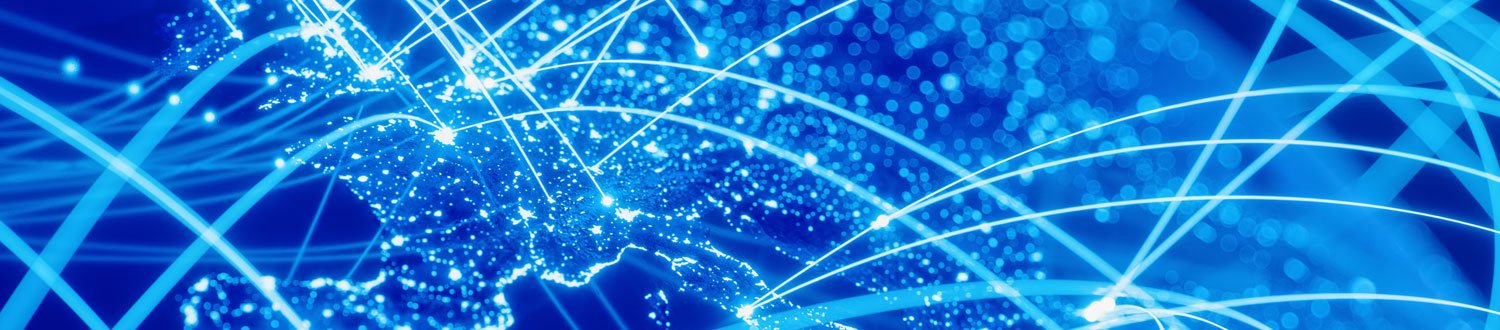
Academic staff
Get in contact with our academic staff.
Academic staff
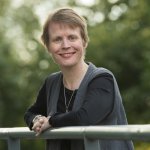
Professor Helen Treharne
Head of School of Computer Science and Electronic Engineering; Professor in Computer Science SFHEA, FBCS
Computer science staff
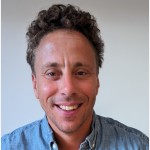
Dr Joseph Appleton
Lecturer in Computer Science
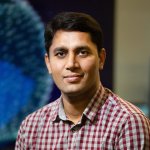
Dr Rizwan Asghar
Reader
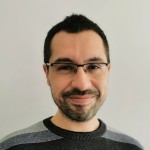
Dr Martin Barrere Cambrun
Lecturer in Digital Resilience
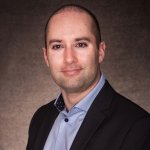
Dr Roman Bauer
Senior Lecturer
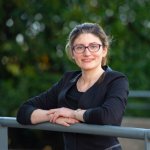
Professor Ioana Boureanu
Professor in Secure Systems & Head of Surrey Centre for Cyber Security (SCCS)
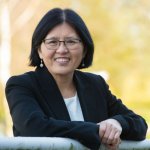
Professor Liqun Chen
Professor in Secure Systems
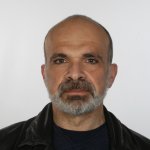
Professor Gregory Chockler
Professor in Computer Science
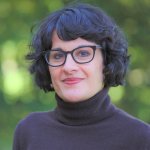
Dr Mariam Cirovic
Lecturer, Senior Programme Lead
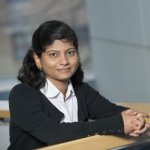
Dr Suparna De
Senior Lecturer in Computer Science
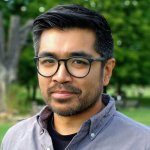
Professor Brijesh Dongol
Director of the UK Research Institute on Verified Trustworthy Software Systems (VeTSS)
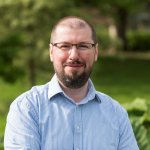
Dr Catalin Dragan
Senior Lecturer in Secure Systems
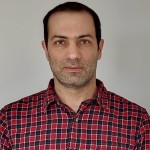
Dr Amir Esfahani
Associate Professor in Robotics
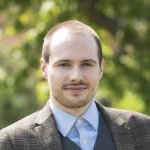
Nick Frymann
Lecturer in Computer Science
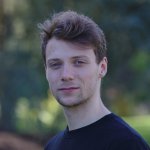
Dr Daniel Gardham
Lecturer
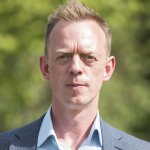
Dr Robert Granger
Reader in Secure Systems
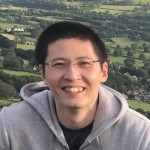
Dr Xiaowei Gu
Senior Lecturer
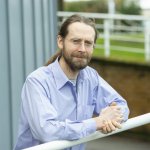
Dr Frank Guerin
Senior Lecturer
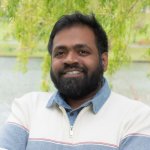
Dr Diptesh Kanojia
Senior Lecturer in People-Centred AI
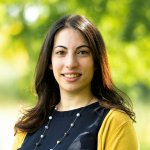
Stella Kazamia
Senior Lecturer | Director of Employability
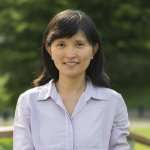
Dr Joey Lam
Lecturer
Dr Chaoyun Li
Lecturer in Software Security
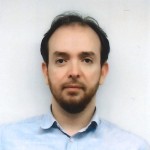
Dr Alaa Marshan
Senior Lecturer in Intelligent Data Analysis
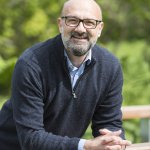
Dr Sotiris Moschoyiannis
Reader in Complex Systems
