operating schedules. Current model formulations are implemented and tested, where preliminary results shed light on the multi-faceted nature of DES and the need to make detailed optimisation models available to stakeholders who are interested in consolidating them. Finally, the project plan outlines how this methodology will be executed over the remaining years of the PhD, and the potential research outputs upon successful completion.
Research theme: Information and Process Systems Engineering, Sustainable Energy and Materials
Studying interactions between the catalytic upgrading of biomethane and fuel cells to obtain optimal flowsheets
Postgraduate Researcher: Anamika Kushwah
We study an advanced energy conversion system, combining next generation of SOFC (solid oxidised fuel cell) with biomethane catalytic upgrading for green electricity production. The study also includes modelling and simulation to first identify optimal potential flowsheets and technologies to identify promising reactor conditions and technology combinations to generate a hybrid hydrogen fuel cell and biofuel energy production system including gasification and Fischer-Tropsch catalysis from biomass using a combination of advanced optimisation modelling techniques and experimental work.
Research theme: Information and Process Systems Engineering, Sustainable Energy and Materials
Optimisation of integrated energy systems in aquaculture
Postgraduate Researcher: Ruosi Zhang
Aquaculture is a fast-growing industry and is becoming an important source of protein, with this growth expected to continue. Recirculated aquaculture systems (RAS) are the most common and critical technology that can reuse the water in the system and be recirculated. RAS control the indoor environment to make harvesting times predictable and maximize the yield. The quality and temperature control of water makes the energy consumption of RAS large, so energy management is an essential part of system design. It is therefore important to establish an economic and sustainable electricity-heat integrated energy system for aquaculture.
Renewable energy has low carbon emissions, sustainability benefits, and low generation costs. However, the nature of renewable generation is stochastic, and inaccuracy of output forecast brings a significant challenge to system operations, which brings risks to the system and markets. To determine the operation schedule, such as the unit on or off status and generators production level, the unit commitment (UC) optimization problem is solved a day ahead to ensure that power generators meet the forecasted load at a minimum cost of system operation. AC optimal power flow is a nonconvex and complex problem and so is computationally expensive and cannot guarantee global solutions. Developed algorithms need to ensure fast computation to global optimality for real-time applications. In addition, these models do not typically explicitly consider the uncertainty in the model and forecasts.
Optimization under uncertainty in large-scale power systems operations with renewable energy has recently become a topic of significant interest. This project will develop a data-driven algorithm, to solve the model predictive control problem of optimal power flow and unit commitment problem for a RAS, in order to minimize the costs associated with these complex systems.
Research theme: Information and Process Systems Engineering
Completed postgraduate research projects I have supervised
Graduated Research Students:
2023
PhD
Ishanki De Mel - Optimal Design of Grid-Connected Distributed Energy Systems with Optimal Power Flow
MSc
Gul Hameed - A Long-term Energy Planning Framework incorporating various Energy Resources, Carbon Trading and other Emission Reduction Technologies
Oluwatobi Ogunsanya - Model Predicitve Control of Building Heating Systems
Ayoub Alnasseri - The Impact of Decentralisation of Electricity Generation on Demand and Tariff in Oman
Yasaman Barati - The role of biogas in the UK energy market
A decision-making framework for assessing biogas upgrading
MEng
Irene Constantinidou - Designing a Net-Zero Microbrewery
Jaskirat Singh - Investigating the Impacts of Uncertainties in Negative Emissions Technologies on Energy Modelling
Mya Ferguson - TEMICO – Transport Emissions and Cost Optimisation Model: Evaluating Carbon Pricing Policies for Stakeholders in Developing Regions
Rohit Murali - A Roadmap to Net Zero for Microbreweries: MILP Optimisation Model for Retrofit Technology Selection
Wein Zhong Han - Incorporating Resistance to Change into Transport Sector Modelling to Achieve Net Zero Emissions
Salah Buul
Tharminy Tharmakulasingam
Kieran McDonagh
2022
MSc
Zain Mahmood - An optimisation algorithm for detailed shell-and-tube heat exchanger designs for multi-period operation
Obaid Khan - Simulation-based design of a distributed solar power generation plant
Ekpavee Pichadulya - Sustainable beer? Developing tools to help local brewery reduce their carbon footprints
Pushpavani Marugesan - The role of digestate in the circular bioeconomy
MEng
Josh Fearns -Investigating the impacts of uncertainties on energy modelling
Parham Roshani - Modelling and multi-objective optimization of variable air/oxygen gasification performance parameters utilising woody biomass using ASPEN Plus and MATLAB, by interlinking through MS Excel
Alaa Hasrat - Biomass gasification using municipal solid waste (MSW): Impacts of variable gasifying agents and optimisation via genetic algorithm (GA)
Alexander Delaney - Semi-Mechanistic Pharmacokinetic Model for Gastric Emptying using [13C] Palmitic Acid Tracer
Harry Chapman - Assessing insulation performance with respect to time in an MILP Distributed Energy System optimisation for residential buildings
Callum Harling - The Impact of Hourly Resolution Insulation Data for A Heating System and Its Effectiveness On Cost and Emissions.
Ken Paulo
Iaroslav Bobrov
2021
MSc
Floris Bierkens - Modelling of consumer heating technologies for optimal design of distributed energy systems
Danyal Suhail - Creating a policymaking planning tool for UK Decarbonisation
Jerrick Athappilly - Simultaneous synthesis of work exchange networks with heat integration while considering pressure drops through heat exchangers
Ghafoor Hussain - Numerical Modelling of CO2 capture and in situ conversion to CH4 on Dual-Functional Materials using Python
MEng
Kojo Asamoah
Marwan Elnesr
Sachin Patel
Joe Prollins
Erwin Stewart
Grishnna Ravinthiran
2020
MSc
Anandmoy Jana - Designing Supply Chains in the Digital Era
MEng
Hasan Amjad
Saim Rafiq
2019
MSc
Somang Kim (University of Cape Town) - The Synthesis of Combined Heat and Mass Exchange Networks (CHAMENs) With Renewables Considering Environmental Impact
Teaching
Currently teach the following subjects
Semester 1:
- Process dynamics, ENG2120 (Year 2)
- Computation for Chemical Engineers, ENG2127 (Year 2, Module Leader)
- Industrial Systems, ENG3186 (Year 3, Module Leader)
Semester 2:
- Capital cost estimating and economic evaluation of projects for final Chemical Engineering design project (Year 3/4)
- Sustainability of projects for final Chemical Engineering design project (Year 3/4)
Sustainable development goals
My research interests are related to the following:
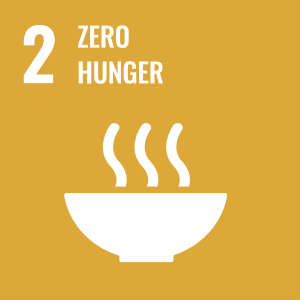
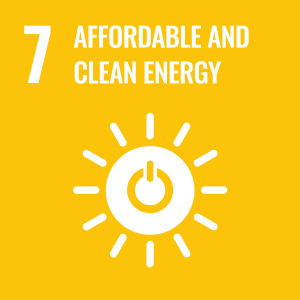
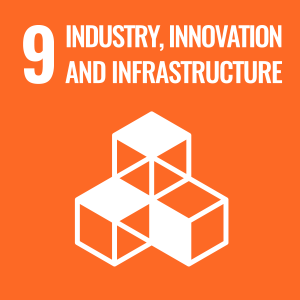
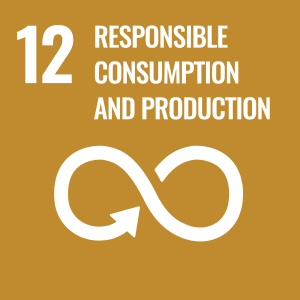
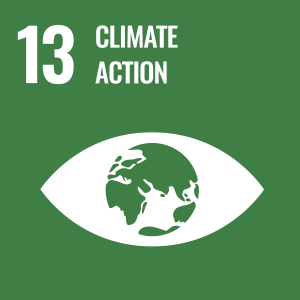
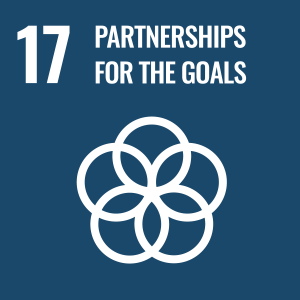
Publications
Gastric emptying (GE) is the process of food being processed by the stomach and delivered to the small intestine where nutrients such as lipids are absorbed into the blood circulation. The combination of an easy and inexpensive method to measure GE such as the CO2 breath test using the stable isotope [ 13 C]octanoic acid with semi-mechanistic modelling could foster a wider application in nutritional studies to further understand the metabolic response to food. Here, we discuss the use of the [ 13 C]octanoic acid breath test to label the solid phase of a meal, and the factors that influence GE to support mechanistic studies. Furthermore, we give an overview of existing mathematical models for the interpretation of the breath test data and how much nutritional studies could benefit from a physiological based pharmacokinetic model approach.
Designing dual function materials (DFMs) entails an optimisation of CO 2 adsorption and catalytic conversion activity, often requiring a large number of experimental parametric studies screening various types and loadings of adsorbent and catalyst components. In this study, we used a Gaussian process model optimised with Bayesian optimisation (BO) to find the DFM composition leading to the highest methanation activity. We focused on optimising Na (adsorbent) loading in a DFM where Na loading was varied from 2.5–15% by weight. The results from the experimental tests indicated that the sample with the highest Na-loading (15 wt%) possessed the highest CO 2 desorption during CO 2 -TPD, however, it was not the best DFM, as it did not show the highest methane production. By testing Bayesian optimisation recommended experiments we identified 7.9 wt% Na as the optimal Na loading, which showed the highest methane production for a cycle (398.6 μmol g DFM −1 ) at 400 °C. This forms a case study for how BO can help accelerate materials discovery for DFMs.
