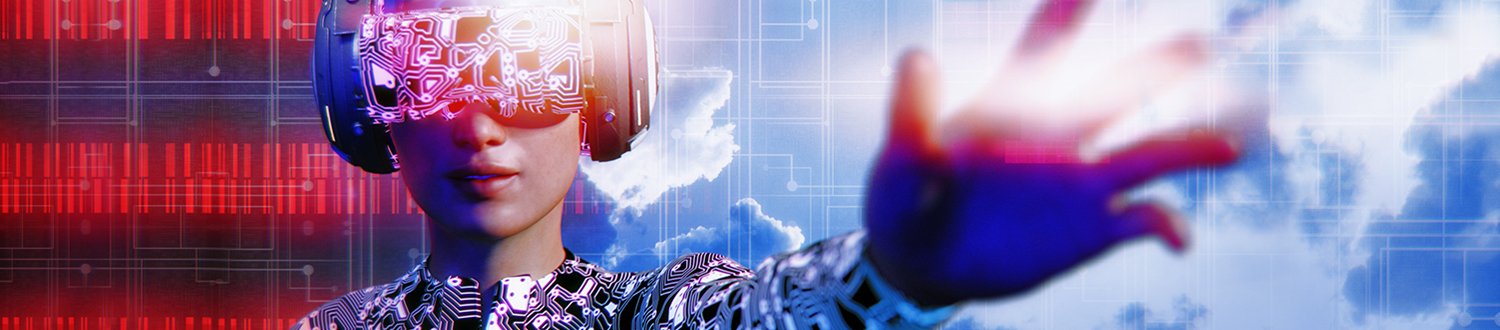
Postgraduate research students
Find out more about our postgraduate research student projects.
Postgraduate research students
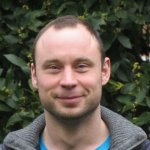
Ian Ackerley
Postgraduate Research Student
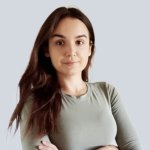
George Alcolado Nuthall
Postgraduate Research Student
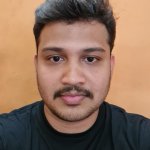
Tony Alex
Postgraduate Research Student
Joan Manuel Amaya Cuesta
Viral Zoonoses Research Scientist
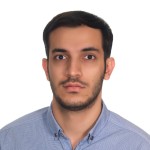
Sobhan Asasi
Postgraduate Research Student
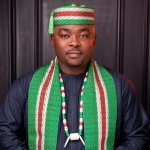
Daniel Ayuba
Postgraduate Research Student
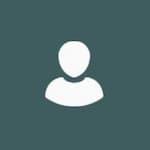
Isaac Baglin
Postgraduate Research Student
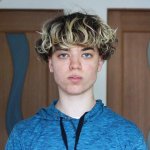
Kar Balan
Postgraduate Research Student
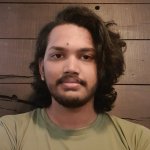
Hmrishav Bandyopadhyay
Postgraduate Research Student
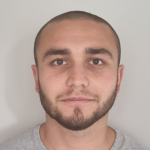
Charlie Baskerville
Postgraduate Research Student
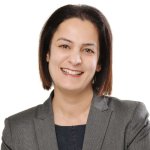
Nivedita Bijlani
Postgraduate Research Student
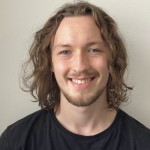
Claude Boucher
Postgraduate research student
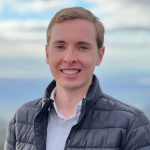
David Butler
Postgraduate Research Student
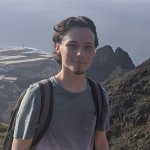
Ollie Camilleri
Postgraduate Research Student
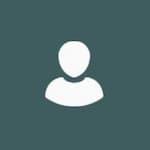
Gemma Canet Tarres
Postgraduate research student
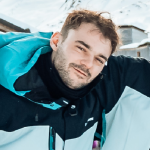
Ben Canini
Postgraduate Research Student
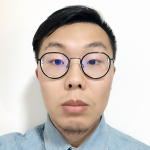
Haojie Chang
Postgraduate Research Student
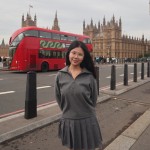
Dr Yuelan Cheng
Postgraduate research student
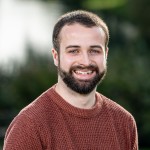
Oliver Cory
Postgraduate Researcher in Deep Learning for Sign Language Production
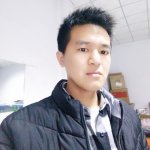
Meng Cui
Postgraduate Research Student
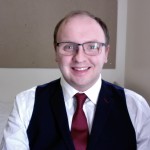
Jake Cumber
Postgraduate Research Student & Research Assistant