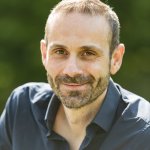
Professor Ferrante Neri
Professor of Machine Learning and Artificial Intelligence, Associate Dean (international) FEPS
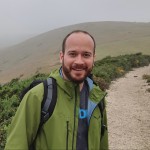
Dr Pedro Porto Buarque De Gusmao
Lecturer in Computer Science
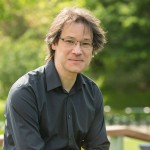
Dr Sasa Radomirovic
Senior Lecturer in Secure Systems; Programme Lead of MSc Information Security
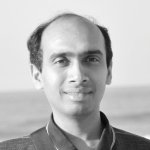
Professor Nishanth Sastry
Professor, Associate Head of Research and Innovation
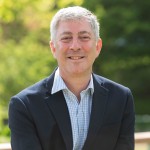
Professor Steve Schneider
Director of Computer Science Research Centre
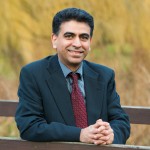
Dr Alireza Tamaddoni Nezhad
Reader (Associate Professor) in Machine Learning and Computational Intelligence
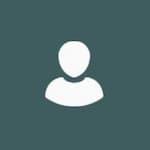
Professor H Lilian Tang
Professor in Artificial Intelligence
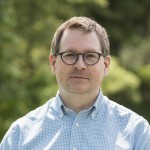
Dr Tom Thorne
Senior Lecturer; Programme Lead of MSc Data Science
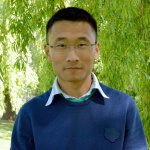
Dr Jack Tian
Lecturer in Cyber Security
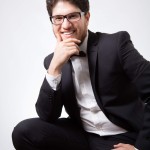
Dr Ehsan Toreini
Lecturer in Software Security
Electronic engineering staff
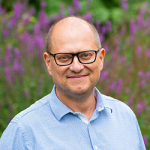
Professor Miroslaw Bober
Professor of Video Processing
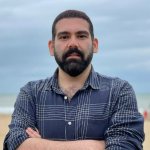
Dr Mahdi Boloursaz Mashhadi
Lecturer in Communications and AI
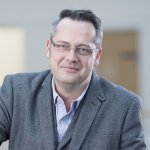
Professor Richard Bowden
Professor of Computer Vision and Machine Learning
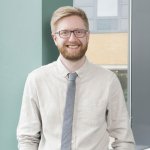
Dr Christopher Bridges
Reader in On-Board Data Handling (OBDH)
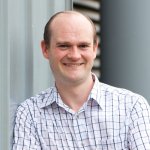
Dr Tim Brown
Senior Lecturer in RF Antennas and Propagation
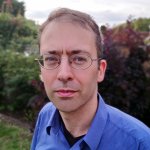
Dr Koen Buisman
Reader in microwave and mm-wave electronics
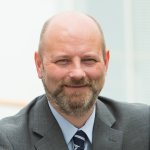
Professor David Carey
Associate Head, School of Computer Science and Electronic Engineering
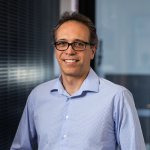
Professor Gustavo Carneiro
Professor of AI and Machine Learning
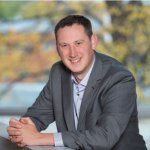
Professor John Collomosse
Professor of Computer Vision and AI | Director DECaDE: UKRI/EPSRC Centre for the Decentralised Digital Economy
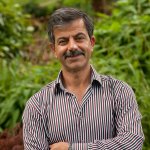
Dr Haitham Cruickshank
Reader
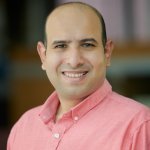