Energy storage is important in future power systems. However, the role of grid-scale energy storage in the power system and in the whole socio-economic system is unclear. A copula-based whole system model is developed to explore the economic and environmental effects of grid-scale energy storage, thus supporting the decision-making at micro and macro levels. A power system optimisation model is linked with an input-output model, and the copula function is embedded in the model to reflect the multiple and interactive uncertainties from electricity demand, emission constraints, and sector disaggregation. We conducted case studies on China and the UK in 2025 considering different storage technologies (Pumped hydro, Battery, Flywheels storage) to show the differences related with power systems and economic structures. We find that increasing energy storage capacity leads to increase in renewable generation capacity (solar generation in China and wind generation in the UK). Thus, it can reduce their total economy-wide carbon emissions. Uncertainty in sector disaggregation will have a large impact on carbon emissions in some extreme cases, especially in those sectors closely linked to the power sector and with high emission intensity.
This study evaluates two integrated pathways for synthetic natural gas (SNG) production via direct air capture (DAC) and utilisation: Dual-Function Material (DFM) technology and Temperature-Vacuum Swing Adsorption (TVSA) combined with a Sabatier reactor. DFM technology, which combines CO₂ capture and methanation in a single unit, is compared against the more established TVSA-Sabatier process regarding techno-economic feasibility. Superstructure optimisation is employed to assess the performance of these two pathways across various upstream and downstream operating units and to examine the impact of different design factors on economic outcomes. For a capturing scenario of 10,000 tCO2/year, results indicate that DFM technology presents promise, reaching an estimated cost of $740/tCO2 (assuming a 7% interest rate) under optimal conditions, comparable to the TVSA-Sabatier pathway. Sensitivity analysis underscores the importance of interest rates, energy prices, and carbon credits, highlighting the potential of policy support in facilitating DFM technology. Comparative findings suggest that DFM can potentially reduce equipment complexity and energy use through in situ heat integration however, it requires further thermo-kinetic analysis and experimental validation. Future research is needed on kinetic modelling of DFM's and advancements are also required in material adsorption performance, more cost-effective catalyst alternatives, and addressing limitations related to pressure drop in further process intensification efforts. This study offers a comprehensive benchmark for DAC-to-SNG processes, indicating that while DFM technology demonstrates potential for streamlined operations and cost savings, targeted advancements are essential for commercial viability, contributing critical insights to sustainable carbon capture and utilisation strategies.
In this study, we used fermentation off-gases from a brewery for integrated CO2 capture and utilisation in order to produce CH4 with a dual-function material (DFM) containing NiRu as catalyst and dispersed CaO as adsorbent. CH4 was produced from captured CO2 via 2 pathways (fast and slow), proceeding through formyl intermediates according to the operando DRIFTS-MS results. The NiRuCa DFM showed a stable CH4 capacity over 8 cycles (105 μmol/gDFM) with fermentation off-gases being used as a CO2 capture feed. H2O and O2, which were present in small amounts in the emissions feed, resulted in the passivation of Ni in the form of a NiO layer that protected the DFM from excessive oxidation and deactivation. This work constitutes a first in terms of validating the use of DFMs with real industrial emissions, and it directly correlates the DFM activity performance with its reaction mechanism and intermediate species.Those are the data generated for our collaborative project with Spain. All the data are given in excel files except of some figures that are png format. The uploaded data also include the data used in the supplementary information and those figures and tables are denoted with an 'S' before the number. The data include the activity data of a sample under real conditions, its reaction mechanism under ideal and realistic conditions, and its physiochemical characterisation.
Experimental inversion of circadian and behavioural rhythms by 12-hours adversely affects markers of metabolic health. We investigated the effects of a more modest 5-hour delay in behavioural cycles. Fourteen participants completed an 8-day in-patient laboratory protocol, with controlled sleep-wake opportunities, light-dark cycles, and diet. The 5-hour delay in behavioural cycles was induced by delaying sleep opportunity. We measured: melatonin to confirm central circadian phase; fasting markers and postprandial metabolism; energy expenditure; subjective sleepiness; and appetite, throughout the waking period. After the phase delay, there was slower gastric emptying at breakfast, lower fasting plasma glucose, higher postprandial plasma glucose and triglycerides, and lower thermic effect of feeding. Any changes were abolished or attenuated within 48-72 hours. These data extend our previous findings, which showed no time-of-day effect in healthy adults on daytime energy expenditure or thermic effect of feeding when accounting for circadian variation in resting metabolic rate.
Beer is the most produced and consumed alcoholic beverage in the world, but the agricultural production of its most common ingredient, i.e. malted barley, is a significant contributor to the overall environmental footprint of beer. In addition, food wastage, particularly bread with millions of slices wasted daily, poses a waste management challenge across the globe. This study aims to address both issues through brewing beer with waste bread that would have otherwise ended up in landfill by replacing a portion of malted barley with waste bread. A sourdough pale ale was brewed at various bread percentages to understand how the inclusion of bread changed the sugar profile and fermentability of the beer. The samples were mashed at two different temperatures, 65 °C and 70 °C, to assess the impacts of mashing. It was found that the volume of alcohol produced declined with increasing bread amounts, but brewing with up to 60 wt% bread produced the same volume of alcohol as a standard beer. A life cycle assessment was performed to quantify the change in cradle to grave environmental impact for brewing beers with varying bread percentages with the view to conduct more targeted feasibility studies in the future with waste bread substitution. Significant reductions in emissions were observed as regards global warming potential, terrestrial ecotoxicity, acidification, eutrophication, ozone depletion, and abiotic depletion of fossil fuels. In particular, the global warming potential for the real-life example microbrewery studied in this work was decreased by 7.13% of the total carbon dioxide equivalent annually, demonstrating the environmental advantages of brewing beer with waste bread.
•Microbreweries have difficulty economically competing with larger breweries.•A mixed-integer nonlinear optimisation model is developed for microbrewery retrofits.•An optimal configuration can reduce operating costs by 62.9 % and emissions by 77.1 %. Microbreweries have greater production costs per litre of beer compared to large breweries, as well as higher carbon footprints. Due to the range of different retrofit technologies available and the different capacities and configurations of microbreweries, it is not always clear what retrofits will improve operations. Therefore, this work proposes a novel mixed-integer nonlinear programming decision-making tool to be used by any microbrewery, that determines the technoeconomic feasibility and sizing of energy efficiency-improving retrofits, including solar and wind power, battery storage, anaerobic digestion, boiler type selection, heat integration by heat storage, and carbon capture via dual-function materials. The model was demonstrated on a real UK microbrewery case study. The model gave an optimal configuration of a 10 m3 anaerobic digester, 30 solar panels outputting 380 W each, an 800 W wind turbine and a 2.3 m3 heat storage tank, reducing annual operating costs by 62.9 % and carbon dioxide emissions by 77.1 % with a payback period of 8 years. The tool is designed to be flexible for use by any microbrewery in any location with any brewing recipe and allow the owner(s) to develop more profitable and sustainable microbreweries. Tweetable abstract Microbreweries can implement mathematically optimised renewable energy, heat integration and anaerobic digestion to reduce operating costs by 62.9 % and carbon emissions by 77.1 %. [Display omitted]
This study introduces Dual Function Materials (DFM) technology within the context of direct air capture (DAC) and incorporates it into a superstructure optimisation framework for optimal flowsheet development. A comparative assessment against an established DAC process, temperature vacuum swing adsorption (TVSA), underscores the potential operational and economic advantages of DFMs. The application of superstructure optimisation reveals critical determinants, including the reactor geometry, mass and heat transfer parameters, and the equilibrium CO2 capacity of the sorbent-catalyst, as crucial for evaluating the feasibility of DFMs in relation to TVSA. Apart from hydrogen production, the operational cost is significantly impacted by the pressure drop in the adsorption section, with reactor/contactor size and quantity primarily constrained by pressure drop rather than adsorption rates.
The year 2020 has seen the emergence of a global pandemic as a result of the disease COVID-19. This report reviews knowledge of the transmission of COVID-19 indoors, examines the evidence for mitigating measures, and considers the implications for wintertime with a focus on ventilation. This work was undertaken as a contribution to the Rapid Assistance in Modelling the Pandemic (RAMP) initiative, coordinated by the Royal Society.
This study integrates scalable carbon trading models into an energy planning framework to address carbon emissions challenge associated with increasing energy consumption in emerging economies. The models utilise mixed-integer nonlinear programming (MINLP) formulations to optimise power generation, emissions, and costs. A case study is demonstrated on an ASEAN (Association of Southeast Asian Nations) country, Malaysia. Results reveal that carbon trading enhances both financial gains and environmental sustainability, with direct optimisation (where emission rights or carbon prices are variables) approach proving more effective in maximising carbon markets’ efficacy, leading to better cost to emission ratios when compared to indirect optimisation (with emission rights prices as parameters, estimated via demand-supply curve). For emissions minimisation, direct optimisation resulted in cost to emissions ratios about 1.3 to 2.1 times more than those for indirect optimisation for three out of four studied periods. The study highlights the potential of coordinated emissions trading and optimal investment decision-making, providing valuable insights for energy planning in Malaysia which is aiming for net-zero target by year 2050.
