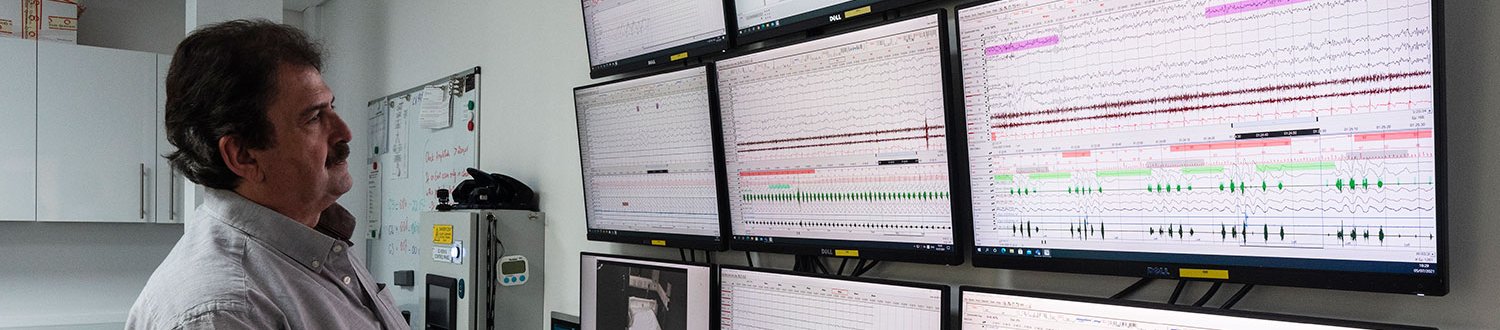
Digital health technology and artificial intelligence
The capacity to unobtrusively monitor sleep and circadian physiology over long periods of time and at home, is essential for understanding the contribution of sleep to health. Digital health technology and artificial intelligence offer new opportunities to detect changes in sleep that may serve as an early warning signal for deterioration of health.
Overview
We are testing a wide range of digital health technologies and novel data analysis approaches.
Selected publications
Mohammadi SM, Enshaeifar S, Hilton A, Dijk DJ, Wells K. Transfer Learning for Clinical Sleep Pose Detection Using a Single 2D IR Camera. IEEE Trans Neural Syst Rehabil Eng. 2021;29:290-299. doi: 10.1109/TNSRE.2020.3048121. Epub 2021 Mar 1. PMID: 33378261.
Mikkelsen KB, Ebajemito JK, Bonmati-Carrion MA, Santhi N, Revell VL, Atzori G, Della Monica C, Debener S, Dijk DJ, Sterr A, de Vos M. Machine-learning-derived sleep-wake staging from around-the-ear electroencephalogram outperforms manual scoring and actigraphy. J Sleep Res. 2019 Apr;28(2):e12786. doi: 10.1111/jsr.12786. Epub 2018 Nov 13. PMID: 30421469; PMCID: PMC6446944.
Skeldon AC, Dijk DJ, Meyer N, Wulff K. Extracting Circadian and Sleep Parameters from Longitudinal Data in Schizophrenia for the Design of Pragmatic Light Interventions. Schizophr Bull. 2021 Nov 10:sbab124. doi: 10.1093/schbul/sbab124. Epub ahead of print. PMID: 34757401.