Dr Ahmed Elzanaty
Lecturer in Communication systems
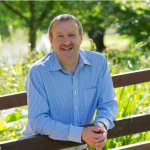
Professor Jonathan England
Professor of Ion Implantation Technology
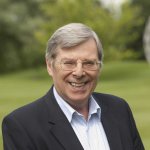
Professor Barry Evans
Professor-Information Systems Engineering
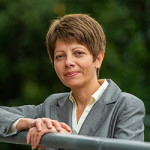
Dr Lucia Florescu
Lecturer in Medical Imaging
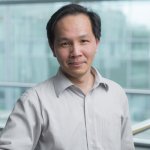
Dr Chuan Foh
Senior Lecturer
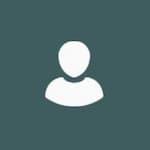
Professor Yue Gao
Visting Professor of Wireless Communications
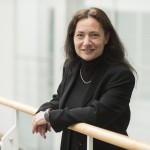
Dr Raffaella Guida
Reader in Satellite Remote Sensing
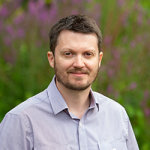
Dr Jean-Yves Guillemaut
Senior Lecturer in 3D Computer Vision
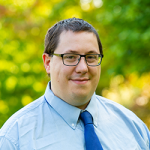
Dr Simon Hadfield
Associate Professor (Reader) in Robot Vision and Autonomous Systems
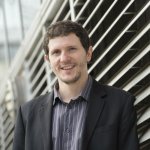
Dr Fabien Heliot
Lecturer in Wireless Communications
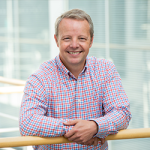
Professor Adrian Hilton
Director Surrey Institute for People-Centred AI | Director of Centre for Vision, Speech and Signal Processing
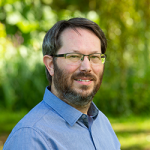
Professor Philip Jackson
Professor in Machine Audition
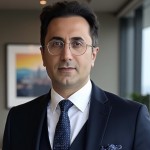
Dr Mohsen Khalily
Associate Professor in Antenna and Propagation
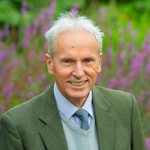
Professor Josef Kittler
Distinguished Professor
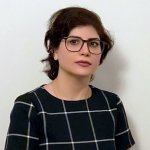
Dr Samaneh Kouchaki
Senior Lecturer in Machine Learning for Healthcare
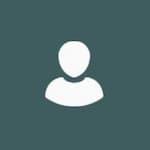
Dr Andrea Lucca Fabris
Senior Lecturer in Electric Propulsion
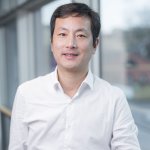
Professor Yi Ma
Professor of Cognitive Communications
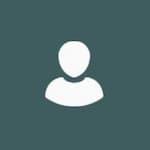
Professor Jiri Matas
Vice Chancellor Fellow
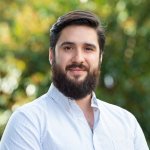
Dr Oscar Mendez Maldonado
Lecturer in Robotics and Artificial Intelligence
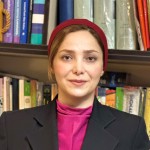
Dr Mahtab Mirmohseni
Senior Lecturer
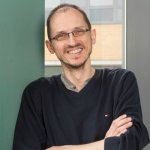
Professor Klaus Moessner
Professor in Cognitive Networks
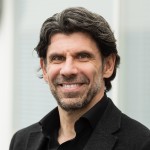
Dr Konstantinos Nikitopoulos
Professor in Wireless Communications & Signal Processing
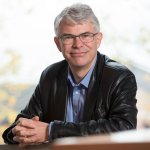
Professor Mark Plumbley
Professor of Signal Processing, EPSRC Fellow in "AI for Sound"