In this study, we used fermentation off-gases from a brewery for integrated CO2 capture and utilisation in order to produce CH4 with a dual-function material (DFM) containing NiRu as catalyst and dispersed CaO as adsorbent. CH4 was produced from captured CO2 via 2 pathways (fast and slow), proceeding through formyl intermediates according to the operando DRIFTS-MS results. The NiRuCa DFM showed a stable CH4 capacity over 8 cycles (105 μmol/gDFM) with fermentation off-gases being used as a CO2 capture feed. H2O and O2, which were present in small amounts in the emissions feed, resulted in the passivation of Ni in the form of a NiO layer and hence, the DFM did not undergo excessive oxidation and deactivation. This work constitutes a first in terms of validating the use of DFMs with real industrial emissions, and it directly correlates the DFM activity performance with its reaction mechanism and intermediate species. [Display omitted] •The operation of a DFM is demonstrated using real CO2 emissions from a microbrewery.•Operando DRIFTS shows that CH4 is produced via a fast and a slow reaction pathway.•During operation a passivating NiO layer forms and protects the DFM from excessive oxidation.
Distributed energy systems (DES) have the potential to minimise costly network upgrades while increasing the proportion of renewable energy generation in the electrical grid, when properly designed. In contrast, poorly designed DES can accelerate the degeneration of existing network infrastructure. Most optimisation models used to design grid-connected DES have oversimplified or excluded constraints associated with alternating current (AC) power flow, as the latter has been studied in a standalone class of models known as Optimal Power Flow (OPF). A small subset of models, labelled DES-OPF models, have attempted to combine these independent frameworks. However, the impacts of using a DES-OPF framework on the resulting designs, as opposed to a conventional DES framework without AC power flow, have not been studied in previous work. This study aims to shed light on these impacts by proposing a bi-level method to solve the computationally-expensive DES-OPF framework, and simultaneously comparing results to a baseline MILP model that utilises direct current (DC) approximations in place of AC OPF, as found abundantly in literature. Two test cases of varying scale are employed to test the frameworks and compare resulting designs. The practical feasibility of the designs is assessed, based on whether the designs can mitigate network violations and energy wastage during standard operation. Results demonstrate that the baseline MILP underestimates total costs due to its inability to detect current and voltage violations, resulting in a 37% increase for one case study when tested with the DES-OPF framework. Major implications on battery capacity are also observed, primarily to manage higher levels of renewable energy curtailment, which emphasise the need to use DES-OPF frameworks when designing grid-connected DES.
The criticality of climate change is such that any further delay in mitigation action would result in irreversible damage. This work develops a novel software framework for optimal decarbonisation in energy planning to determine the optimum deployment of renewable energy sources, alternative low carbon fuels, negative emission technologies (NETs) and CO2 capture and storage (CCS). The mathematical programming-based tools in this work provide rigorous optimal solutions, subject to budget, demand, and emission constraints. The application of the software framework is demonstrated with a Malaysian energy decarbonisation case study. The results indicate a heavy reliance on NETs, alongside reductions in coal and natural gas use to achieve CO2 neutrality by 2050.
To combat severe climate change, several governments around the world have set ambitious targets to reach net-zero emissions in the coming decades. To this end, policymakers will need decarbonisation planning tools, such that a decarbonisation pathway optimised to local constraints is selected and implemented. There are several policymaking planning tools available for macro-scale planning and operations at region-wide level, however tools and research on smaller, enterprise-level scale remain limited, despite commitments being made by several large companies to become net-zero carbon emitters. This work presents an optimisation-based decarbonisation planning tool for use by industrial companies to plan for carbon emission reduction by implementing a variety of different technologies. The model can consider feedstock changes and alternative energy sources and, given a set of demands and constraints, can suggest the optimal technology selection of carbon, capture and storage (CCS), low emission energy and feedstocks, and negative emissions technologies to achieve emissions targets. Unlike previous models, the model accounts for price changes across decades and provides a plan on how companies can invest in the right technologies, either constrained by a budget or given an emissions target, while still delivering products to satisfy demands. The model is demonstrated on a case study based on ExxonMobil’s Baytown refinery complex which consists of an oil refinery, a plastics plant, an olefins plant and a chemical plant, where a net-zero emission limit is set within six 5-year periods. The pathway created by the model is able to suggest a full reduction of emissions in the chemical, plastics and olefins plant respectively within a 10-year implementation of biogas and negative emissions technologies and is able to reduce the oil refinery from 44 Mt/y of CO2 emissions to 4.5 Mt/y after 25 years by phasing out oil in favour of renewable biogas refining.
The delay in action to mitigate climate change has compounded its impacts and there is now a greater dependence on carbon dioxide removal (CDR) to achieve net-zero carbon targets by 2050. This work develops a software framework for optimal decarbonised energy planning to determine the optimum deployment of renewable energy sources, negative emission technologies (NETs), and CO2 capture and storage (CCS), which has been underexplored in previous work. The novel mathematical optimisation-based tools in this work provide rigorous guarantees on the qualities of the solution, subject to planning constraints i.e., budget etc. The application of the software framework is demonstrated with a case study containing seven power plants. In this multiperiod work, CCS deployment is favoured for coal-based power plants due to its highest carbon intensity, while energy-producing NETs (EP-NETs) is deployed for all periods.
A dependable and sustainable energy supply is crucial as energy consumption continues to rise due to population growth, economic development, and improved living standards. The use of fossil fuels leads to CO2 emissions and are subject to volatility in prices. Capital-intensive technologies to reduce emissions are challenging to implement on a practical scale, and economic instruments are likely to play a role in future energy systems by encouraging adoption of these technologies. Carbon trading is an emerging economic instrument that enables entities (plants, governments, etc.) to exchange emission rights, allowing economic and environmental aspects to be balanced. This study introduces a scalable carbon trading modelling approach, integrated into previously developed DECO2 open-source energy planning framework. Direct and indirect optimisation approaches are proposed, both consisting of superstructure-based mixed-integer nonlinear programming formulations. Carbon price is a variable in the direct optimisation or a parameter in the indirect optimisation approach. While the direct optimisation approach involves more non-linearity, it is shown to result in solutions with greater decarbonisation, higher profits, and lower costs, compared to the indirect optimisation results. A novel feature of this multi-period model not considered in previous works is the simultaneous emissions trading across time periods and among entities (power plants and government). This enables efficient and coordinated emission allowances trading among various entities and timeframes. Various new costs and revenue streams are added into the energy planning framework; therefore, profits can also be predicted, along with predictions of electricity prices. New energy resources (nuclear and wind) and carbon capture utilisation and storage are also introduced to the modified DECO2 model. The models are tested on the Pakistan’s power sector. Minimisation of emissions using direct optimisation showed that the carbon trading increased profits significantly in the second, third, and fourth planning periods (4.74, 3.86, and 3.55 times, respectively), but in the first period, profits were slightly higher without carbon trading (1.06 times more). Minimisation of budget using indirect optimisation showed higher profits in case of no carbon trading for all the periods. Between 2021 and 2040, hydropower is expected to grow the most (by a minimum of 3.14 times and a maximum of 15.87 times), followed by solar (with an expected increase between 2.54 and 3.26 times) and wind generation (which may increase by 2.35 to 2.66 times). Deployment of emission reduction technologies is significantly lower when carbon trading is implemented as compared to when it is not, due to increased pressure on CO2-intensive generation. Results show that incorporating carbon trading into an energy market leads to both financial (increased profits) and environmental (lower emissions) sustainability, and that using direct optimisation approach increases benefits of carbon markets.
A new method for the detailed design of shell and tube heat exchangers is presented through the formulation of coupled differential heat equations, along with algebraic equations for design variables. Heat exchanger design components (tube passes, baffles, and shells) are used to discretize the differential equations and are solved simultaneously with the algebraic design equations. The coupled differential algebraic equation (DAE) system is suitable for numerical optimization as it replaces the nonsmooth log mean temperature difference (LMTD) term. Discrete decisions regarding the number of shells, fluid allocation, tube sizes, and number of baffles are determined by solving an LMTD‐based method iteratively. The resulting heat exchanger topology is then used to discretize the detailed DAE model, which is solved as a nonlinear programming model to obtain the detailed exchanger design by minimizing an economic objective function through varying the tube length. The DAE model also provides the stream temperature profiles inside the exchanger simultaneously with the detailed design. It is observed that the DAE model results are almost equal to the LMTD‐based design model for one‐shell heat exchangers with constant stream properties but shows significant differences when streams properties are allowed to vary with temperature or the number of shells are increased. The accuracy of the solutions and the required computational costs show that the model is well suited for solving heat exchanger network synthesis problems combined with detailed exchanger designs, which is demonstrated in Part 2 of the paper.
Sugarcane bagasse (SCB) is an agro-industrial residue extracted during sugar processing. Sugar mills use basic process technologies for bagasse valorisation. Residues are often disposed improperly or burned inefficiently by thermal boilers causing environmental pollution. Biorefining bagasse as substrate for bio-based chemical production is superior to biomass disposal by incineration, burning and landfill. The conversion of bagasse for value-added applications may be economical with less environmental impact on humans and the ecosystem. Computer aided tools such as ontologies for knowledge modelling represent available information for bagasse feedstocks. This paper presents a reference model referred to as the SCB Ontology which is limited to a framework for the modelling of knowledge on the current utilisation of SCB feedstocks from principal sugarcane-cultivating countries. The SCB Ontology identifies opportunities for efficient bagasse valorisation by principal sugarcane producers. Potential application of the SCB Ontology for bagasse valorisation which is a circular bioeconomy initiative was also discussed.
In a highly competitive retail market, many microbreweries have attempted to maximise profits and decrease energy consumption through retrofitting their operations with renewable energy. This paper develops an optimisation model to minimise investment and operation costs of a microbrewery meeting the dynamic energy demands, via an integrated photovoltaic (PV) system with energy storage and different boiler choices to lower carbon emissions. A microbrewery in UK has been used for the case study to demonstrate the approach on real data, with challenges in implementation and real-world constraints and considerations discussed. A set of rigorous multi-objective optimisation and sensitivity analyses are performed to analyse the resulting system. For the particular brewery, a modern electric boiler combined with photovoltaic system is an economic and sustainable choice, due to the cooling and other electric requirements in the brewery, leading to a 33 percent reduction in operational costs with a payback time of 2.6 years.
