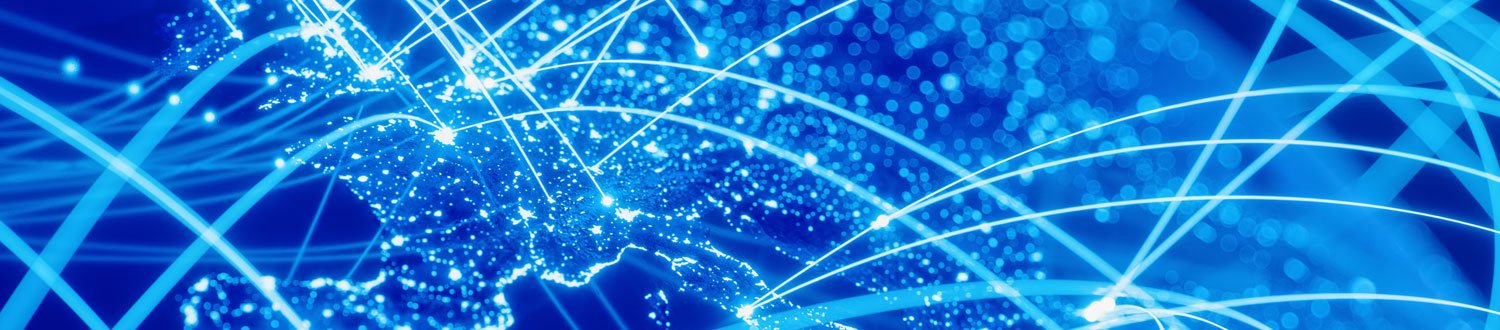
Research staff
Get in contact with our research staff.
Research staff
Computer science staff
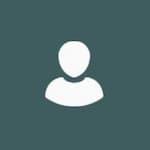
Dr Sharar Ahmadi
Research Fellow
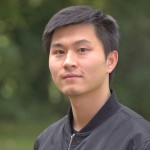
Xiongjie Chen
Postdoctoral Researcher
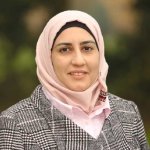
Dr Nada El Kassem
Research Fellow in Trusted Computing and Applied Cryptography
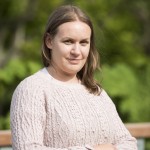
Dr Ashley Fraser
Research Fellow in the DECaDE centre for the Decentralised Digital Economy
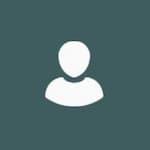
Mohamed Kassem
Research Fellow
Dr Vinod Khandkar
Research Fellow in Adaptive Online Safety and Privacy
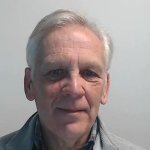
Dr Christopher Newton
Research Fellow
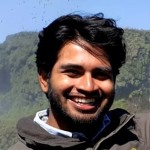
Dr Chandresh Pravin
Postdoctoral Research Fellow
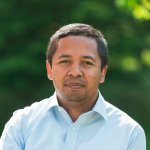
Dr Solofomampionona Fortunat Rajaona
Research Fellow in Secure Systems
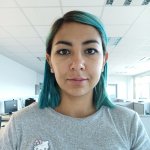
Dr Karla Vargas
Research Fellow in Distributed Computing
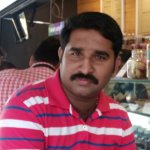
Dr Dany Varghese
Research Fellow
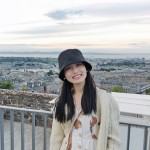
Dr Xilu Wang
Surrey Future Fellow
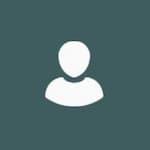
Dr Rafik Zitouni
Research Fellow B | Lead Software Engineer
Electronic engineering staff
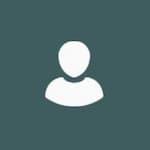
Dr Alireza Ahrabian
Research Fellow
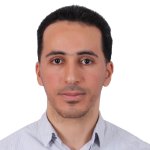
Dr Ahmed Almradi
Research Fellow
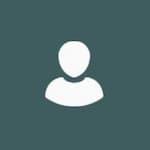
Dr Majdi Alnowami
Research Fellow
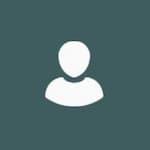
Matthew Barker
Researcher in Space Radiation Environment and Effects
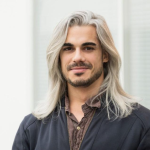
Gabriel Bibbo
Research Fellow in Sound Sensing
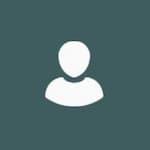
Dr Francois Carrez
Senior Research Fellow
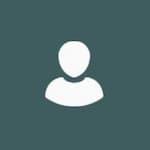
Dr Chi Ho Chan
Research Fellow
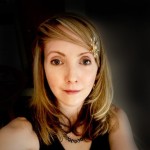
Dr Emily Corrigan-Kavanagh
Surrey Future Fellow in Designing AI for Home Wellbeing, Surrey AI Fellow