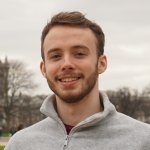
Adam Dowse
Postgraduate Research Student
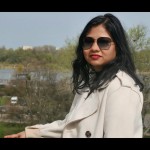
Ayushi Dutta
Postgraduate research student
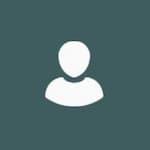
Umar Farooq
Postgraduate Research Student
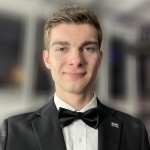
Rogier Fransen
Postgraduate Research Student
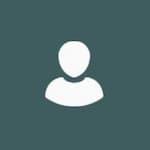
Alejandro Hernandez Diaz
PhD Researcher in Computer Vision
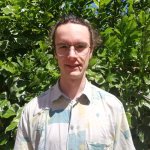
Jack Hilliard
Postgraduate Research Student
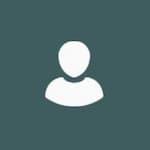
Maxim Ivashechkin
Postgraduate Research Student
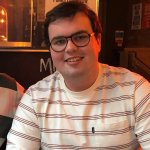
Robert John
Postgraduate Research Student
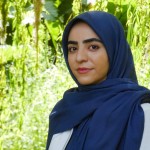
Elaheh Kalantari
Postgraduate Research Student
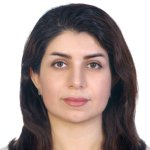
Bahar Khorram
Postgraduate Research Student
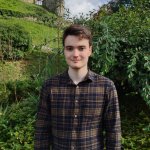
James King
Postgraduate Research Student
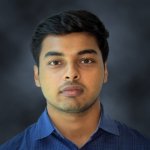
Subhadeep Koley
Postgraduate Research Student in Computer Vision & Deep Learning
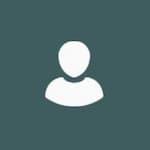
Yash Kulthe
Postgraduate Research Student
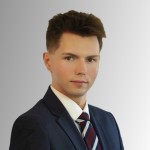
Nick Leybourne
Postgraduate Research Student
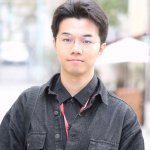
Haohe Liu
Postgraduate Research Student
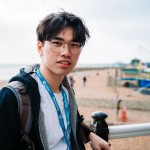
Jian He Low
Postgraduate Researcher in Computer Vision and Deep Learning
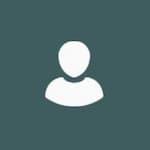
Layi Macaulay
Postgraduate research student
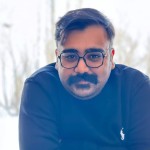
Milad Masroor
PG/R - Comp Sci & Elec Eng
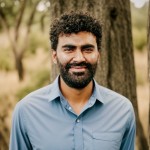
Asmar Nadeem
Postgraduate Research Student/ Research Fellow in Multimodal AI
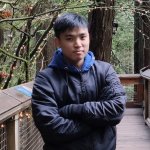
Kam Woh Ng
Postgraduate Research Student
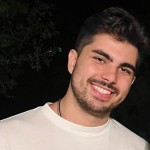
Alp Bora Orgun
Postgraduate research student
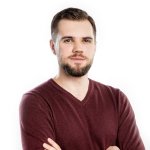
Anton Pelykh
Postgraduate Research Student