Marginal abatement cost curves are widely used as an effective means of visualizing trade-offs between emissions reduction and cost. They involve plotting multiple mitigation options on rectangular coordinates, with cumulative abatement as the horizontal axis and specific abatement cost as the vertical axis. The options are arranged in order of ascending specific cost such that, for any given marginal cost threshold, the corresponding cumulative abatement can be readily determined and easily communicated to decision-makers. This approach is very effective for decision support and can potentially be applied to constraints other than cost. In this paper, an extension of this technique is developed for decarbonization measures while considering secondary environmental footprints instead of cost. A case study on six terrestrial Negative Emissions Technologies (NETs) using global sequestration potentials is used to show how trade-offs between climate change mitigation and land or water footprints can be visualized using these Marginal Abatement Footprint (MAF) curves. The results show that targeting an annual negative emission of 8.8 Gt CO2/y requires a MAF of at least 58 Mha/Gt CO2 for land, and at least 1.3 × 10-5 Gt/Gt CO2 for water. Based on the MAF thresholds applied in the study, water is more limiting than land.
In the past two decades, various process integration methods have been proposed for the optimum synthesis of resource conservation networks, for the recovery of energy and material resources such as water, gas, and solvent. In this paper, a mathematical programming framework is proposed for simultaneous optimization of mass exchanger networks (MENs) and direct reuse/recycle networks (DRNs). Both MEN and DRN synthesis are now relatively established fields with numerous methods developed. Note however that there is a lack of work that considers both areas simultaneously. The newly proposed simultaneous optimization method in this work identifies opportunity for a DRN that allows the material waste to be recycled within the MEN, which is the main novelty of this work. The approach is demonstrated with a vinyl acetate monomer production problem. The latter consists of several mass exchange operations, in which an MEN is synthesized. Opportunity to develop an DRN is also identified, which allows its waste to be recycled without regeneration. Another novelty of the work is that, the supply and target compositions in the MEN problem are expressed in terms of mass ratios as the compositions are relatively large. Results show that the integrated network has a total annualized cost that is reduced by 24.9% as compared to the base case configuration, where both networks were solved independently. This shows the importance of considering entire process systems during process synthesis, as opposed to subsystems independently.
This study develops an optimal integrated energy network for 15 decentralised rural neighbourhood structures (sectors and clients) that are isolated from the main energy grid in Tsumkwe, Namibia. The structures have different demand profiles dictated by the type of building, the time of day, as well as the season. Because of the logistical difficulty and cost implication associated with bringing fuel (such as diesel) from distant suppliers to remote locations, the isolated communities need to make optimum use of the locally available biomass resources and have effective planning of outsourced fuel in their supply chain (SC). Biomass resources considered in this study are animal dung, fuel wood, human waste, and crop residue. These biomass sources were coupled with photovoltaic (PV) units and lithium storage batteries. The optimal network showed PV-SC selection with minimal battery storage selection. The SC shows a favourable cost advantage over the energy storage batteries and can support most of the intermittency of the PV supply. The SC contributed 94.1 % and 42.7 % with regard to the total cost and energy supply to demand structures. The PV panel was the preferred energy supply technology for the Distributed Energy System (DES) because of its cost effectiveness (57.2 % and 5.94 % energy and cost contribution to the network). The total annual power supply of the minigrid is 0.465 GWh/y. The total annual cost (TAC) of the minigrid was found to be N$ 2,580,000. The minigrid power production cost was found to be 39 % more expensive than energy from the National grid tariff. However, it does not require long-range transmission and makes provision for local job opportunities.
The synthesis of heat exchanger networks (HENs) has received significant attention in the last four decades. On the other hand, mass exchanger networks (MENs), whose synthesis methods have been based on analogies drawn from HEN synthesis, has received relatively less attention. This paper presents a new synthesis method for interplant MENs synthesis by drawing analogies from the interplant synthesis methods developed for its HENs counterpart. The approach adopted in this paper entails the utility hub concept where the plants that are participating in the integrated network are connected through external mass separating agents located at the utility hub. The integrated network of this paper is modelled using a modified version of the stage-wise superstructure synthesis method for MENs. The newly developed method is applied to a case study involving two plants and one utility hub. The solution obtained involves two mass exchangers in plant 1, two mass exchangers in plant 2 and four mass exchangers at the utility hub. A 21 % reduction in cost was obtained when the same external mass separating agent is used to absorb multiple components from rich streams at the utility hub when compared to the case where different external mass separating agents are used.
Biomass resources have the potential to become a viable renewable technology and play a key role within the future renewable energy paradigm. Since CO2 generated in bio-energy production is equal to the CO2 absorbed during the growth of the biomass, this renewable energy is a net zero emissions resource. Biomass gasification is a versatile method for transforming waste into energy in which biomass material is thermochemically converted within a reactor. Gasification's superior flexibility, including both in terms of biomass type and heat generation or energy production alternatives, is what stimulates biomass gasification scientific and industrial potential. Downdraft gasifiers seem to be well for small-scale generation of heat along with energy, whereas fluidised bed and entrained flow gasifiers currently attain significant economies of scale for fuel production. The operation of gasifiers is influenced by several factors, including operational parameters, feedstock types, and reactor design. Modelling is a valuable tool for building a unit based on the results of model prediction with different operational parameters and feedstock in such scenarios. Once verified, a suitable model may be used to assess the sensitivity of a gasifier's performance to changes in various operational and design factors. Effective models may help designers to theorise and predict the impacts of a variety of characteristics without the need for further empirical observations, which can help in the design and implementation of this technology. This work provides an overview of gasification technologies and a succinct guidance to the modelling decisions and modelling strategies for biomass gasification to enable a successful biomass to fuel conversion. A technical description and critical analysis of thermodynamic, stoichiometric, computational fluid dynamic and data-driven approaches is provided, including crucial modelling considerations that have not been explored in earlier studies. The review aims to aid researchers in the field to select the appropriate approach and guide future work.
•Detailed heat exchanger models are embedded within a heat exchanger network synthesis optimization.•A trust region filter algorithm is used as a surrogate modelling strategy for HENS for the first time to incorporate pressure drops, numbers of shells, etc.•An integer-cut strategy algorithm is introduced to explore different topologies.•Results are compared to existing methods for solving HENS with detailed models, demonstrating excellent performance. We develop a trust region filter strategy for simultaneous optimal design of heat exchanger networks that includes detailed design of shell-and-tube heat exchangers. The strategy first solves a mixed-integer nonlinear programming (MINLP) formulation with shortcut models to generate candidate network topologies, which are then used in a non-isothermal mixing nonlinear programming (NLP) suboptimization with detailed optimal exchanger design models embedded using a modified trust region filter (TRF) algorithm. An integer cut based strategy is used to bound the solutions from MINLP and the NLP which aids in convergence to the solution of the overall simultaneous design problem. Under assumptions, the TRF based strategy can guarantee convergence to near optimal solutions of the overall design problem. The presented solution strategy is thus able to find optimal heat exchanger network designs based on the simultaneous optimization of the network topology and mass and energy balances, together with detailed shell-and-tube heat exchanger optimization, including the number of shell and tube passes, pressure drops, and tubes, tube lengths, etc. The proposed strategy is tested on three literature based case studies and their results are compared with previous studies to showcase its performance.
Optimisation models for the design of distributed energy systems (DES) often exclude inherent nonlinearities and constraints associated with alternating current (AC) power flow and the underlying distribution network. This study aims to assess this gap by comparing the performance of linear and nonlinear formulations of DES design models, connected to and trading with an AC grid. The inclusion of the optimal power flow (OPF) constraints within the DES design framework is demonstrated in the methodology. A residential case study is used to test both models and compare the designs obtained from the two formulations. The results highlight that DES designs obtained are different when constraints related to the underlying distribution network are added, particularly when electricity storage is not considered. Overall, this study highlights the need for future modelling efforts to include OPF within DES optimisation frameworks to obtain practically feasible designs, rather than considering them as standalone problems.
The optimal selection, sizing, and location of small-scale technologies within a grid-connected distributed energy system (DES) can contribute to reducing carbon emissions, consumer costs, and network imbalances. There is a significant lack of studies on how DES designs, especially those with electrified heating systems, impact unbalanced low-voltage distribution networks to which most DES are connected. This is the first study to present an optimisation framework for obtaining discrete technology sizing and selection for grid-connected DES design, while simultaneously considering multiphase optimal power flow (MOPF) constraints to accurately represent unbalanced low-voltage distribution networks. An algorithm is developed to solve the resulting Mixed-Integer Nonlinear Programming (MINLP) formulation. It employs a decomposition based on Mixed-Integer Linear Programming (MILP) and Nonlinear Programming (NLP) and uses integer cuts and complementarity reformulations to obtain discrete designs that are also feasible with respect to the network constraints. A heuristic modification to the original algorithm is also proposed to improve computational speed. Improved formulations for selecting feasible combinations of air source heat pumps (ASHPs) and hot water storage tanks are also presented. Two networks of varying size are used to test the optimisation methods. Designs with electrified heating (ASHPs and tanks) are compared to those with conventional gas boilers. The algorithms outperform one of the existing state-of-the-art commercial deterministic MINLP solvers, which fails to find any solutions in two instances within specified time limits. While feasible solutions were obtained for all cases, convergence was not achieved for all, especially for those involving the larger network. Where converged, the algorithm with the heuristic modification has achieved results up to 70% faster than the original algorithm. Results for case studies suggest that including ASHPs can support up to 16% higher renewable generation capacity compared to gas boilers, albeit with higher ASHP investment costs, as local generation and consumption minimises network violations associated with excess power export. The results also show the importance of including nonlinear power flow constraints in DES design problems. The optimisation framework and results can be used to inform stakeholders such as policymakers and network operators, to increase renewable energy capacity and aid the decarbonisation of domestic heating systems. •The selection of discrete technologies in the presence of MOPF constraints is enabled.•An improved formulation selects feasible ASHP - thermal storage combinations.•The algorithm finds feasible solutions where the state-of-the-art MINLP solver fails.•The proposed heuristic modification decreases computational time.•ASHPs with local renewable energy generation can help minimise network violations.
