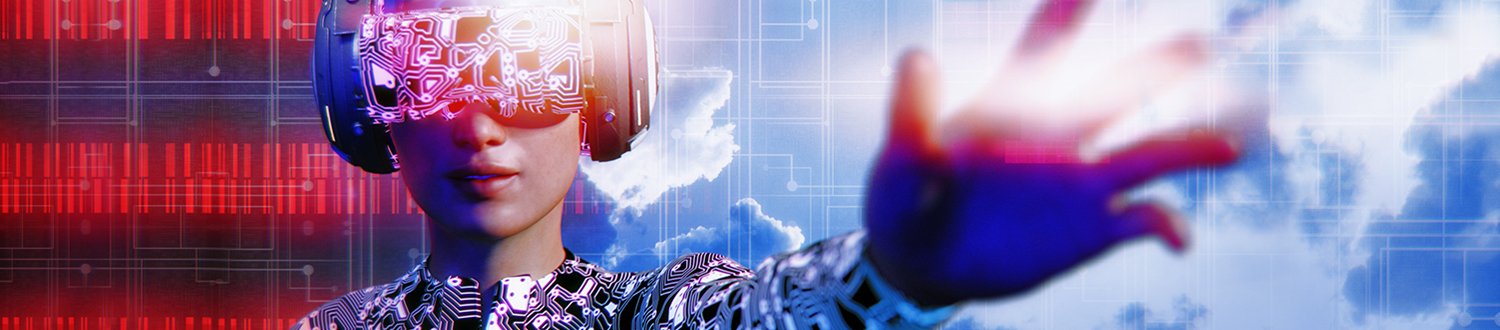
Academic staff
Find out more about our academic staff.
Academic staff
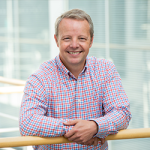
Professor Adrian Hilton
Director Surrey Institute for People-Centred AI | Director of Centre for Vision, Speech and Signal Processing
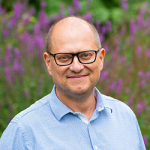
Professor Miroslaw Bober
Professor of Video Processing
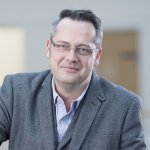
Professor Richard Bowden
Professor of Computer Vision and Machine Learning
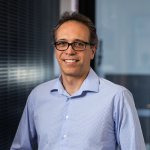
Professor Gustavo Carneiro
Professor of AI and Machine Learning
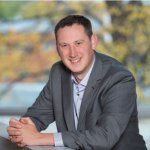
Professor John Collomosse
Professor of Computer Vision and AI | Director DECaDE: UKRI/EPSRC Centre for the Decentralised Digital Economy
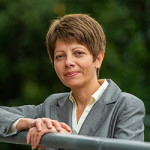
Dr Lucia Florescu
Lecturer in Medical Imaging
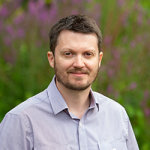
Dr Jean-Yves Guillemaut
Senior Lecturer in 3D Computer Vision
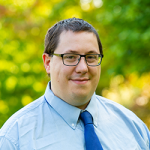
Dr Simon Hadfield
Associate Professor (Reader) in Robot Vision and Autonomous Systems
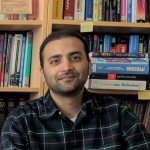
Dr Syed Sameed Husain
Lecturer in People-Centred AI
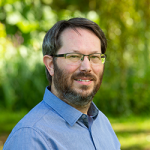
Professor Philip Jackson
Professor in Machine Audition
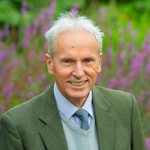
Professor Josef Kittler
Distinguished Professor
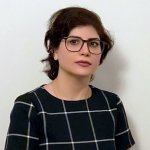
Dr Samaneh Kouchaki
Senior Lecturer in Machine Learning for Healthcare
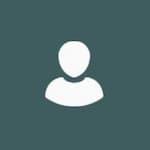
Professor Jiri Matas
Vice Chancellor Fellow
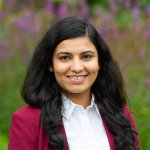
Dr Armin Mustafa
Associate Professor in Computer Vision and AI
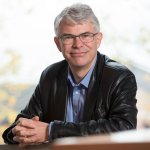
Professor Mark Plumbley
Professor of Signal Processing, EPSRC Fellow in "AI for Sound"
Dr Muhammad Rana
Trustworthy and Responsible AI
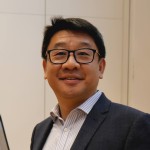
Professor Yi-Zhe Song
Co-Director Surrey Institute for People-Centred AI; Programme Lead of MSc in Artificial Intelligence
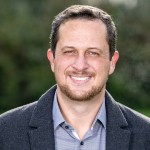
Dr Erick Sperandio Nascimento
Associate Professor (Reader) in AI for Clean Air and Programme Lead of AI and Sustainability
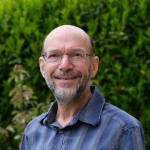
Dr Graham Thomas
Professor of Creative Technology
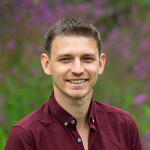
Dr Marco Volino
Lecturer in Computer Vision and Graphics
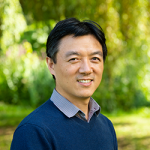
Professor Wenwu Wang
Professor in Signal Processing and Machine Learning; Associate Head (External Engagement), School of Computer Science and Electronic Engineering
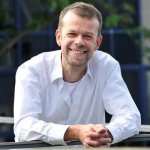
Professor Kevin Wells
Professor of AI in Human and Veterinary Healthcare
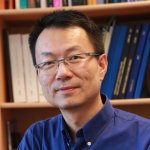
Professor Tao Xiang
Professor of Computer Vision and Machine Learning
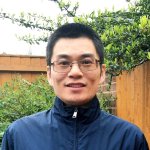
Dr Xiatian Zhu
Senior Lecturer in People-Centred AI
Affiliate academic staff
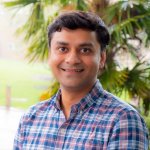
Dr Anjan Dutta
Senior Lecturer in Artificial Intelligence (AI)