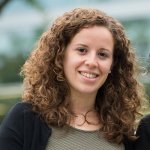
Dr Catia Costa
Research Fellow
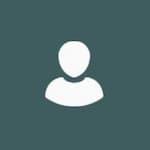
Christopher Davis
Research Fellow in Space Weather and Atmospheric Radiation Modelling
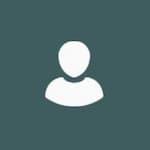
Dr Juan Carlos De Luna Ducoing
Research Fellow
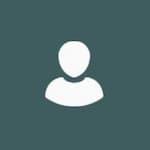
Dr Farshad Einabadi
Senior Research Engineer
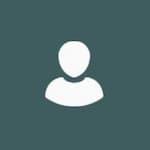
Dr Fariborz Entezami
5G Test Bed Manager
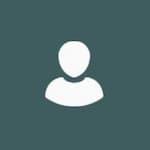
Jason Forshaw
Research Fellow II
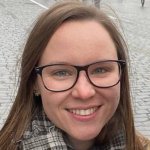
Dr Yulia Gryaditskaya
Lecturer in People Centred Artificial Intelligence
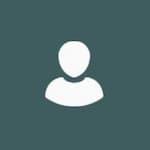
Dr Alexander Hands
Research Assistant
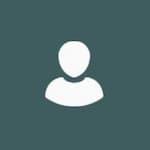
Dr Seyed Hoseinitabatabaei
Senior Research Fellow
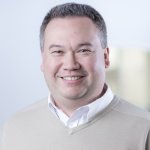
Bernard Hunt
5G Programme Manager
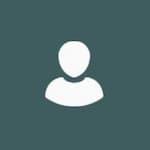
Dr Yasin Isfahani
Research Fellow
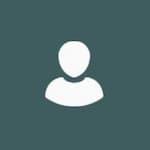
Dr Burak Karadag
Research Fellow
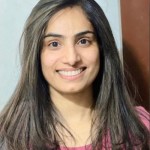
Dr Amrit Kaur
Research Fellow in Electronic Systems
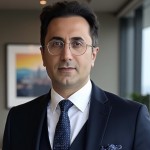
Dr Mohsen Khalily
Associate Professor in Antenna and Propagation
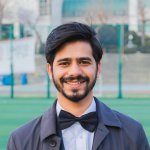
Dr Fasihullah Khan
Research Fellow
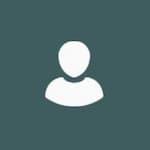
Dr Satish Kumar
Research Fellow
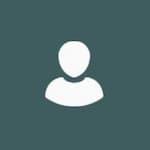
Dr Alexandr Kuzminskiy
Senior Research Fellow
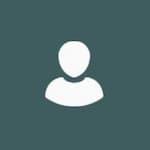
Dr Mohamed Lakhal
Research Fellow
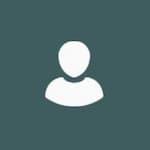
Ao Lei
Research Fellow in Communication Network Security
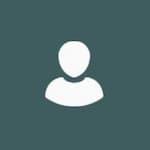
Giulio Luzzati
Research Staff
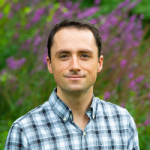
Dr Charles Malleson
Research Fellow in Computer Vision, CVSSP
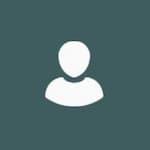
Chunxu Mao
Senior Research Fellow
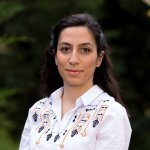
Dr Ozge Mercanoglu Sincan
Research Fellow in Computer Vision and Deep Learning
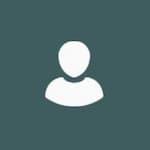
Dr Karim Nasr
Research Fellow