These datasets are generated using a modified version of the DECO2 software and contain detailed optimization results for Pakistan's power sector, which includes 208 power plants, over the planning period of 2021-2040. The '1 - Min_Emission_CT_OFF.xlsx' file provides the optimized results for emissions minimization without carbon trading, while the '2 - Min_Emission_CT_ON_Direct_Optimization.xlsx' file contains the results for emissions minimization with carbon trading. Similarly, the '1 - Min_Budget_CT_OFF.xlsx' file lists the optimized results for budget minimization without carbon trading, while the '2 - Min_Budget_CT_ON_Indirect_Optimization.xlsx' file contains the results for budget minimization with carbon trading.
The deployment of CO2 capture and storage (CCS) and negative emissions technologies (NETs) are crucial to meeting the net-zero emissions target by the year 2050, as emphasised by the Glasgow Climate Pact. Over the years, several energy planning models have been developed to address the temporal aspects of carbon management. However, limited works have incorporated CCS and NETs for bottom-up energy planning at the individual plant scale, which is considered in this work. The novel formulation is implemented in an open-source energy system software that has been developed in this work for optimal decarbonisation planning. The DECarbonation Options Optimisation (DECO2) software considers multiperiod energy planning with a superstructural model and was developed in Python with an integrated user interface in Microsoft Excel. The software application is demonstrated with two scenarios that differ in terms of the availabilities of mitigation technologies. For the more conservative Scenario 1, in which CCS is only available in later years, and other NETs are assumed not to be available, all coal plants were replaced with biomass. Meanwhile, only 38% of natural gas plants are CCS retrofitted. The remaining natural gas plants are replaced with biogas. For the more aggressive Scenario 2, which includes all mitigation technologies, once again, all coal plants undergo fuel substitution. However, close to half of the natural gas plants are CCS retrofitted. The results demonstrated the potential of fuel substitutions for low-carbon alternatives in existing coal and natural gas power plants. Additionally, once NETs are mature and are available for commercial deployment, their deployment is crucial in aiding CO2 removal in minimal investment costs scenarios. However, the results indicate that the deployment of energy-producing NETs (EP-NETs), e.g., biochar and biomass with CCS, are far more beneficial in CO2 removal versus energy-consuming NETs (EC-NETs), e.g., enhanced weathering. The newly developed open-source software demonstrates the importance of determining the optimal deployment of mitigation technologies in meeting climate change targets for each period, as well as driving the achievement of net-zero emissions by mid-century.
This chapter presents a synthesis method for multi‐period combined heat and mass exchange networks (CHAMENs). The mass exchange network involves a regeneration network (REN) superstructure with multiple recyclable mass‐separating agents (MSAs) and multiple regenerating streams. The CHAMENs superstructure with REN is integrated with utilities generated from both renewable and fossil‐based energy sources. Hence, the environmental impact of such networks is studied. Lastly, the proposed CHAMEN model is extended to handle multi‐objective optimization (MOO) of both environmental impact and economics, to identify an optimum network configuration.
Mass exchanger networks (MENs) are used to remove/recover contaminants from polluted streams through absorption with available process streams or external mass separating agents. Process Integration techniques such as Pinch Technology (PT) or mathematical optimisation can be used to synthesise optimal networks, however a lack of accessible software and difficulties in formulating the non-convex problem has stunted research. This article presents an open-source Python package for the synthesis of optimal MENs. The package uses the algebraic modelling language, Pyomo, and takes advantage of Python’s object-oriented nature to solve a series of optimisation problems, improving on the performance of previous approaches to the problem of incorporating detailed unit designs into MEN synthesis. The package uses automated initialisation strategies to first solve a superstructure-based mixed-integer nonlinear program (MINLP). Thereafter, a detailed optimisation model, formulating the packed column as a system of differential-algebraic equations, is used to design the columns. This detailed packed column design is used to update the MINLP through correction factors, driving the network solution towards the detailed unit optimisation solutions. The new software, called MExNetS, implements this strategy in a user-friendly package that is easily modified and well-documented. In addition to the new software implementation, novel strategies are employed to ensure feasibility at each iteration, which is a challenge in these non-convex optimisation formulations, and new binary cuts are generated and applied to the MINLP that can significantly speed up convergence compared to the previous study. The package also contains automatic superstructure generation based on user-inputted data, with the hope that this software can inspire further research in this area and be accessible to practitioners.
Developing a flexible heat exchanger network, that will remain operable in the face of potential variations in stream parameters, or variables, around some nominal values, especially for large problems, are difficult to solve simultaneously. A hybrid synthesis approach that systematically combines sequential and mathematical programming techniques may be more suitable to adopt. The proposed method presented in this paper entails firstly generating two representative single period networks using the Pinch Technology Stream Temperature versus Enthalpy Plot (STEP) approach and the multi-period stage-wise superstructure model for heat exchanger network synthesis. Streams for the representative network are obtained using the largest stream heat demand as criteria. The second stage of the proposed method entails generating a reduced multi-period stage-wise superstructure, using a combination of the matches obtained in the single period representative networks of the first step as initialising matches. The solution of the reduced superstructure of the second step, which is solved as a Mixed Integer Non-Linear Programming (MINLP) model, is then selected as the best multi-period network. The newly developed method of this paper is tested using an example from the literature. One of the solutions obtained is only 0.9 % higher, in terms of total annual cost, than the best solution presented in the literature but has the benefit of a fewer number of units.
Objectives: Experimental inversion of circadian and behavioural rhythms by 12-hours adversely effects markers of metabolic health. The objective of this work was to investigate the effects of a more modest 5-hour delay in behavioural cycles (as observed in e.g. trans-Atlantic or trans-continental jetlag) on circadian and metabolic markers. Methods: Fourteen participants completed an 8-day inpatient laboratory protocol, with controlled sleep-wake opportunities, light-dark cycles, meal timing and diet composition. The 5-hour delay in sleep/wake and mealtimes was induced by delaying sleep opportunity. We measured melatonin to assess central circadian phase; markers of postprandial metabolism; subjective sleepiness, and appetite. Results: Melatonin rhythms gradually adapted to the new behavioural cycle. In the 4-hours after the phase delay, there was slower gastric emptying at breakfast, lower fasting plasma glucose, higher postprandial plasma glucose and triglycerides, and lower thermic effect of feeding (p < 0.05). These changes were abolished or attenuated within 48–72 hours of the phase delay. Conclusions: Moderate (5-hour) circadian desynchrony only transiently disrupts metabolism. Occasional jetlag may therefore pose low metabolic health risk
This work presents a new method for integrating various renewable energy feedstock sources with the utility systems of combined heat and power generation hubs and heat exchanger networks (HENs). The combined heat and power hub of the integrated network involves two turbines fed with high pressure steam. The steam can be generated from fuel sources such as wood, corn stover, and glycerine. The power system is required to produce a fixed amount of shaft power while optimally satisfying the hot utility demand of the HEN of a process plant through the high-pressure steam and intermediate pressure steams exiting the turbines. The superstructure of the integrated system comprises three layers and is synthesised using the utility hub approach. The first layer of the superstructure, which comprises the feedstock supply chain network, is modelled as a mixed integer linear program. The second layer of the superstructure, which comprises the combined heat and power hub wherein are the steam system and turbines for the power plant, is modelled using linear program to represent the material and energy balances of the turbine system. The third layer, which comprises a HEN, is modelled using the simplified stagewise superstructure (SWS) synthesis approach. The objective function of the integrated model comprises operating costs, capital costs, and environmental impact. The newly developed method is applied to a case study using the weighted method of multi-objective optimisation and the results obtained involves the selection of corn stover and glycerine for the generation of heat and power. Also, only high-pressure steam and medium pressure steam were selected for use as hot utilities in the integrated HEN of the system.
Multiperiod carbon-constrained energy planning considers long-term demand variations as well as progressively stringent CO2 emission limits towards net-zero carbon goals. Previous work on multiperiod energy planning has predominantly focused on the power generation sector. Nevertheless, emissions originating from industries must be mitigated to achieve decarbonisation at different (e.g., corporate, national, or regional) scales. This work develops a multiperiod energy planning model to determine the optimum deployment of energy sources for CO2-intensive industries other than the power generation sector. A hybrid approach is employed in this work, making use of the combined automated targeting model (ATM) and superstructure models. The hybrid approach in this work overcomes the non-linearity in the CO2 intensity calculations. Solving the ATM and superstructure model simultaneously ensures that both demand and CO2 emission limits for all industries are satisfied in each period. The multiperiod energy planning model is demonstrated with a case study with and without CO2 load transfer scenarios. The case study is performed for 21 industries across three 5-year periods. For the CO2 load transfer scenarios, the CO2 emission limits may be violated in earlier periods, triggering CO2 debts. The latter are compensated by CO2 credits in later periods where the total CO2 load is below the emissions limit due to aggressive mitigation strategies. Alternatively, in the absence of CO2 load transfer, the CO2 emission limits for all periods must be satisfied. Results show that the deployment of low-carbon energy sources (e.g., biomass and biogas) is necessary for the satisfaction of the CO2 emission limits within each period. Once CO2capture and storage (CCS) is available, its deployment would complement existing mitigation strategies in later periods. The deployment of CCS on power plants fueled by biomass and biogas make them as negative emissions technologies (NETs) which are useful for CO2 removal (CDR). The development of the multiperiod energy planning model in this work allows decarbonisation strategies to be simultaneously employed across all industries for a cumulative CO2 emissions reduction.