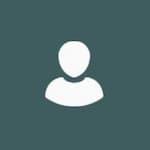
Zhiyu Qu
Postgraduate Research Student
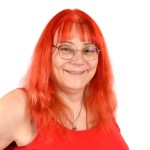
Dawn Raison
Postgraduate Research Student
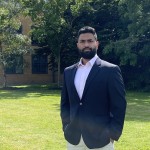
Taimoor Rizwan
Postgraduate Research Student
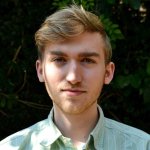
Tav Shore
Postgraduate Research Student in AI and Computer Vision
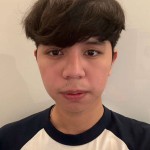
Wish Suharitdamrong
Postgraduate Research Student
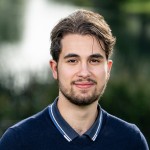
Alexandre Symeonidis-Herzig
Postgraduate Research Student
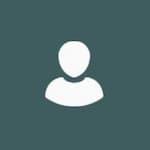
Will Tapper
Postgraduate Research Student
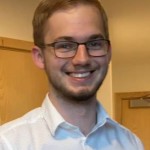
Marshall Thomas
Postgraduate Research Student
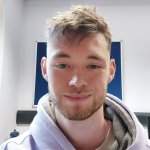
Billy Vale
Postgraduate Researcher in AI and Medical Imaging
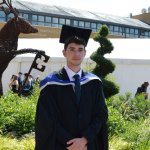
Harry Walsh
Postgraduate Research Student
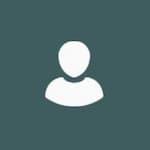
Dongyu Wang
Postgraduate Research Student
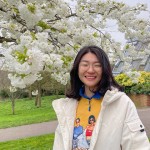
Wenqing Wang
Postgraduate Research Student
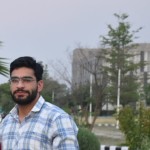
Mohd Ubaid Wani
Postgraduate Research Student
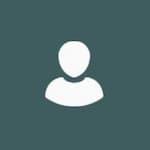
Ryan Wong
Postgraduate Research Student
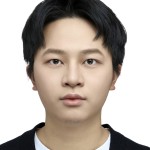
Yang Xiang
Postgraduate Research Student
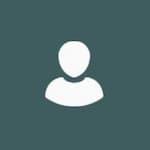
Yanze Xu
Postgraduate Research Student
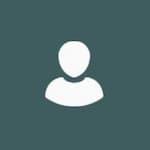
Sam Yap
Postgraduate research student
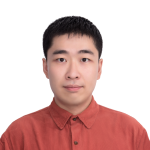
Yi Yuan
Postgraduate Research Student
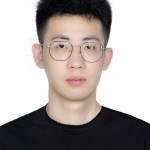
Zheng Zhang
Postgraduate Research Student
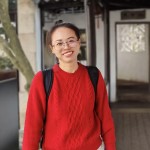
Peng Zhang
Deep learning for audio-visual scene analysis
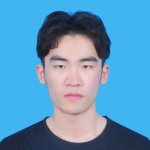
Jinzheng Zhao
Postgraduate research student
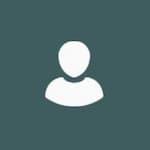
Junqi Zhao
Postgraduate Research Student
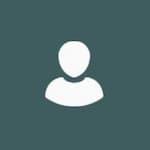
Özkan Çayli
Postgraduate Research Student