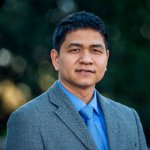
Dr Hung Nguyen
Research Staff
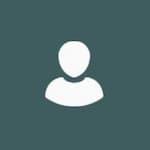
Dr Colin O'Reilly
Research Fellow
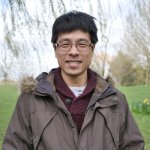
Dr Eng-Jon Ong
Research Fellow
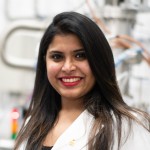
Dr Hashini Perera
Research Fellow in Organic Optoelectronics
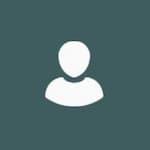
Dr Haris bin Pervaiz
Research Fellow
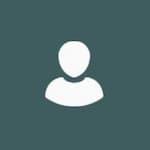
Dr Craig Pitcher
Research Fellow
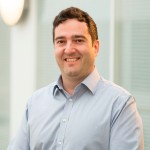
Dr Riccardo Pozza
Chief Network Architect
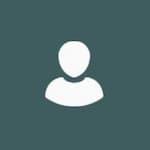
Matthew Price
Research Staff
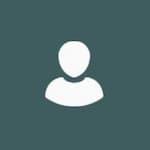
Dr Peng Qian
Research Fellow
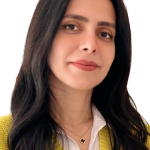
Dr Zahra Rahimian Omam
Research Fellow
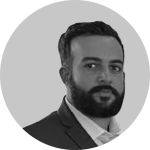
Dr Taran Rai
Data Scientist
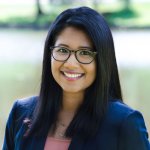
Dr Indrachapa Bandara Rajapakshe Mudiyanselage
Research Fellow in Organic Electronics
Dr Muhammad Rana
Senior Lecturer in Trustworthy and Responsible AI. Leaading the research on foundation models and self-supervised learning
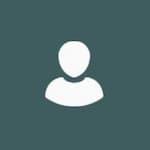
Dr Ruth Rawcliffe
Research fellow
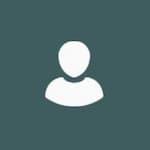
Dr Maria Ribera Vicent
Research Fellow
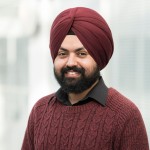
Dr Arshdeep Singh
UKAN+ Early Career Acoustic Champion, Research Fellow A in AI4S Project + Sustainability Fellow at the Institute for Sustainability
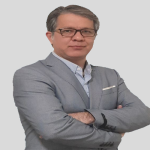
Dr Seyed Ahmad Soleymani
Research Fellow
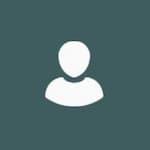
Dr Dionysia Triantafyllopoulou
Senior Research Fellow
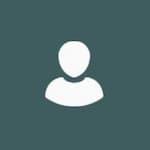
Dr Seiamak Vahid
Senior Research Fellow
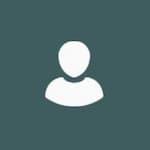
Yong Xia
Research Fellow
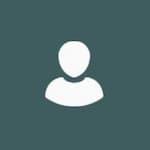
Lixia Xiao
Research Fellow
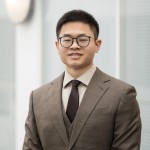
Dr Kai Yang
Lecturer in Energy Materials & Nanotechnology
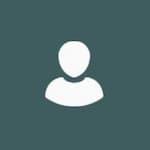
Dr Na Yi
Senior Research Fellow
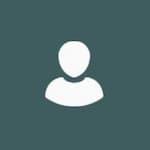
Ahmed Zoha
Research Fellow