The optimal design of grid-connected distributed energy systems (DES) has been previously investigated, but most studies exclude nonlinear multiphase optimal power flow (MOPF) constraints which capture imbalances in alternating current (AC) distribution networks. Previous DES design studies considering AC power flow assume balanced loads and networks, which is an assumption that does not hold for distribution networks that are often inherently unbalanced. This is the first study to present an optimisation framework for designing grid-connected DES while simultaneously considering multiphase optimal power flow (MOPF) constraints to accurately represent unbalanced low-voltage distribution. This study proposes a new algorithm for obtaining DES designs subject to nonlinear multiphase power flow constraints with regularised complementarity reformulations for operational constraints containing binary variables. DES design models with either MOPF or OPF are tested using an unbalanced distribution network, where rooftop solar, gas boilers, and battery storage are considered as distributed resources. Despite the increased complexity, DES design with MOPF obtains the best solution, with 81% greater solar capacity and 6% lower total annualised cost when compared to DES with OPF. Designs from a commonly used linear DES design framework based on direct current (DC) approximations produce the highest costs (8% more than initially predicted) when tested with MOPF. The proposed algorithm with complementarity reformulations achieves a 19% improvement in the objective value over a previous two-stage decomposition for DES with OPF, where the entire binary topology in the nonlinear model is fixed. The study therefore enables the acquisition of DES designs that can work symbiotically within unbalanced distribution networks.
The decarbonisation of residential heating systems has become increasingly important to meet the global goals of minimising carbon emissions and combating climate change. However, with rising energy costs, this can be a significant challenge for low-income households. This study presents a novel optimisation framework to aid the decarbonisation of residential heating in the United Kingdom by combining technology-related decision-support with policy decisions. The framework can recommend the optimal retrofit of low-carbon heating technologies and fabric improvement measures such as insulation upgrades for improving energy efficiency. Concurrently, the optimal financial contributions towards investment costs from grants supporting low-income households and social housing is determined. It also includes piecewise linearisations to capture the detailed operation of air source heat pumps, which are set to replace natural gas-based heating systems, and assesses the eligibility of each dwelling for grant funding. A large case study consisting of social housing stock in Woking, UK, has been used to test the framework. Three scenarios are used to assess the efficacy of existing technology and policy combinations to meet local emissions reduction targets, which are benchmarked against emissions from existing gas-based heating systems and insulation measures. Results highlight the limitations of existing UK grants, as these can only achieve an emissions reduction of 33.5% without incurring significant additional investment costs to the local council. The lack of support towards installing hot water tanks, which are required for the operation of heat pumps, is another major limitation in existing grants. A proposed scenario, which introduces a fictional grant with unlimited funding, sheds light on the much larger grant contributions expected to achieve an emissions reduction of 66.8%, which surpasses local targets. These results also suggest the need for operational support to cope with much higher energy bills, especially for low-income and/or fuel-poor households, due to the electrification of heating systems. Overall, the framework is a useful tool for local councils, policy makers, and other stakeholders to make informed decisions on the affordable decarbonisation of residential heating systems.
In many developing nations, the carbon intensity of the grid is high while capacity is also constrained. Local distributed energy resources are expensive to deploy and own by private households. This work presents an optimisation-based approach to optimally locate renewable technologies at both local prosumer’s dwellings and at community-level locations, incorporating the addition of distribution lines in a distributed energy system (DES) model to implement a solar photovoltaic (PV) energy farm with energy storage at the community level in densely populated urban areas. A case study of three scenarios in Karachi, Pakistan is selected to test the model. Scenario 1 presents a decentralised approach where every node has the possibility to install batteries locally. Scenario 2 considers a more centralised approach where only 2 central battery nodes are connected to different residential nodes. Lastly, the scalability of the model is tested with Scenario 3 which considers a model with 4 times as many nodes. The optimisation model indicates the profitability of different centralised/decentralised approaches with the highest level of decentralisation showing both the lowest cost and highest profit made, demonstrating the trade-off between battery sizing and line costs for privatised local energy storage.
Aquaculture is a growing industry that provides high-quality protein, however, the growth of aquaculture production has a range of environmental concerns. Recirculating aquaculture systems (RAS) are a popular solution to accomplish intensifying fish production, and integrating renewable technologies is an attractive option that may provide low-carbon energy and promote sustainability. This paper presents an optimisation model for the control and operation of a grid-connected distributed energy system (DES) integrated with an RAS. A linear optimal power flow (OPF) combined with model predictive control (MPC) strategy is developed which simulates the thermal and electricity balances present throughout the renewable energy integrated electricity network and RAS, to make real-time optimal operation scheduling plans on different time horizons. The optimal results show that the MPC strategy improves the control performance of system operation and improves the process economics. With renewable energy sources (RES), operational costs can be reduced by up to 27%. Compared with a conventional scheduling plan, the rolling horizon approach can provide 3% energy cost saving, while maintaining fish well-being and system safety.
Multi-period Heat Exchanger Networks (HENs) are designed as heat recovery energy efficient systems over a set of operating conditions for process streams. The problem becomes more complex when detailed exchanger designs are accounted for in the network synthesis problem. Typically, in mixed-integer nonlinear programming (MINLP) multi-period HEN optimisation, the maximum area heat exchanger across all periods is considered. However, when considering detailed designs, often this exchanger is unsuitable for operation over all periods. In this study, a trust-region algorithm is proposed to incorporate detailed exchanger designs for multi-period operation. The exchanger design is modelled using surrogate models inside a network-level NLP model which is derived from the multi-period MINLP HENS model solution. The method is applied to a case study and the results show the effectiveness of the proposed algorithm.
To the editor – The Glasgow Agreement statement on the " phase-down " of coal means that limiting warming to well below 2C will require more ambitious emissions cuts through other means. To achieve mid-century carbon neutrality, aggressive policies are needed to reduce point-source emissions primarily through measures such as increased use of renewables. Since many developing nations will be unable to make this transition by 2050, negative emissions technologies (NETs) will also have to be scaled up rapidly to offset residual emissions from fossil fuels through carbon dioxide removal (CDR) 1,2. Many start-ups have followed the trend of the emerging "drawdown economy" to commercialize NETs through the sale of credits from CDR, but there remains a gap in providing top-down decision support for their strategic deployment considering local and regional constraints. The ramp-up of CDR to the gigaton scale has serious implications for energy systems due to the electricity requirement of many NET alternatives 3. Timing is also critical; recent modelling results focusing on bioenergy with carbon capture and storage and direct air capture show that early deployment can reduce cumulative costs of achieving net-zero emissions in the European Union 4. Exploring diverse NETs portfolios is critical due to their potential to avoid risks in large-scale deployment 5. For example, vast tracts of monoculture plantations for bioenergy with carbon capture and storage (BECCS) can threaten biodiversity 6. No single option can sustainably meet the climate targets, so multiple NETs at moderate scales are needed 7. However, most research papers still focus on individual NETs, with few taking a system-level outlook. The global and regional potential capacities of NETs have been evaluated individually rather than as part of a carbon management portfolio 8,9. Integrated assessment modelling (IAM) studies have focused mainly on BECCS and afforestation and reforestation (AR) analyzed separately 10. Only one IAM study to date has attempted the optimization of a NETs portfolio consisting of BECCS, direct air carbon capture and storage (DACCS), enhanced weathering, and AR 11. The results of the study show that a full portfolio supports the attainment of net-zero faster and with a better balance of regional CDR contributions. Instead of assuming a predefined set of NETs for decarbonization, portfolio optimization approaches will be essential for enabling rapid scale-up to meet net-zero targets (Fig. 1). New models are urgently required to determine the optimal mix of NETs to maximize CDR within cumulative cost, natural resource, and sustainability limits while accounting for the trade of carbon credits. Process integration techniques originally developed to improve the energy and resource efficiency of industrial plants can be adapted for this purpose 12. In the previous decades, various mathematical models and algorithms from process integration have been extended to industrial decarbonization through the optimum allocation of renewable energy resources and optimal planning of CO2 capture and storage 13. Other variants have also been developed to optimize research and development portfolios of low-carbon technologies 14. Such techniques have only been recently developed for the optimal deployment of NETs considering impacts on background energy systems 15 as well as cost and environmental footprints 16. These early works demonstrate the promising potential of this class of models, but much more research remains to be done so they can be used to accelerate the scale-up of NETs in the coming decades.
Mass and heat integration are important to achieving economically and environmentally sustainable processes through increased efficiency. Typically, heat and mass exchange networks are solved separately using process integration techniques such as pinch technology or formulating nonconvex mixed-integer nonlinear programming (MINLP) problems, which are challenging to solve. To simplify the MINLP, shortcut models are employed, which can result in under/overestimation of the real network, leading to suboptimal or infeasible designs. We introduce a new optimisation algorithm for combined heat and mass exchanger network synthesis (CHAMENS), including detailed design models. The method uses shortcut models in an MINLP to find network topology, followed by a nonlinear programming (NLP) suboptimisation. The NLP allows non-isothermal and non-isocompositional mixing, uses detailed unit models of packed columns based on orthogonal collocation on finite elements (OCFE), and detailed shell and tube heat exchanger designs. We incorporate a differential-algebraic equation (DAE) based shell and tube heat exchanger design model via surrogates in a trust region filter (TRF) framework, guaranteeing optimal solutions for the detailed exchanger models are found by the surrogate models. We demonstrate the proposed approach on a case study, showcasing its performance and the need to incorporate detailed unit models in topology optimisation to find practical optimal designs.