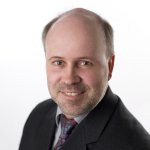
Professor Markus Pollnau
Professor in Photonics
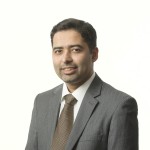
Dr Atta Quddus
Lecturer in Wireless Communications
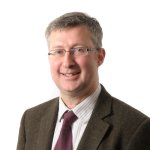
Professor Keith Ryden
Head of Surrey Space Centre, Professor in Space Engineering
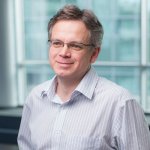
Dr Maxim Shkunov
Senior Lecturer
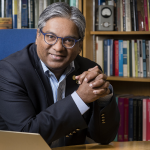
Professor Ravi Silva
Distinguished Professor, Interim Director - Institute for Sustainability (IfS), Director - Advanced Technology Institute (ATI) and Head of NanoElectronics Centre
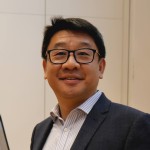
Professor Yi-Zhe Song
Co-Director Surrey Institute for People-Centred AI; Programme Lead of MSc in Artificial Intelligence
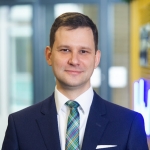
Dr Radu Sporea
Associate Professor in Semiconductor Devices
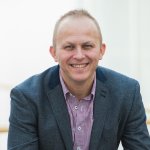
Dr Vlad Stolojan
Associate Professor (Reader)
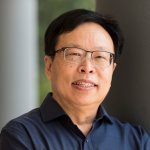
Professor Zhili Sun
Professor of Communication Networking
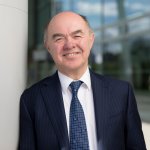
Martin Sweeting
Distinguished Professor of Space Engineering
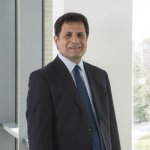
Professor Rahim Tafazolli
Head of the Institute for Communication Systems (ICS)
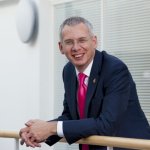
Professor Craig Underwood
Emeritus Professor of Spacecraft Engineering
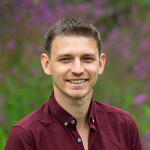
Dr Marco Volino
Lecturer in Computer Vision and Graphics
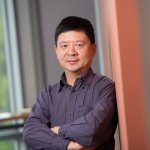
Professor Ning Wang
Professor in Networks
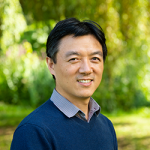
Professor Wenwu Wang
Professor in Signal Processing and Machine Learning; Associate Head (External Engagement), School of Computer Science and Electronic Engineering
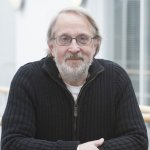
Professor Roger Webb
Director of Ion Beam Centre, Professor of Ion Beam Physics
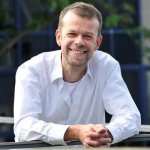
Professor Kevin Wells
Professor of AI in Human and Veterinary Healthcare
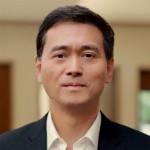
Professor Pei Xiao
Professor of Wireless Communications
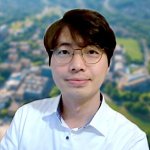
Dr Jae Yun
Lecturer in Energy Technology
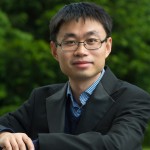
Professor Wei Zhang
Professor of Energy Technology
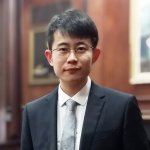
Dr Yunlong Zhao
Visiting Academic