This work provides the first systematic critical review of mass exchanger network synthesis literature. Mass exchanger networks play a central role in many pollution reduction and resource utilisation processes and contain many complex decisions to be made including exchanger types, sizing, and mass separating agent selection. We present a comprehensive review of the key milestones in the development of methods for mass exchanger network synthesis and focus on the key challenges that have hindered research in this area from flourishing in the manner of the conceptually similar heat exchanger network synthesis problem. We find that several important research questions remain for the methods to find wider use in industry. More efficient techniques for solving nonconvex mixed-integer nonlinear programs and better methods of including more accurate, higher-order unit models for industrial problems within network optimisation problems are particularly important, as current methods provide highly simplified unit representations that do not take into account many important practical design considerations that have significant cost implications. Furthermore, we identify significant potential for further research into increasing the scope of the problem to include issues such as flexibility and controllability, inter-plant mass exchanger networks, batch processes, retrofit and further integration of heat and mass exchanger networks, with research into these domains limited. Through further research of these under-developed applications of mass exchanger network synthesis, we envision that techniques for mass integration can become a powerful tool to enhance mass integration techniques for sustainable cleaner production technology. •Existing synthesis methods have been mainly graphical and deterministic.•More robust methods should be developed for multicomponent systems.•Most methods have included simplified unit designs rather than detailed ones.•Controllability and flexibility despite being crucial have received less attention.•Opportunities for interplant mass integration should be explored.
A trust region framework is presented to synthesize heat exchanger network with detailed exchanger designs. The heat exchanger network (HEN) is first synthesized using the stage wise superstructure (SWS) formulation of Yee and Grossmann (1990). After a topology is found in this step the heat exchangers and the connections, flows and intermediate temperatures are designed, using the first principles based differential algebraic (DAE) model presented in our previous work. These detailed DAEs for heat exchanger design are incorporated within a nonlinear programming (NLP) model using reduced order models and solved using NLP solver IPOPT with a trust region method. The results show that the new method is faster than the previous approaches while providing comparable results.
The year 2020 has seen the emergence of a global pandemic as a result of the disease COVID-19. This report reviews knowledge of the transmission of COVID-19 indoors, examines the evidence for mitigating measures, and considers the implications for wintertime with a focus on ventilation.
Residential heating displays huge decarbonisation potential towards Net-Zero. The complexity of heating system and socio-economic system appeals for a systematic design to avoid exacerbating fuel poverty. This study develops a three-layer heat-for-all model which integrates building stocks analysis, distributed heating system optimisation, economic and environmental impacts simulation to tackle heating decarbonisation and fuel poverty simultaneously. This whole system model is a powerful decision support tool that can help conceive heating decarbonisation strategies for wider regions and countries. More than 400,000 scenarios are created, considering the effects of future policy schemes (No Grant, Business as Usual, Proposed), minimum emission reduction target, carbon intensity of grid, future natural gas, and electricity prices. Results show that optimised heating system decarbonisation plan heavily relies on future energy prices. In the case study, only air source heat pumps are chosen when electricity price is lower than 3 times gas price. Secondly, investment in heating system could stimulate the greenhouse gas emission of whole supply chain, hedging the emission reduction achieved in heating system. This further reveals that life cycle thinking is imperative in GHG emission mitigation. Thirdly, electricity decarbonisation plays a vital role in achieving whole system emission reduction. The grid carbon intensity reduction makes substantial contribution to the emission reduction of heating system and industry system. In tackling fuel poverty, it's worth noticing that the fuel poverty is aggravated with more grant support under certain scenarios, since current policy schemes focus on capital investment in heating system but overlook the increased energy bills. It appeals for a more comprehensive policy design considering all stakeholders. [Display omitted] •A three-layer whole system approach to tackle heating decarbonisation and fuel poverty.•Optimised heating system decarbonisation plan heavily relies on future energy prices.•Investment in heating system stimulates the greenhouse gas emissions of whole supply chain.•Electricity decarbonisation plays a pivotal role in whole system emission reduction.•Fuel poverty will be exacerbated without better policy design.
We propose a new strategy to synthesize heat exchanger networks with detailed designs of individual heat exchangers. The proposed strategy uses a multistep approach by first obtaining a heat exchanger network topology through solving a modified version of the mixed integer nonlinear programming (MINLP) stage‐wise superstructure of Yee and Grossmann, which includes a smoothed LMTD approximation and pressure drops. In a second nonlinear programming (NLP) suboptimization step, we allow for nonisothermal mixing to solve problems with or without exchanger bypasses. The selected heat exchangers along with the mass and energy balances obtained are then used to design the network with detailed exchanger designs through solving a sequence of NLPs for individual heat exchanger designs. The NLPs are based on the detailed discretized optimization models of Kazi et al., which solve quickly and reliably to obtain heat exchangers based on rigorous, first‐principles derived coupled differential equations. These models solve a differential algebraic equation system and do not rely on usual assumptions associated with other heuristic‐based exchanger design methods, such as log mean temperature difference and FT correction factors. These detailed exchanger designs are then used to update the network optimization model through sets of correction factors on heat exchanger area, number of shells, heat transfer coefficients, and pressure drops of each exchanger design, in a method based on that of Short et al. The method solves reliably, guaranteeing feasible exchangers for every potential network generated by the shortcut models, through validation with rigorous heat exchanger models at every iteration. In addition, the method does not increase the nonlinearity of the MINLP model, nor does it require any manual intervention or initialization from the user. Three examples are solved and the results are compared to those obtained in the literature.
The flexibility of gasification, which incorporates both characteristics of the kind of biomass and options for producing heat or fuel, is what stimulates interest in biomass gasification research and application possibilities. One of the challenges with gasification is the accurate prediction of gasification products from the wide array of feedstocks and operational conditions, making the design and optimisation challenging. There are several studies on equilibrium models that have been published, but most of the models examined the impact of moisture and heating value. Examining the other parameter effects within the same model can enhance the accuracy of a gasification model. This work aims to create stoichiometric equilibrium models that enable the analysis of parameter effects on a specific feedstock's gasification products for use in optimisation and system modelling. A mathematical model for fixed bed gasifiers that use downdraft gasification for wood as a feedstock is presented. When the algebraic model equations are solved, the conversion behaviour of wood biomass throughout a gasification process is predicted in terms of the composition of the end products. The model has been verified with published experimental data from the literature demonstrating good agreement. The created model may be regarded as a helpful tool to simulate the impact of numerous different biomass feedstocks and operating conditions on gas characteristics and the simple algebraic formulation allows for the application of the model for optimisation purposes.
Optimisation and simulation models for the design and operation of grid-connected distributed energy systems (DES) often exclude the inherent nonlinearities related to power flow and generation and storage units, to maintain an accuracy-complexity balance. Such models may provide sub-optimal or even infeasible designs and dispatch schedules. In DES, optimal power flow (OPF) is often misrepresented and treated as a standalone problem. OPF consists of highly nonlinear and nonconvex constraints related to the underlying alternating current (AC) distribution network. This aspect of the optimisation problem has often been overlooked by researchers in the process systems and optimisation area. In this review we address the disparity between OPF and DES models, highlighting the importance of including elements of OPF in DES design and operational models to ensure that the design and operation of microgrids meet the requirements of the underlying electrical grid. By analysing foundational models for both DES and OPF, we identify detailed technical power flow constraints that have been typically represented using oversimplified linear approximations in DES models. We also identify a subset of models, labelled DES-OPF, which include these detailed constraints and use innovative optimisation approaches to solve them. Results of these studies suggest that achieving feasible solutions with high-fidelity models is more important than achieving globally optimal solutions using less-detailed DES models. Recommendations for future work include the need for more comparisons between high-fidelity models and models with linear approximations, and the use of simulation tools to validate high-fidelity DES-OPF models. The review is aimed at a multidisciplinary audience of researchers and stakeholders who are interested in modelling DES to support the development of more robust and accurate optimisation models for the future.
Increasing deployment of renewable energy resources for power generation has been playing a pivotal role in reducing carbon emissions associated with electrical power systems. Distributed Energy Systems (DES) enable the integration of small-scale renewable energy resources and storage technologies within low-voltage (LV) distribution networks, which supply power to residential and commercial consumers. Care must be taken when designing these systems, as they could potentially impair the operation and infrastructure of existing power networks. While nonlinear balanced optimal power flow formulations have historically been incorporated into oversimplified Mixed-Integer Linear Programming (MILP) DES design models, these do not accurately model the distribution networks to which most DES are connected. Low-voltage radial distribution networks are most closely represented by nonconvex multi-phase formulations, which are computationally complex and difficult to solve. The exclusion of these constraints within DES design models could, however, lead to infeasible designs, i.e., designs which are incompatible with the existing network and its operations. This study proposes a novel optimisation algorithm, capable of solving the large-scale and combined problem of designing DES with multiphase optimal power flow. A large-scale Nonlinear Programming (NLP) model with full power flow constraints and reformulated complementarity constraints for DES operation is used to find a feasible upper bound, if the lower bound proposed by the MILP for DES design is infeasible. The algorithm is tested using a residential case study based on a section of the IEEE EU LV network. Results for this case study show that the proposed algorithm finds a feasible DES design and operational schedule by installing three times the battery capacity initially recommended by the MILP. The MILP design remains infeasible with respect to the multiphase power flow constraints. This framework could be used to support the increase of local renewable energy generation and consumption, and the subsequent reduction of carbon intensity in existing power networks.
Negative emissions technologies (NETs) will be needed to reach net-zero emissions by mid-century. However, NETs can have wide-ranging effects on land and water availability, food production, and biodiversity. The deployment of NETs will also depend on regional and national circumstances, technology availability, and decarbonization strategies. Process integration (PI) can be the basis for decision support models for the selection, planning, and optimization of the large-scale implementation of NETs. This paper reviews the literature and maps the role of PI in NETs deployment. Techniques such as mathematical programming, pinch analysis (PA), process graphs (P-graphs), are powerful methods for planning NET systems under resource or footprint constraints. Other methods such as multi-criteria decision analysis (MCDA), marginal abatement cost curves, causality maps, and machine learning (ML) are also discussed. Current literature focuses mainly on bioenergy with carbon capture and storage (BECCS) and afforestation/reforestation (AR), but other NETs need to be integrated into future models for large-scale decarbonization.
Net-zero emission targets are being set by countries and companies globally. To meet net-zero targets, negative emission technologies (NETs) such as bioenergy with carbon capture and storage (BECCS) and biochar (BC) can be implemented alongside replacing traditional energy sources with renewables. Various high-level tools for energy planning are available but rarely consider NETs and net-zero emissions planning. In this work, a graphical procedure was developed using marginal abatement cost (MAC) curves for net-zero emissions planning. Carbon dioxide (CO2)-emitting energy sources are arranged in increasing order of specific costs, on a specific cost vs cumulative CO2 emissions diagram. NETs are then plotted to counterbalance the emissions in order to determine the negative emission targets. The methodology is illustrated with a case study in the power generation sector. In this case, coal and natural gas (500 MW each) are used to generate power for users. In order to reach net-zero emission for this sector, a BECCS plant (500 MW) and a reduction in the power rating of coal by around 200 MW is necessary. Besides, an additional 1.59 Mt/y of CO2 sequestration is to be carried out through BC. The proposed technique provides a simple and clear method for net-zero emissions planning.
Multivariate spectroscopic data is increasingly abundant in the chemical and pharmaceutical industries. However, it is often challenging to estimate reaction kinetics directly from it. Recent advances in obtaining kinetic parameter estimates from spectroscopic data based on large-scale nonlinear programming (NLP), maximum likelihood principles, and discretization on finite elements lead to increased speed and efficiency (Chen et al., 2016). These new techniques have great potential for widespread use in parameter estimation. However they are currently limited due to their applicability to relatively small problem sizes. In this work, we extend the open-source package for estimating reaction kinetics directly from spectra or concentration data, KIPET, for use with multiple experimental datasets, or multisets (Schenk et al., 2020). Through a detailed initialization scheme and by taking advantage of large-scale nonlinear programming techniques and problem structure, we are able to solve large problems obtained from multiple experiments, simultaneously. The enhanced KIPET package can solve problems wherein multiple experiments contain different reactants and kinetic models, different dataset sizes with shared or unshared individual species’ spectra, and can obtain confidence intervals quickly based on the NLP sensitivities. In addition, we propose a new variance estimation technique based on maximum likelihood derivations for unknown covariances from two sample populations. This new variance estimation technique is compared to the previously proposed iterative-heuristics-based algorithm of Chen et al. (2016) for distinguishing between variances of the noise in model variables and in the spectral measurements. We demonstrate the new techniques on a variety of example problems, with sample code, to show the utility of the approach and its ease of use. We also include the curve-fitting problem to cases where we have concentration data given directly, and are required to estimate kinetic parameters across multiple experimental datasets.
This paper presents a systematic synthesis method that considers multiple shells and logarithmic mean temperature difference (LMTD) FT correction factor for heat exchanger networks (HENs) involving multiple periods of operation. The approach adopted entails firstly generating a reduced multiperiod HEN superstructure using network solutions obtained when the STEP (Stream Temperature Versus Enthalpy Plot) and HEAT (Heat Allocation and Targeting) synthesis methods are applied to each subperiod. The second stage entails generating an initial multiperiod HEN solution from the reduced superstructure synthesis approach. The number of shells, as well as the FT correction factor, required by each exchanger in each period of the initial multiperiod HEN are then manually calculated and used to initialise the multiperiod HEN to obtain updated representative heat exchanger areas for all stream pairs in all periods of operations. The solution obtained, when the method of this paper is applied to a literature example, shows that the assumption of 1 – 1 (1 shell pass – 1 tube pass) design configuration for multiperiod HEN problems, underestimates the representative heat exchanger areas by 12.3%.
The design and operation of distributed energy systems (DES) have often been modelled as linear optimisation problems. Although DES are increasingly connected to existing alternating current (AC) distribution networks, state-of-the-art DES modelling frameworks use oversimplified approximations which either exclude network constraints or overlook the inherent three-phase unbalance present in distribution networks. This can lead to poor designs which amplify network operational issues and result in greater costs to both the network and consumers/producers. This study presents a new modelling framework for DES design, which incorporates unbalanced optimal power flow within DES models for the first time. Furthermore, Robust Optimisation is included in this detailed modelling framework to ensure design feasibility under worst-case scenarios. Results show that previous frameworks tend to either overestimate or underestimate objectives when compared with the DES model combined with unbalanced power flow. Robust scenarios demonstrate that the new combined model is capable of closing the gap between objectives when compared with a linear DES-only model, albeit with different designs that do not violate grid constraints during baseline operation. These results suggest that this detailed framework can be utilised for DES design and network planning, as it produces more robust designs which can potentially help avert operational issues.
Many autonomous microgrids have extensive penetration of distributed generation (DG). Optimal power flow (OPF) is necessary for the optimal dispatch of networked microgrids (NMGs). Existing convex relaxation methods for three-phase OPF are limited to radial networks. In light of this, we develop a semidefinite programming (SDP) convex relaxation model which can cope with meshed networks and also includes a model of three-phase DG and under-load voltage regulators with different connection types. The SDP model solves the OPF problem of multi-phase meshed network effectively, with satisfactory accuracy, as validated by real 6-bus, 9-bus, and 30-bus NMGs, and the IEEE 123-bus test cases. In the SDP model, the convex symmetric component of the three-phase DG model is demonstrated to be more accurate than a three-phase DG modelled as three single-phase DG units in three-phase unbalanced OPF. The proposed method also has higher accuracy than the existing convex relaxation methods. The resultant optimal control variables obtained from the convex relaxation optimization can be used for both final optimal dispatch strategy and initial value of the non-convex OPF to obtain the globally optimal solution efficiently.
This paper presents KIPET (Kinetic Parameter Estimation Toolkit) an open-source toolbox for the determination of kinetic parameters from a variety of experimental datasets including spectra and concentrations. KIPET seeks to overcome limitations of standard parameter estimation packages by applying a unified optimization framework based on maximum likelihood principles and large-scale nonlinear programming strategies for solving estimation problems that involve systems of nonlinear differential algebraic equations (DAEs). The software is based on recent advances proposed by Chen et al. (2016) and puts their original framework into an accessible framework for practitioners and academics. The software package includes tools for data preprocessing, estimability analysis, and determination of parameter confidence levels for a variety of problem types. In addition KIPET introduces informative wavelength selection to improve the lack of fit. All these features have been implemented in Python with the algebraic modeling package Pyomo. KIPET exploits the flexibility of Pyomo to formulate and discretize the dynamic optimization problems that arise in the parameter estimation algorithms. The solution of the optimization problems is obtained with the nonlinear solver IPOPT and confidence intervals are obtained through the use of either sIPOPT or a newly developed tool, k_aug. The capabilities as well as ease of use of KIPET are demonstrated with a number of examples.
Additional publications
Short, M., Isafiade, A.J., Biegler, L.T., Kravanja, Z., 2018, Synthesis of mass exchanger networks
in a two-step hybrid optimization strategy, Chemical Engineering Science, 178, 118-135.
Isafiade, A.J., Short, M., Bogataj, M., Kravanja, Z., 2017, Integrating Renewables into Multi-
Period Heat Exchanger Network Synthesis Considering Economics and Environmental Impact,
Computers & Chemical Engineering , 99, 51-65.
Short, M., Isafiade, A.J., Fraser, D.M., Kravanja, Z., 2016, Two-step hybrid approach for the
synthesis of multi-period heat exchanger networks with detailed exchanger design, Applied
Thermal Engineering, 105, 807-821.
Isafiade, A.J., Short, M., 2016, Simultaneous synthesis of flexible heat exchanger exchange
networks for unequal multi-period operations, Process Safety and Environmental Protection ,
103, 377-390.
Short, M., Isafiade, A.J., Fraser, D.M., Kravanja, Z., 2016, Synthesis of heat exchanger networks
using mathematical programming and heuristics in a two-step optimisation procedure with
detailed exchanger design, Chemical Engineering Science, 144, 372-385.
Crimes, J., Isafiade, A.J., Fraser, D.M., Short, M., Bonomi, A., 2016, Assessment of pre-treatment
technologies for bioethanol production from sugarcane bagasse considering economics and
environmental impacts, Asia-Pacific Journal of Chemical Engineering , 12 (2), 212–229.
Fraser, D.M., Short, M., Crimes, J., Azeez, O.S., Isafiade, A.J., 2016, A systematic comparison of
stagewise/interval-based superstructure approaches for the optimal synthesis of heat
exchange networks, Chemical Engineering Transactions , 52, 793-798.
Isafiade, A.J., Short, M., 2016, Synthesis of mass exchange networks for single and multiple
periods of operations considering detailed cost functions and column performance, Process
Safety and Environmental Protection , 103, 391-404.
Isafiade, A.J., Short, M., 2016, Multi-period heat exchanger network synthesis involving multiple
sources of utilities and environmental impact, Computer-Aided Chemical Engineering, 38,
2067-2072.
Short, M., Isafiade, A.J., Fraser, D.M., Kravanja, Z., 2015, Heat exchanger network synthesis
including detailed exchanger designs using mathematical programming and heuristics,
Chemical Engineering Transactions , 45, 1849-1854.
Isafiade, A.J., Jegede, K., Cele, S., Crimes, J., Short, M., Wan Alwi, S.R., 2015, Synthesis of multiperiod
multiple utilities heat exchanger networks considering economics and environmental
impact, APCCHE 2015, 942.